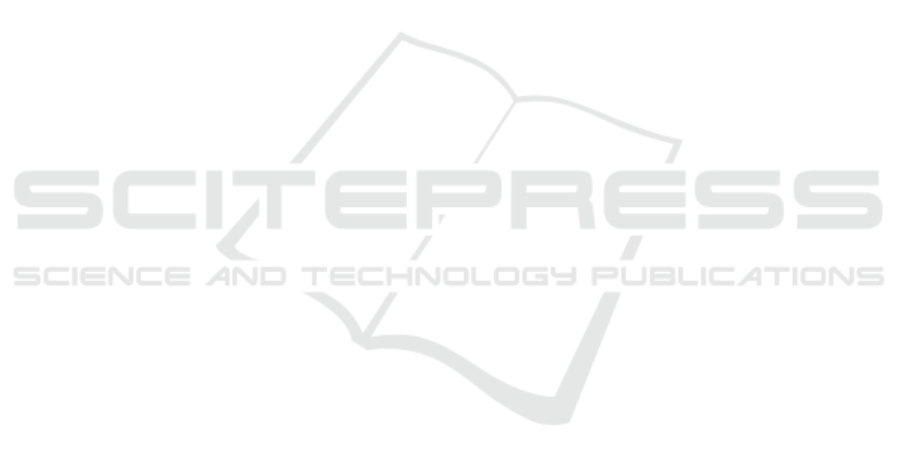
Olivares, L. (2024). Innovative extenso-inclinometer
for slow-moving deep-seated landslide monitoring in
an early warning perspective. JOURNAL OF ROCK
MECHANICS AND GEOTECHNICAL ENGINEER-
ING.
de Cristofaro, M., Asadi, M. S., Chiaradonna, A., Dami-
ano, E., Netti, N., Olivares, L., and Orense, R. (2024).
Modeling the excess porewater pressure buildup in py-
roclastic soils subjected to cyclic loading. JOURNAL
OF GEOTECHNICAL AND GEOENVIRONMENTAL
ENGINEERING, 150.
Di Bonito, L. P., Campanile, L., Di Natale, F., Mastroianni,
M., and Iacono, M. (2024). explainable artificial intel-
ligence in process engineering: Promises, facts, and
current limitations. Applied System Innovation, 7(6).
Di Bonito, L. P., Campanile, L., Napolitano, E., Iacono,
M., Portolano, A., and Di Natale, F. (2023). Analy-
sis of a marine scrubber operation with a combined
analytical/ai-based method. Chemical Engineering
Research and Design, 195:613 – 623.
Dietterich, T. G. (2000). Ensemble methods in machine
learning. Lecture Notes in Computer Science (includ-
ing subseries Lecture Notes in Artificial Intelligence
and Lecture Notes in Bioinformatics), 1857 LNCS:1 –
15.
Domingos, P. (2012). A few useful things to know about
machine learning. Commun. ACM, 55(10):78–87.
eli5 Development Team (Accessed 2022). eli5 Documenta-
tion - Permutation Importance.
Gunning, D. and Aha, D. W. (2019). Darpa’s explainable ar-
tificial intelligence program. AI Magazine, 40(2):44–
58.
Guyon, I. and Elisseeff, A. (2003). An introduction to
variable and feature selection. J. Mach. Learn. Res.,
3(null):1157–1182.
Karpatne, A., Atluri, G., Faghmous, J. H., Steinbach, M.,
Banerjee, A., Ganguly, A., Shekhar, S., Samatova, N.,
and Kumar, V. (2017). Theory-guided data science:
A new paradigm for scientific discovery from data.
IEEE Transactions on Knowledge and Data Engineer-
ing, 29.
Ke, G., Meng, Q., Finley, T., Wang, T., Chen, W., Ma, W.,
Ye, Q., and Liu, T.-Y. (2017). Lightgbm: A highly ef-
ficient gradient boosting decision tree. In Advances
in Neural Information Processing Systems, volume
2017-December, page 3147 – 3155.
