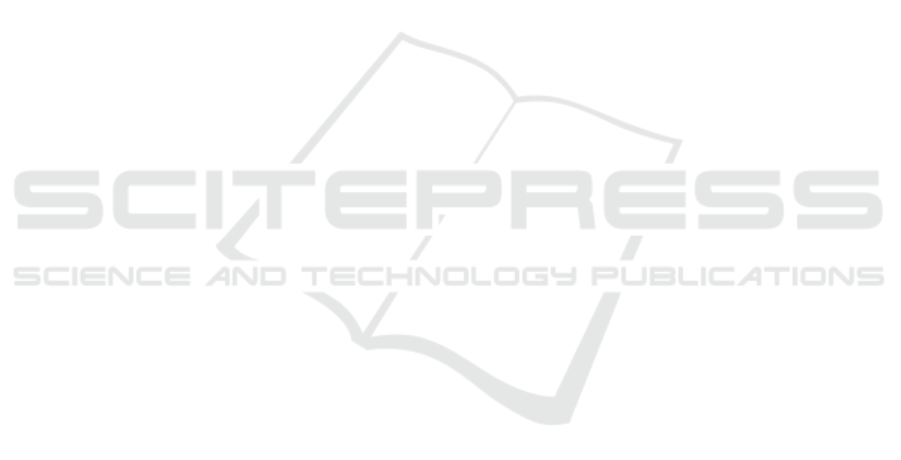
latter facilitates the improvement of the data sources
and enhances overall system reliability.
4 VEHICLE IMPLEMENTATIONS
This section provides more detailed information on
the ISA use case and related vehicle implementations
within the DITM project. The developed concept de-
sign is being implemented into real vehicles (TNO
carlabs) and DRI-elements are deployed and used for
ISA testing under real-life conditions.
4.1 Misbehaviour Detectors
The in-vehicle MBD&R functions are depicted in
Fig. 3 and are divided into two main blocks:
• On-board Unit (OBU) stack: this is part of the
V2X communication system and does checks on
the received data. The low-level communication
function does security checks (V2X-PKI, creden-
tials, integrity), correct forwarding, data consis-
tency check, timing check etc. It can already filter
out untrusted, corrupted messages. Trusted data is
forward into specific MBD and AD functions.
• Automated Driving (AD) stack: this part contains
all in-vehicle AD related functions like sensors,
world model, drive planning and vehicle control.
For ISA it has camera and map MB-detectors and
uses the output coming from the OBU message
detectors. A central function (re-)calculates trust
scores and collects evidence to be used in MBD-
reports. Quality scores are calculated and are used
to determine which speed limit data is used as in-
put for the ISA application.
All received V2X data from DRI elements are pro-
cessed via the ”communication trust” (blue) block of
the OBU stack. This part detects generic communi-
cation misbehaviour with checks possible at all com-
munication layers (ETSI, 2010). The security layer
is the first layer of this detector in which messages
are being assessed based on security-related checks:
trusted source, security profiles, integrity checks, etc.
If the message is not filtered out at this stage, other
checks are performed related to message formats and
structure, consistency and timing checks. If messages
are discarded at this stage, the collected evidence can
be used to file a MB report. For trusted messages,
a trust score is calculated, upon which they are for-
warded to the ITS message detector (red block). The
ITS message detector performs checks based on the
type of message received. For ISA the IVIM is as-
sessed and specific checks on message type, expected
structure, data elements content checks are executed.
Misbehaviour checks are being performed, with spe-
cific interest in road layout information and speed
limit information at road and lane level, to verify if
data are within expected bounds, if data is consistent
over time, etc. The ITS message detector filters out
irrelevant information (for the ISA application) and
forwards the speed limit, road layout information to-
gether with a calculated trust score. In the AD stack,
all in-vehicle related Misbehaviour Detectors are per-
forming similar specific data checks and calculate in-
dividual trust scores. For ISA this are the Camera
Detector and Map Detector (red blocks). First checks
are basic system-related checks to validate healthy
operation, followed by more specific checks on sen-
sor and system data consistency, plausibility, expected
timing of signals, etc. A central block (orange) is pro-
cessing the output from all detectors to calculate an
overall trust score and to collect the evidence for de-
tected misbehaviours, which are being forwarded as
input for potential MB-reporting. In addition, qual-
ity scores are being calculated based on comparing
the different sources (Camera, Map, IVIM) which are
used to determine which speed limit to forward to the
ISA application. The quality score is, again, built-
up of several data-checks (e.g. thresholds), but also
based on comparison of sources, and use of confi-
dence values (if available). In Fig. 3 the green Road
Model block uses these inputs to determine the ap-
plicable speed limit, since ultimately the application
needs a single source-of-truth speed limit to act upon.
4.2 Trust and Quality Score
Calculations
4.2.1 Trust Score
This is a continuation of our work done in (Oliveira,
2024), which already uses trust and quality scores as
part of ISA MBD functionality. A reputation sys-
tem concept is developed targeting all ISA entities, or
nodes (data sources), such as communication chan-
nels, perception systems, possibly other vehicles (via
V2X communication) etc. The used trust scoring con-
cept is based on the theory of reputation scores from
publications of (Michiardi and Molva, 2002), (Lein-
muller et al., 2008) and (Bißmeyer et al., 2012). The
trust scores are intended to track the occurrence of
misbehaviour in each node. A misbehaviour can di-
rectly be linked to a check of a detector, with a detec-
tor covering multiple checks applied in logical rela-
tions. Additionally, the interest is not only in trust at
a specific moment, but more in a historical trust over
a certain window of time. This ensures that false pos-
VEHITS 2025 - 11th International Conference on Vehicle Technology and Intelligent Transport Systems
634