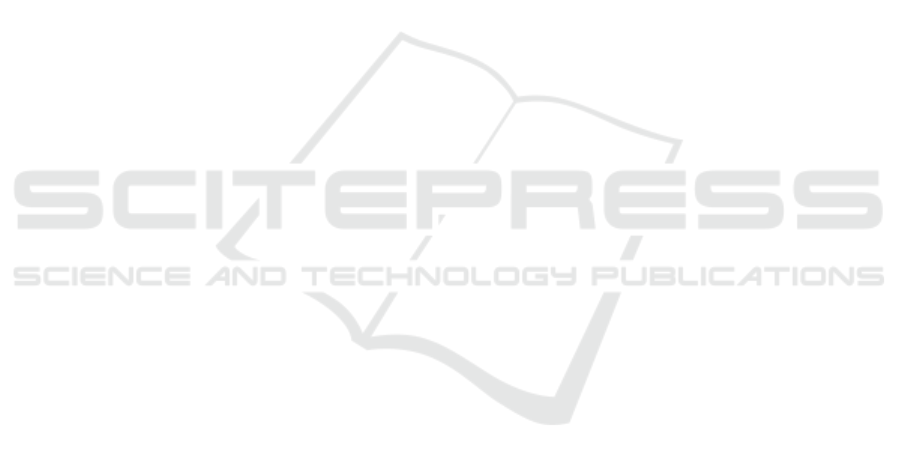
and investigated the potential subjectivity of the au-
thor in data processing. By employing text analysis
in accordance with the recommended data processing
standards, we aimed to reduce this risk. We showed
participants that we were committed to ethics by in-
forming them of our goal, by using anonymous data
gathering methods, and letting them know how we in-
tended to use the data.
7 CONCLUSIONS AND FUTURE
WORK
The study is founded on four central research ques-
tions which we have methodologically approached
to investigate students’ perception of Big Data in
higher education. The results confirm the main chal-
lenges related to Big Data in education, as identified
in the related work of the paper. As the first impli-
cation of the study’s results, students need method-
ological guidance from university educators in order
to be able to learn and adapt to the emergent nature
of the Big Data domain. Most importantly, in the
field of Big Data, students need curricula that align
with their learning objectives and expectations and
with their future professional careers. The curricula
should build competencies around fundamental con-
cepts and techniques, and most importantly around
hands-on activities. Students learn best in relation
with each other; therefore, we recommend that learn-
ing occur in groups or teams of students with diverse
backgrounds and experiences, in settings as close to
the real world as possible. Another important factor
to the success of students’ learning path is the con-
tinuous academia-industry collaboration. Integrating
guest lectures from industry professionals and prac-
tical workshops led by experts working daily with
emerging Big Data technologies, where students can
validate their competencies, is crucial for learning in
this domain.
A longitudinal study to reveal further insights into
the course’s lasting impact is in progress. Moreover,
it would be interesting to analyze how the students’
perceptions and interests evolve after the course. This
would imply another survey, possibly combined with
student evaluation results. The future approach could
also be used to assess learning outcomes and their cor-
relation with students’ professional trajectories upon
graduation. This would bring an important contribu-
tion towards the continuous improvement of the exist-
ing curriculum and the teaching approach, in order to
better prepare students for the challenges and oppor-
tunities of Big Data Engineering.
REFERENCES
Baig, M. I., Shuib, L., and Yadegaridehkordi, E. (2020). Big
data in education: a state of the art, limitations, and
future research directions. Int J Educ Technol High
Educ, 17(44).
Dzuranin, A. C., Jones, J. R., and Olvera, R. M. (2018). In-
fusing data analytics into the accounting curriculum:
A framework and insights from faculty. Journal of
Accounting Education, 43:24–39.
Kim, K., Kwon, K., and Ottenbreit-Leftwich, A. (2023).
Exploring middle school students’ common naive
conceptions of artificial intelligence concepts, and
the evolution of these ideas. Educ Inf Technol,
28:9827–9854.
Komljenovic, J., Sellar, S., and Birch, K. (2024). Turning
universities into data-driven organisations: seven di-
mensions of change. High. Educ.
Kumar, S., Rao, P., Singhania, S., Verma, S., and Kheterpal,
M. (2024). Will artificial intelligence drive the ad-
vancements in higher education? a tri-phased explo-
ration. Technological Forecasting and Social Change,
201.
Lundie, D. (2024). The ethics of research and teaching in
an age of big data. Journal of Comparative & Inter-
national Higher Education, 16.
Mamadou, L. and Ernesto, E. (2020). University 4.0: The
industry 4.0 paradigm applied to education. J. of the
Acad. Mark. Sci., 43:115–135.
Nelson, M. S. and Pouchard, L. (2017). A pilot “big data”
education modular curriculum for engineering gradu-
ate education: Development and implementation. In
2017 IEEE Frontiers in Education Conference (FIE),
pages 1–5.
Ralph, P. (2021). Acm sigsoft empirical standards for
software engineering research, version 0.2. 0. URL:
https://github. com/acmsigsoft/EmpiricalStandards.
Sigman, B. P., Garr, W., Pongsajapan, R., Selvanadin, M.,
Bolling, K., and Marsh, G. (2014). Teaching big data:
Experiences, lessons learned, and future directions.
Educ Inf Technol.
Sledgianowski, D., Gomaa, M., and Tan, C. (2017). Toward
integration of big data, technology and information
systems competencies into the accounting curriculum.
Journal of Accounting Education, 38:81–93.
Song, I. Y. and Zhu, Y. (2016). Big data and data science:
what should we teach? Expert Systems, 29:364–373.
Valle, N. N., Kilat, R. V., Lim, J., General, E., Cruz, J. D.,
Colina, S. J., Batican, I., and Valle, L. (2024). Mod-
eling learners’ behavioral intention toward using ar-
tificial intelligence in education. Social Sciences &
Humanities Open, 10.
Xu, L., Zhang, J., Ding, Y., Sun, G., Zhang, W., Philbin, S.,
and Guo, B. (2022). Assessing the impact of digital
education and the role of the big data analytics course
to enhance the skills and employability of engineering
students. Front. Psychol, 13.
Students’ Perception of Big Data Engineering in Higher Education Curricula: Expectations, Interest and Ethical Implications
797