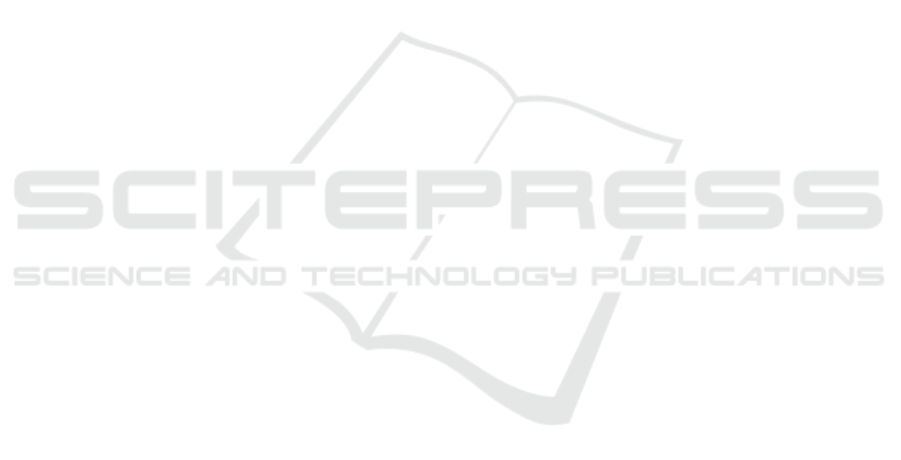
ACKNOWLEDGEMENTS
The publication of this article was partially supported
by the 2024 Development Fund of the Babes-Bolyai
University.
REFERENCES
Author(s), A. (accessed January 2025). Healthcare
bias in ai: A systematic literature review. =
https://figshare.com/s/cc41c628a51a442181fd.
Belenguer, L. (2022). Ai bias: exploring discriminatory
algorithmic decision-making models and the appli-
cation of possible machine-centric solutions adapted
from the pharmaceutical industry. AI and Ethics,
2(4):771–787.
Bouderhem, R. (2024). Shaping the future of ai in health-
care through ethics and governance. Humanities and
Social Sciences Communications, 11(1):1–12.
Burema, D., Debowski-Weimann, N., von Janowski,
A., Grabowski, J., Maftei, M., Jacobs, M., Van
Der Smagt, P., and Benbouzid, D. (2023). A sector-
based approach to ai ethics: Understanding ethical is-
sues of ai-related incidents within their sectoral con-
text. In Proceedings of the 2023 AAAI/ACM Confer-
ence on AI, Ethics, and Society, pages 705–714.
Clark, P., Kim, J., and Aphinyanaphongs, Y. (2023). Mar-
keting and us food and drug administration clear-
ance of artificial intelligence and machine learning en-
abled software in and as medical devices: a system-
atic review. JAMA Network Open, 6(7):e2321792–
e2321792.
Fraser, H., Coiera, E., and Wong, D. (2018). Safety of
patient-facing digital symptom checkers. The Lancet,
392(10161):2263–2264.
Guidance, W. (2021). Ethics and governance of artificial
intelligence for health. World Health Organization.
Haddaway, N. R., Page, M. J., Pritchard, C. C., and
McGuinness, L. A. (2022). Prisma2020: An r package
and shiny app for producing prisma 2020-compliant
flow diagrams, with interactivity for optimised digital
transparency and open synthesis. Campbell systematic
reviews, 18(2):e1230.
Jain, A., Brooks, J. R., Alford, C. C., Chang, C. S., Mueller,
N. M., Umscheid, C. A., and Bierman, A. S. (2023).
Awareness of racial and ethnic bias and potential so-
lutions to address bias with use of health care algo-
rithms. In JAMA Health Forum, volume 4, pages
e231197–e231197. American Medical Association.
Kamulegeya, L., Bwanika, J., Okello, M., Rusoke, D., Nas-
siwa, F., Lubega, W., Musinguzi, D., and B
¨
orve, A.
