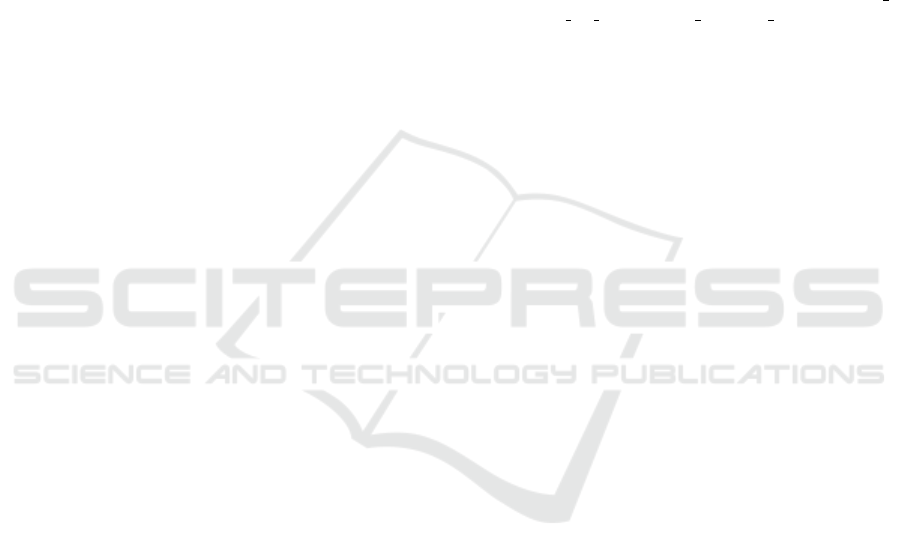
Continual pre-training for cross-lingual llm adapta-
tion: Enhancing Japanese language capabilities. In
Proceedings of the First Conference on Language
Modeling, COLM, pages 1–25, University of Penn-
sylvania, USA, Oct. 2024.
Y. Gao, L. Bing, W. Chen, M. Lyu, and I. King. Diffi-
culty controllable generation of reading comprehen-
sion questions. pages 4968–4974, 08 2019. doi:
10.24963/ijcai.2019/690.
J. Iwasawa, K. Suzuki, and W. Kawakami.
Llama3 preferred medswallow 70b,
2024. URL https://huggingface.co/pfnet/
Llama3-Preferred-MedSwallow-70B.
Y. Kido., H. Yamada., T. Tokunaga., R. Kimura., Y. Miura.,
Y. Sakyo., and N. Hayashi. Automatic question gen-
eration for the Japanese National Nursing Examina-
tion using large language models. In Proceedings of
the 16th International Conference on Computer Sup-
ported Education - Volume 1, pages 821–829. IN-
STICC, SciTePress, 2024. ISBN 978-989-758-697-2.
doi: 10.5220/0012729200003693.
G. Kumar, R. Banchs, and L. D’Haro. Automatic fill-the-
blank question generator for student self-assessment.
pages 1–3, 10 2015. doi: 10.1109/FIE.2015.7344291.
G. Kurdi, J. Leo, B. Parsia, U. Sattler, and S. Al-Emari.
A systematic review of automatic question generation
for educational purposes. International Journal of Ar-
tificial Intelligence in Education, 30:121 – 204, 2020.
M. Lewis, Y. Liu, N. Goyal, M. Ghazvininejad, A. Mo-
hamed, O. Levy, V. Stoyanov, and L. Zettlemoyer.
BART: Denoising sequence-to-sequence pre-training
for natural language generation, translation, and com-
prehension. In D. Jurafsky, J. Chai, N. Schluter, and
J. Tetreault, editors, Proceedings of the 58th Annual
Meeting of the Association for Computational Lin-
guistics, pages 7871–7880, Online, July 2020. Asso-
ciation for Computational Linguistics. doi: 10.18653/
v1/2020.acl-main.703. URL https://aclanthology.org/
2020.acl-main.703.
