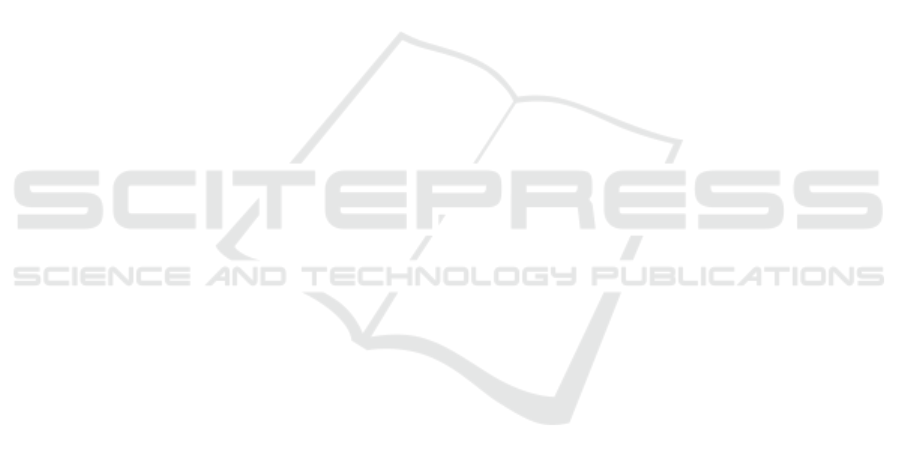
Bae, K. T., Kim, J.-S., Na, Y.-H., Kim, K. G., and Kim,
J.-H. (2005). Pulmonary nodules: automated detec-
tion on ct images with morphologic matching algo-
rithm—preliminary results. Radiology, 236(1):286–
293.
Ballabio, D., Todeschini, R., and Consonni, V. (2019). Re-
cent advances in high-level fusion methods to classify
multiple analytical chemical data. Data Handling in
Science and Technology, 31:129–155.
Chan, T. and Vese, L. (1999). An active contour model
without edges. In International Conference on Scale-
Space Theories in Computer Vision, pages 141–151.
Springer.
Chen, L.-C., Zhu, Y., Papandreou, G., Schroff, F., and
Adam, H. (2018). Encoder-decoder with atrous sepa-
rable convolution for semantic image segmentation. In
Proceedings of the European conference on computer
vision (ECCV), pages 801–818.
Codella, N. C., Gutman, D., Celebi, M. E., Helba, B.,
Marchetti, M. A., Dusza, S. W., Kalloo, A., Liopy-
ris, K., Mishra, N., Kittler, H., et al. (2018). Skin
lesion analysis toward melanoma detection: A chal-
lenge at the 2017 international symposium on biomed-
ical imaging (isbi), hosted by the international skin
imaging collaboration (isic). In 2018 IEEE 15th In-
ternational Symposium on Biomedical Imaging (ISBI
2018), pages 168–172. IEEE.
Daghrir, J., Tlig, L., Bouchouicha, M., and Sayadi, M.
(2020). Melanoma skin cancer detection using deep
learning and classical machine learning techniques: A
hybrid approach. In 2020 5th International Confer-
ence on Advanced Technologies for Signal and Image
Processing (ATSIP), pages 1–5. IEEE.
He, K., Zhang, X., Ren, S., and Sun, J. (2016a). Deep resid-
ual learning for image recognition. In Proceedings of
the IEEE conference on computer vision and pattern
recognition, pages 770–778.
He, K., Zhang, X., Ren, S., and Sun, J. (2016b). Iden-
tity mappings in deep residual networks. In Euro-
pean conference on computer vision, pages 630–645.
Springer.
Heinzerling, L. and Eigentler, T. K. (2021). Skin cancer
in childhood and adolescents: Treatment and implica-
tions for the long-term follow-up. In Late Treatment
Effects and Cancer Survivor Care in the Young, pages
349–355. Springer.
Jadhav, A. R., Ghontale, A. G., and Shrivastava, V. K.
