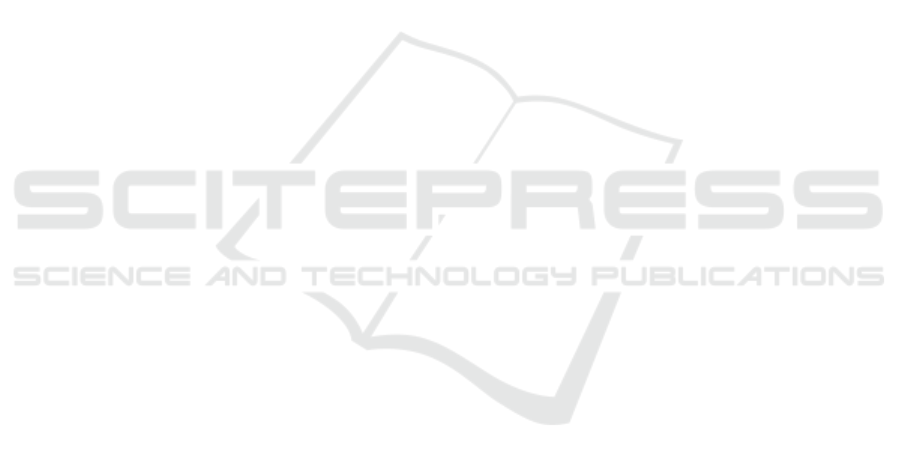
5 CONCLUSIONS
Based on our findings, the current model demon-
strates acceptable performance in recognizing facial
emotions during video game testing. However, there
is considerable potential for improvement.
One key area for enhancement is the incorporation
of facial landmarks in future evaluations. These land-
marks can provide more detailed information about
facial expressions, which could significantly improve
the model’s accuracy.
Additionally, fine-tuning the model parameters
through more extensive training and validation could
further enhance its performance by reducing false
positive rates. When compared to the RANDA model,
our current model exhibits lower accuracy, underscor-
ing the necessity for additional optimizations.
These optimizations might include refining the
feature extraction process, experimenting with differ-
ent machine learning algorithms, or employing more
sophisticated data augmentation techniques to better
handle the variability in facial expressions. By ad-
dressing these areas, we aim to achieve precision lev-
els that are comparable to, or even surpass, those of
the RANDA model.
Moreover, conducting more comprehensive test-
ing with a larger and more diverse dataset could help
identify specific weaknesses and areas for further re-
finement. (Burga-Gutierrez et al., 2020) Continuous
iteration and feedback from real-world testing scenar-
ios will be crucial in evolving our model to meet the
high standards required for effective emotion recog-
nition in video game development.
Looking forward, we aim to integrate our model
into a computer application designed for real-time
analysis of facial emotions during video game testing.
(Guillermo et al., 2023) This application will lever-
age the improved accuracy and reduced false positive
rates achieved through incorporating facial landmarks
and fine-tuning model parameters.
By enabling real-time emotion detection, this tool
could provide invaluable insights into player experi-
ences, helping developers identify areas of frustra-
tion, excitement, or disengagement. (de Rivero et al.,
2023) This immediate feedback can streamline the de-
velopment process, allowing for timely adjustments
to improve overall game design and user experience.
The development of this application will also
involve optimizing the model’s computational effi-
ciency to ensure it operates effectively within the con-
straints of real-time processing during video game
testing sessions.
REFERENCES
Blom, P. M., Bakkes, S., Tan, C. T., Whiteson, S., Roijers,
D. M., Valenti, R., and Gevers, T. (2014). Towards
personalised gaming via facial expression recognition.
In Horswill, I. and Jhala, A., editors, Proceedings of
the Tenth AAAI Conference on Artificial Intelligence
and Interactive Digital Entertainment, AIIDE 2014,
October 3-7, 2014, North Carolina State University,
Raleigh, NC, USA. AAAI.
Burga-Gutierrez, E., Vasquez-Chauca, B., and Ugarte, W.
(2020). Comparative analysis of question answering
models for HRI tasks with NAO in spanish. In SIM-
Big, volume 1410 of Communications in Computer
and Information Science, pages 3–17. Springer.
Chaturvedi, I., Cambria, E., Welsch, R. E., and Herrera, F.
(2018). Distinguishing between facts and opinions for
sentiment analysis: Survey and challenges. Inf. Fu-
sion, 44:65–77.
de Rivero, M., Tirado, C., and Ugarte, W. (2023). Formal-
styler: Gpt-based model for formal style transfer with
meaning preservation. SN Comput. Sci., 4(6):739.
Dumas, J. S. and Redish, J. C. (1993). A practical guide to
usability testing. Intellect.
El-Nasr, M. S., Drachen, A., and Canossa, A., editors
(2013). Game Analytics, Maximizing the Value of
Player Data. Springer.
Guillermo, L., Rojas, J., and Ugarte, W. (2023). Emotional
3d speech visualization from 2d audio visual data.
Int. J. Model. Simul. Sci. Comput., 14(5):2450002:1–
2450002:17.
Kit, N. C., Ooi, C.-P., Tan, W. H., Tan, Y.-F., and Cheong,
S.-N. (2023). Facial emotion recognition using deep
learning detector and classifier. International Jour-
nal of Electrical and Computer Engineering (IJECE),
13(3):3375–3383.
Lin, W., Li, C., and Zhang, Y. (2023). A system of emo-
tion recognition and judgment and its application in
adaptive interactive game. Sensors, 23(6):3250.
Nacke, L. and Drachen, A. (2011). Towards a framework of
player experience research (pre-print). In Foundations
of Digital Games Conference.
Politowski, C., Gu
´
eh
´
eneuc, Y., and Petrillo, F. (2022). To-
wards automated video game testing: Still a long way
to go. In 6th IEEE/ACM International Workshop on
Games and Software Engineering, GAS@ICSE, Pitts-
burgh, PA, USA, May 20, 2022, pages 37–43. ACM.
Vedantham, R. and Reddy, E. S. (2023). Facial emotion
recognition on video using deep attention based bidi-
rectional LSTM with equilibrium optimizer. Multim.
Tools Appl., 82(19):28681–28711.
Wang, Y., Song, W., Tao, W., Liotta, A., Yang, D., Li, X.,
Gao, S., Sun, Y., Ge, W., Zhang, W., and Zhang, W.
(2022). A systematic review on affective computing:
emotion models, databases, and recent advances. Inf.
Fusion, 83-84:19–52.
Emotionalyzer: Player’s Facial Emotion Recognition ML Model for Video Game Testing Automation
943