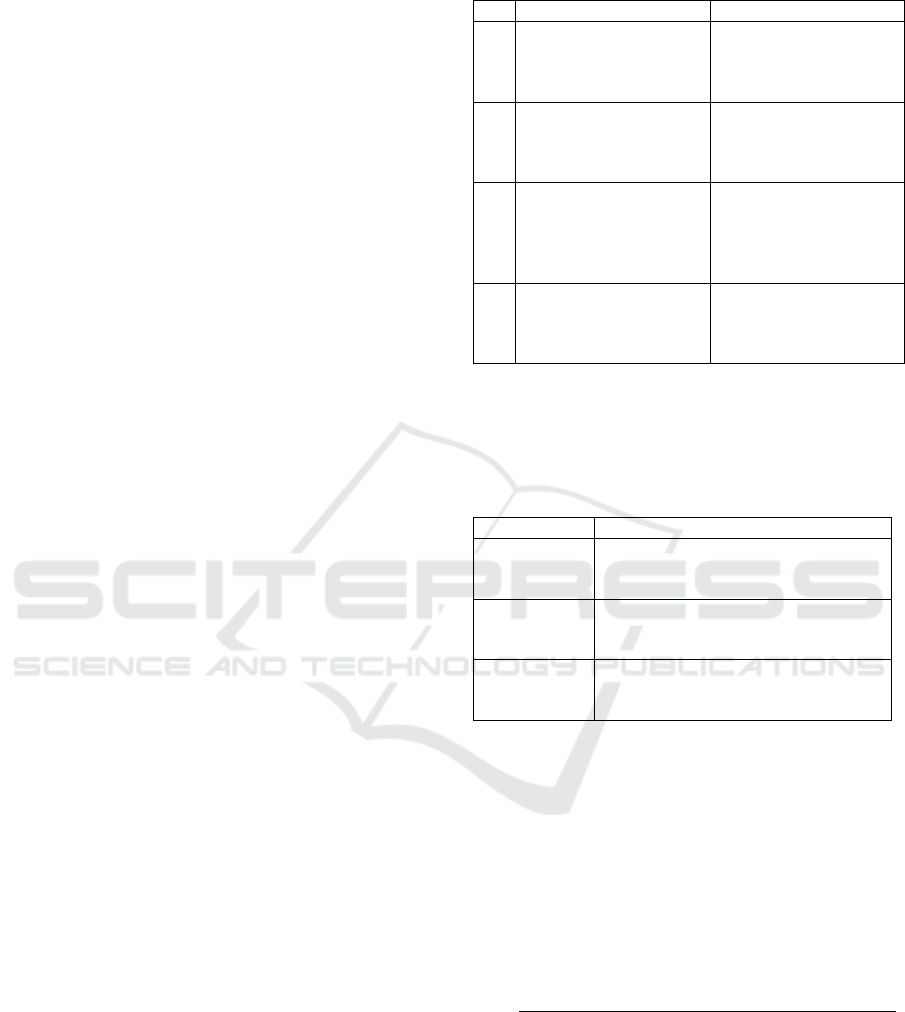
view], which naturally groups students into prede-
fined teams.
The primary goal of this study is to investigate the
impact of individual and group modeling approaches
on the quality of BPMN models and students’ emo-
tions during the learning process. To achieve this ob-
jective, we define three research questions:
RQ1: What emotions do students report when per-
forming individual and group BPMN modeling?
RQ2: What are the differences in the technical qual-
ity of BPMN models (correctness and completeness)
between individual and group modeling?
RQ3: Does personality profile, as defined by Keirsey’s
typology, influence the technical quality of models or
students’ emotions during modeling activities?
Aims to answer these questions, this quasi-
experiment occurred in three main phases:
1. Training and Familiarization. Students partici-
pated in three classes about BPMN, covering the-
oretical foundations and practical modeling tech-
niques using BPMN tools. A categorization ques-
tionnaire was administered to measure students’
prior experience with process modeling, ensuring
a baseline for future comparisons.
2. Experimental Sessions. This phase occurred in
three stages:
Stage 1. All 36 participants individually modeled
a process in BPMN.
Stage 2. The six groups were randomly assigned
by drawing lots. Groups 2, 3, and 6 performed
the modeling activity in groups, while Groups 1,
4, and 5 completed the exercise individually.
Stage 3. Students modeled another business pro-
cess. Roles were reversed for the subsequent
task, enabling cross-comparisons between indi-
vidual and collaborative conditions.
3. Feedback and Data Collection. After each
session, students completed self-report question-
naires to assess their emotional experiences (mo-
tivation, enjoyment, and relaxation) using a Lik-
ert scale represented by emojis. BPMN models
were evaluated for correctness (accuracy in us-
ing BPMN elements and relationships) and com-
pleteness (including all relevant information for
the modeled scenario) by assistant teachers using
predefined criteria.
3.3 Hypotheses, Variables and
Measurements
The formulation of research hypotheses, outlined in
Table 1, was guided by the study’s central questions
and objectives research.
Table 1: Research Hypotheses.
ID H
0
(Null Hypothesis) H
1
(Alternative Hypothesis)
H1 There is no significant differ-
ence in correctness of BPMN
models produced individually
and in groups.
There is significant difference
in correctness of BPMN mod-
els between individual and
group approaches.
H2 There is no significant dif-
ference in completeness of
BPMN models produced indi-
vidually and in groups.
There is significant difference
in completeness of BPMN
models between individual
and group approaches.
H3 There is no significant dif-
ference in students’ emotions
(motivation, enjoyment, and
relaxation) between individual
and group modeling.
There is significant differ-
ence in students’ emotions be-
tween individual and group
approaches.
H4 Keirsey profiles do not signif-
icantly influence correctness
and completeness of BPMN
models.
Keirsey profiles significantly
influence correctness and
completeness of BPMN
models.
The variables were categorized to provide a struc-
tured understanding of the interactions between the
treatments applied and the observed outcomes, as out-
lined in Table 2.
Table 2: Variables and Attributes.
Category Description
Independent
Variables (Pa-
rameters)
Tool used (Bizagi Modeler), model structures
(AS-IS and TO-BE), evaluation method.
Independent
Variables (Fac-
tors)
Modeling approach (individual or group),
Keirsey profile (Guardian, Artisan, Idealist,
Rational).
Dependent Vari-
ables
Quality of BPMN models (Correctness and Com-
pleteness), Students’ emotions (Motivation, En-
joyment, and Relaxation).
Correctness (CR) and completeness (CM) were
chosen as critical indicators of model quality, sup-
ported by prior BPMN evaluation studies (Paschoal
and et al., 2020). CR was calculated using a weighted
formula for error severity (light, medium, severe), of-
fering a nuanced assessment of technical accuracy.
This weighted system provides a more detailed
evaluation than binary correctness measures, captur-
ing the practical significance of different error types
for the model’s usability. The formula for CR is:
CR =
EC
EC + (1 × EI
Light
) + (2 × EI
Medium
) + (3 × EI
Severe
)
The maximum correctness value (CR = 1) is
achieved when all model elements are correct, with
no errors. Conversely, CR = 0 reflects all elements
being incorrect and classified as severe errors.
Completeness (CM) was calculated similarly, in-
corporating the presence of relevant information and
the severity of omissions. This metric evaluates
the quantity of information included and the impact
CSEDU 2025 - 17th International Conference on Computer Supported Education
622