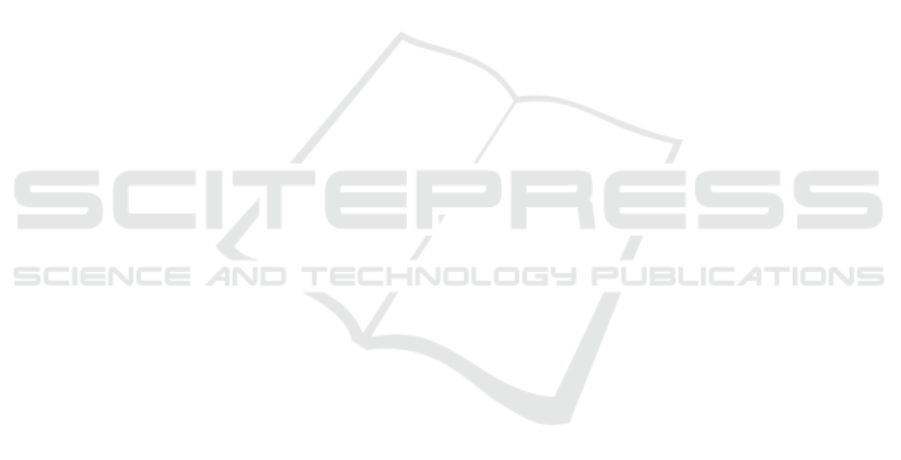
REFERENCES
Cassel, A., Bergenhem, C., Christensen, O. M., Heyn, H.-
M., Leadersson-Olsson, S., Majdandzic, M., Sun, P.,
Thors
´
en, A., and Trygvesson, J. (2020). On percep-
tion safety requirements and multi sensor systems for
automated driving systems. SAE International Jour-
nal of Advances and Current Practices in Mobility,
2(2020-01-0101):3035–3043.
Clavijo, M., Jim
´
enez, F., Serradilla, F., and D
´
ıaz-
´
Alvarez,
A. (2022). Assessment of cnn-based models for
odometry estimation methods with lidar. Mathemat-
ics, 10(18):3234.
Fazekas, M., G
´
asp
´
ar, P., and N
´
emeth, B. (2020). Identifica-
tion of kinematic vehicle model parameters for local-
ization purposes. In 2020 IEEE International Confer-
ence on Multisensor Fusion and Integration for Intel-
ligent Systems (MFI), pages 373–380. IEEE.
Goelles, T., Schlager, B., and Muckenhuber, S. (2020).
Fault detection, isolation, identification and recovery
(fdiir) methods for automotive perception sensors in-
cluding a detailed literature survey for lidar. Sensors,
20(13):3662.
Hsu, L.-Y. and Chen, T.-L. (2012). Vehicle dynamic predic-
tion systems with on-line identification of vehicle pa-
rameters and road conditions. Sensors, 12(11):15778–
15800.
Jin, X., Yin, G., and Chen, N. (2019). Advanced estima-
tion techniques for vehicle system dynamic state: A
survey. Sensors, 19(19):4289.
Katoch, S., Chauhan, S. S., and Kumar, V. (2021). A re-
view on genetic algorithm: past, present, and future.
Multimedia tools and applications, 80:8091–8126.
Khan, N. H. and Adnan, A. (2017). Ego-motion estimation
concepts, algorithms and challenges: an overview.
Multimedia Tools and Applications, 76:16581–16603.
Kwon, W., Lee, H., Kim, A., and Yi, K. (2025). Lidar-based
odometry estimation using wheel speed and vehicle
model for autonomous buses. International Journal
of Control, Automation, and Systems, 23(1):41–54.
Matos, F., Bernardino, J., Dur
˜
aes, J., and Cunha, J. (2024).
A survey on sensor failures in autonomous vehicles:
Challenges and solutions. Sensors, 24(16):5108.
Par, K., Peker, A. U., Ghahremaninejad, R.,
¨
Ozcan, A., and
Sahin, E. (2024). Introducing flowride® logger, an on-
board data collection framework for commercial auto-
mated vehicles. In VEHITS, pages 488–495.
Pillai, S. and Leonard, J. J. (2017). Towards visual ego-
motion learning in robots. In 2017 IEEE/RSJ Interna-
tional Conference on Intelligent Robots and Systems
(IROS), pages 5533–5540. IEEE.
Saadeddin, K., Abdel-Hafez, M. F., and Jarrah, M. A.
(2014). Estimating vehicle state by gps/imu fusion
with vehicle dynamics. Journal of Intelligent &
Robotic Systems, 74:147–172.
Safavi, S., Safavi, M. A., Hamid, H., and Fallah, S. (2021).
Multi-sensor fault detection, identification, isolation
and health forecasting for autonomous vehicles. Sen-
sors, 21(7):2547.
Smith, S. W. (1997). The scientist and engineer’s guide to
digital signal processing. California Technical Pub.
Wang, Y., Mangnus, J., Kosti
´
c, D., Nijmeijer, H., and
Jansen, S. T. (2011). Vehicle state estimation using
GPS/IMU integration. IEEE.
Wu, M., Lam, S.-K., and Srikanthan, T. (2017). A frame-
work for fast and robust visual odometry. IEEE
Transactions on Intelligent Transportation Systems,
18(12):3433–3448.
Vehicle Longitudinal Speed Estimation Using 3DOF Localization Information and Genetic Solver
227