(2019). Segmentation and border detection of
melanoma lesions using convolutional neural network
and svm. In Computational Intelligence: Theories,
Applications and Future Directions-Volume I, pages
97–108. Springer.
James, G., Witten, D., Hastie, T., and Tibshirani, R. (2013).
An introduction to statistical learning, volume 112.
Springer.
Jamil, U., Sajid, A., Hussain, M., Aldabbas, O., Alam, A.,
and Shafiq, M. U. (2019). Melanoma segmentation
using bio-medical image analysis for smarter mobile
healthcare. Journal of Ambient Intelligence and Hu-
manized Computing, 10(10):4099–4120.
Kang, B., Zhang, P., Gao, Z., Chhipi-Shrestha, G., Hewage,
K., and Sadiq, R. (2020). Environmental assessment
under uncertainty using dempster–shafer theory and
z-numbers. Journal of Ambient Intelligence and Hu-
manized Computing, 11(5):2041–2060.
Khazaei, Z., Ghorat, F., Jarrahi, A., Adineh, H., Sohrabi-
vafa, M., and Goodarzi, E. (2019). Global incidence
and mortality of skin cancer by histological subtype
and its relationship with the human development in-
dex (hdi); an ecology study in 2018. World Cancer
Res J, 6(2):e13.
Litjens, G., Kooi, T., Bejnordi, B. E., Setio, A. A. A.,
Ciompi, F., Ghafoorian, M., Van Der Laak, J. A.,
Van Ginneken, B., and S
´
anchez, C. I. (2017). A survey
on deep learning in medical image analysis. Medical
image analysis, 42:60–88.
Maglogiannis, I. and Doukas, C. N. (2009). Overview of ad-
vanced computer vision systems for skin lesions char-
acterization. IEEE transactions on information tech-
nology in biomedicine, 13(5):721–733.
Marquez-Neila, P., Baumela, L., and Alvarez, L. (2013). A
morphological approach to curvature-based evolution
of curves and surfaces. IEEE Transactions on Pattern
Analysis and Machine Intelligence, 36(1):2–17.
Mbarki, W., Bouchouicha, M., Frizzi, S., Tshibasu, F.,
Farhat, L. B., and Sayadi, M. (2020). Semi-automatic
segmentation of intervertebral disc for diagnosing her-
niation using axial view mri. In 2020 5th International
Conference on Advanced Technologies for Signal and
Image Processing (ATSIP), pages 1–6. IEEE.
Ronneberger, O., Fischer, P., and Brox, T. (2015). U-net:
Convolutional networks for biomedical image seg-
mentation. In International Conference on Medical
image computing and computer-assisted intervention,
pages 234–241. Springer.
Russakovsky, O., Deng, J., Su, H., Krause, J., Satheesh, S.,
Ma, S., Huang, Z., Karpathy, A., Khosla, A., Bern-
stein, M., et al. (2015). Imagenet large scale visual
recognition challenge. International journal of com-
puter vision, 115(3):211–252.
Schadendorf, D., van Akkooi, A. C., Berking, C.,
Griewank, K. G., Gutzmer, R., Hauschild, A., Stang,
A., Roesch, A., and Ugurel, S. (2018). Melanoma.
The Lancet, 392(10151):971–984.
Sharma, N. and Ray, A. K. (2006). Computer aided seg-
mentation of medical images based on hybridized ap-
proach of edge and region based techniques. In Proc.
Int. Conf. Math. Biol, pages 150–155.
Yuan, Y. (2017). Automatic skin lesion segmentation with
fully convolutional-deconvolutional networks. arXiv
preprint arXiv:1703.05165.
Yue, D., Guo, M., Chen, Y., and Huang, Y. (2012). A
bayesian decision fusion approach for microrna target
prediction. BMC genomics, 13(8):1–11.
ICT4AWE 2025 - 11th International Conference on Information and Communication Technologies for Ageing Well and e-Health
376