Laub, J. A. (1999). Assessing the servant organization; de-
velopment of the organizational leadership assessment
(ola) model. dissertation abstracts international,. Pro-
cedia - Social and Behavioral Sciences, 1.
Lundberg, S. M. and Lee, S.-I. (2017). A unified approach
to interpreting model predictions. In Advances in Neu-
ral Information Processing Systems, volume 2017-
December, page 4766 – 4775.
Malami, S. I., Anwar, F. H., Abdulrahman, S., Haruna, S. I.,
Ali, S. I. A., and Abba, S. I. (2021). Implementation of
hybrid neuro-fuzzy and self-turning predictive model
for the prediction of concrete carbonation depth: A
soft computing technique. Results in Engineering, 10.
Mangalathu, S., Hwang, S.-H., and Jeon, J.-S. (2020). Ma-
chine learning-based failure mode identification of re-
inforced concrete shear walls. Engineering Structures,
207:110257.
McGovern, A., Lagerquist, R., Gagne, D. J., Jergensen,
G. E., Elmore, K. L., Homeyer, C. R., and Smith,
T. (2019). Making the black box more transparent:
Understanding the physical implications of machine
learning. Bulletin of the American Meteorological So-
ciety, 100.
Momeny, M., Latif, A. M., Sarram, M. A., Sheikhpour, R.,
and Zhang, Y. D. (2021). A noise robust convolutional
neural network for image classification. Results in En-
gineering, 10.
Pearson, K. (1895). Note on regression and inheritance in
the case of two parents. Proc. R. Soc. Lond., 58:240–
242.
Prokhorenkova, L., Gusev, G., Vorobev, A., Dorogush,
A. V., and Gulin, A. (2018). Catboost: Unbi-
ased boosting with categorical features. In Advances
in Neural Information Processing Systems, volume
2018-December, page 6638 – 6648.
Santhosh, A. J., Tura, A. D., Jiregna, I. T., Gemechu, W. F.,
Ashok, N., and Ponnusamy, M. (2021). Optimization
of cnc turning parameters using face centred ccd ap-
proach in rsm and ann-genetic algorithm for aisi 4340
alloy steel. Results in Engineering, 11.
Sharma, D. K., Jalil, N. A., Nassa, V. K., Vadyala, S. R.,
Senthamil, L. S., and Thangadurai, N. (2021). Deep
learning applications to classify cross-topic natural
language texts based on their argumentative form. In
Proceedings - 2nd International Conference on Smart
Electronics and Communication, ICOSEC 2021.
Vadyala, S. R., Betgeri, S. N., and Betgeri, N. P. (2022).
Physics-informed neural network method for solving
one-dimensional advection equation using pytorch.
Array, 13.
Vadyala, S. R., Betgeri, S. N., Sherer, E. A., and Amrit-
phale, A. (2021). Prediction of the number of covid-
19 confirmed cases based on k-means-lstm. Array, 11.
Vadyala, S. R. and Sherer, E. A. (2021). Natural
language processing accurately categorizes indica-
tions, findings and pathology reports from multicenter
colonoscopy: Qualitative focus study (preprint). JMIR
Cancer.
Velliangiri, S., Alagumuthukrishnan, S., and Thankumar
joseph, S. I. (2019). A review of dimensionality re-
duction techniques for efficient computation. Proce-
dia Computer Science, 165:104–111.
Zona, R., Ferla, P., and Minutolo, V. (2021). Limit anal-
ysis of conical and parabolic domes based on semi-
analytical solution. Journal of Building Engineering,
44.
Zona, R. and Minutolo, V. (2024). A dislocation-based fi-
nite element method for plastic collapse assessment in
solid mechanics. Archive of Applied Mechanics.
AI4EIoT 2025 - Special Session on Artificial Intelligence for Emerging IoT Systems: Open Challenges and Novel Perspectives
480