M. Liu, R. A. Calvo, and V. Rus. Automatic question gen-
eration for literature review writing support. In Inter-
national Conference on Intelligent Tutoring Systems,
2010. URL https://api.semanticscholar.org/CorpusID:
13917826.
Y. Liu, T. Han, S. Ma, J. Zhang, Y. Yang, J. Tian,
H. He, A. Li, M. He, Z. Liu, Z. Wu, L. Zhao,
D. Zhu, X. Li, N. Qiang, D. Shen, T. Liu, and
B. Ge. Summary of chatgpt-related research and per-
spective towards the future of large language mod-
els. Meta-Radiology, 1(2):100017, 2023. ISSN
2950-1628. doi: https://doi.org/10.1016/j.metrad.
2023.100017. URL https://www.sciencedirect.com/
science/article/pii/S2950162823000176.
S. Oh, H. Go, H. Moon, Y. Lee, M. Jeong, H. S. Lee,
and S. Choi. Evaluation of question generation needs
more references. In A. Rogers, J. Boyd-Graber, and
N. Okazaki, editors, Findings of the Association for
Computational Linguistics: ACL 2023, pages 6358–
6367, Toronto, Canada, July 2023. Association for
Computational Linguistics. doi: 10.18653/v1/2023.
findings-acl.396.
N. Okazaki, K. Hattori, H. Shota, H. Iida, M. Ohi, K. Fu-
jii, T. Nakamura, M. Loem, R. Yokota, and S. Mizuki.
Building a large Japanese Web corpus for large lan-
guage models. In Proceedings of the First Conference
on Language Modeling, COLM, pages 1–18, Univer-
sity of Pennsylvania, USA, Oct. 2024.
E. M. Perkoff, A. Bhattacharyya, J. Z. Cai, and J. Cao.
Comparing neural question generation architectures
for reading comprehension. In Proceedings of the 18th
Workshop on Innovative Use of NLP for Building Ed-
ucational Applications (BEA 2023), pages 556–566,
2023.
A. Radford, J. Wu, R. Child, D. Luan, D. Amodei, and
I. Sutskever. Language models are unsupervised
multitask learners. OpenAI, 2019. URL https:
//cdn.openai.com/better-language-models/language
models are unsupervised multitask learners.pdf.
Accessed: 2024-11-15.
C. Raffel, N. Shazeer, A. Roberts, K. Lee, S. Narang,
M. Matena, Y. Zhou, W. Li, and P. J. Liu. Explor-
ing the limits of transfer learning with a unified text-
to-text transformer. J. Mach. Learn. Res., 21(1), Jan.
2020. ISSN 1532-4435.
P. Rajpurkar, J. Zhang, K. Lopyrev, and P. Liang. SQuAD:
100,000+ questions for machine comprehension of
text. In J. Su, K. Duh, and X. Carreras, editors, Pro-
ceedings of the 2016 Conference on Empirical Meth-
ods in Natural Language Processing, pages 2383–
2392, Austin, Texas, Nov. 2016. Association for Com-
putational Linguistics. doi: 10.18653/v1/D16-1264.
URL https://aclanthology.org/D16-1264.
D. Shin and J. H. Lee. Can ChatGPT make reading
comprehension testing items on par with human ex-
perts? Language Learning & Technology, 27(3):27–
40, 2023.
X. Yuan, T. Wang, Y.-H. Wang, E. Fine, R. Abdelghani,
H. Sauz
´
eon, and P.-Y. Oudeyer. Selecting better sam-
ples from pre-trained LLMs: A case study on ques-
tion generation. In A. Rogers, J. Boyd-Graber, and
N. Okazaki, editors, Findings of the Association for
Computational Linguistics: ACL 2023, pages 12952–
12965, Toronto, Canada, July 2023. Association for
Computational Linguistics. doi: 10.18653/v1/2023.
findings-acl.820.
AIG 2025 - Special Session on Automatic Item Generation
764