(2023). Using artificial intelligence on dermatology
conditions in uganda: A case for diversity in training
data sets for machine learning. African Health Sci-
ences, 23(2):753–63.
Kitchenham, B. and Charters, S. (2007). Guidelines for per-
forming systematic literature reviews in software en-
gineering. 2.
Kumar, A., Aelgani, V., Vohra, R., Gupta, S. K., Bhagawati,
M., Paul, S., Saba, L., Suri, N., Khanna, N. N., Laird,
J. R., et al. (2024). Artificial intelligence bias in med-
ical system designs: A systematic review. Multimedia
Tools and Applications, 83(6):18005–18057.
Lecher, C. (2020). Can a robot decide my medical treat-
ment?
Ledford, H. (2019). Millions affected by racial bias in
health-care algorithm. Nature, 574(31):2.
Meline, T. (2006). Selecting studies for systemic re-
view: Inclusion and exclusion criteria. Contempo-
rary issues in communication science and disorders,
33(Spring):21–27.
Mienye, I. D., Obaido, G., Emmanuel, I. D., and Ajani,
A. A. (2024). A survey of bias and fairness in health-
care ai. In 2024 IEEE 12th International Confer-
ence on Healthcare Informatics (ICHI), pages 642–
650. IEEE.
Page, M. J., McKenzie, J. E., Bossuyt, P. M., Boutron, I.,
Hoffmann, T. C., Mulrow, C. D., Shamseer, L., Tet-
zlaff, J. M., Akl, E. A., Brennan, S. E., et al. (2021).
The prisma 2020 statement: an updated guideline for
reporting systematic reviews. bmj, 372.
Panch, T., Mattie, H., and Atun, R. (2019). Artificial intel-
ligence and algorithmic bias: implications for health
systems. Journal of global health, 9(2).
Simonite, T. (2020). How an algorithm blocked kidney
transplants to black patients— wired.
Singh, B., Rahim, M. A. B. U., Hussain, S., Rizwan, M. A.,
and Zhao, J. (2023). Ai ethics in healthcare-a sur-
vey. In 2023 IEEE 23rd International Conference on
Software Quality, Reliability, and Security Compan-
ion (QRS-C), pages 826–833. IEEE.
Sjoding, M. W., Dickson, R. P., Iwashyna, T. J., Gay, S. E.,
and Valley, T. S. (2020). Racial bias in pulse oxime-
try measurement. New England Journal of Medicine,
383(25):2477–2478.
Thompson, A. (2020). Coronavirus: Models pre-
dicting patient outcomes may be “flawed” and
“based on weak evidence. Yahoo! Retrieved from
https://sg.style.yahoo.com/style/coronavirus-covid19-
models-patientoutcomes-flawed-153227342.html.
van Assen, M., Beecy, A., Gershon, G., Newsome, J.,
Trivedi, H., and Gichoya, J. (2024). Implications
of bias in artificial intelligence: considerations for
cardiovascular imaging. Current Atherosclerosis Re-
ports, 26(4):91–102.
Wiggers, K. (2020a). Covid-19 vaccine distribution algo-
rithms may cement health care inequalities.
Wiggers, K. (2020b). Google’s breast cancer-predicting ai
research is useless without transparency, critics say.
Williamson, S. M. and Prybutok, V. (2024). Balanc-
ing privacy and progress: a review of privacy chal-
lenges, systemic oversight, and patient perceptions in
ai-driven healthcare. Applied Sciences, 14(2):675.
Yfantidou, S., Sermpezis, P., Vakali, A., and Baeza-Yates,
R. (2023). Uncovering bias in personal informatics.
Proceedings of the ACM on Interactive, Mobile, Wear-
able and Ubiquitous Technologies, 7(3):1–30.
ENASE 2025 - 20th International Conference on Evaluation of Novel Approaches to Software Engineering
842