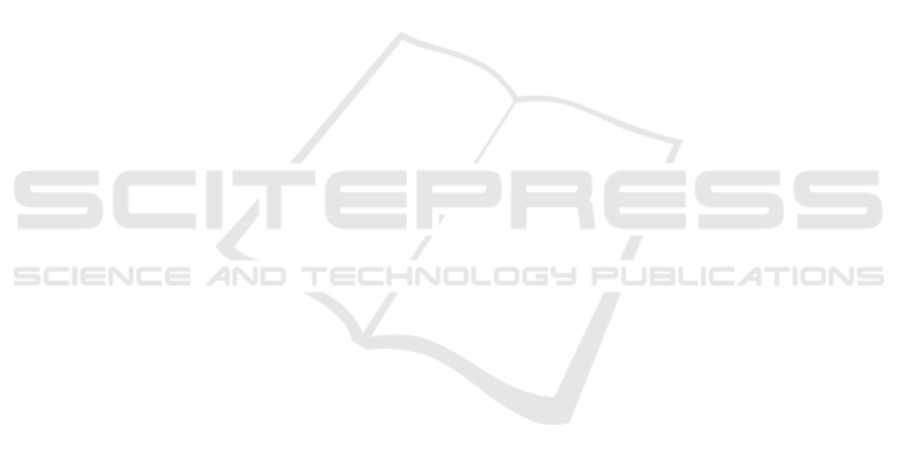
mance metrics, tenure progression, and fluctua-
tions in workload can provide deeper insights into
the dynamics influencing turnover.
• Additional Features. Introducing new variables
like employee engagement scores, satisfaction
surveys, training participation, and career devel-
opment opportunities can enrich the dataset and
enhance model accuracy.
• Longitudinal Analysis. Employing longitudinal
studies to track employee behavior over extended
periods may uncover latent factors contributing to
turnover, enabling more proactive interventions.
• External Factors. Including external data such as
economic indicators, industry trends, and regional
employment rates can help contextualize turnover
patterns within the broader market environment.
By expanding the feature set and integrating tem-
poral aspects, future models can achieve improved
generalization and predictive performance. These
enhancements would allow organizations to iden-
tify at-risk employees more accurately and imple-
ment targeted retention strategies, ultimately reducing
turnover rates and associated costs.
REFERENCES
Adeusi, K., Amajuoyi, P., and Benjami, L. (2024). Utiliz-
ing machine learning to predict employee turnover in
high-stress sectors. International Journal of Manage-
ment & Entrepreneurship Research, 6:1702–1732.
Akiba, T., Sano, S., Yanase, T., Ohta, T., and Koyama, M.
(2019). Optuna: A Next-generation Hyperparameter
Optimization Framework.
Al Akasheh, M., Hujran, O., Malik, E. F., and Zaki,
N. (2024). Enhancing the prediction of employee
turnover with knowledge graphs and explainable ai.
IEEE Access.
Chakraborty, R., Mridha, K., Shaw, R. N., and Ghosh, A.
(2021). Study and prediction analysis of the employee
turnover using machine learning approaches. In 2021
IEEE 4th International Conference on Computing,
Power and Communication Technologies (GUCON),
pages 1–6. IEEE.
Chawla, N. V., Bowyer, K. W., Hall, L. O., and Kegelmeyer,
W. P. (2011). SMOTE: Synthetic Minority Over-
sampling Technique.
Emerson, D. J., Hair Jr, J. F., and Smith, K. J. (2023).
Psychological distress, burnout, and business student
turnover: The role of resilience as a coping mecha-
nism. Research in higher education, 64(2):228–259.
Gopinath, K. and Appavu alias Balamurugan, S. (2024).
Human Resource Analytics: Leveraging Machine
Learning for Employee Attrition Prediction. pages
137–158.
Hancock, J. I., Allen, D. G., Bosco, F. A., McDaniel, M.,
and Pierce, C. A. (2013). Meta-analytic review of em-
ployee turnover as a predictor of firm performance.
Journal of Management, 39(3):573–603.
Li, H., Yuan, B., Yu, Y., Li, J., and Meng, Q. (2022).
Work motivation of primary health workers in china:
the translation of a measurement scale and its corre-
lation with turnover intention. Risk Management and
Healthcare Policy, pages 1369–1381.
Lim, C. S., Malik, E. F., Khaw, K. W., Alnoor, A., Chew, X.,
Chong, Z. L., and Al Akasheh, M. (2024). Hybrid ga–
deepautoencoder–knn model for employee turnover
prediction. Statistics, Optimization & Information
Computing, 12(1):75–90.
Mar
´
ın D
´
ıaz, G., Gal
´
an Hern
´
andez, J. J., and Gald
´
on Sal-
vador, J. L. (2023). Analyzing Employee Attrition
Using Explainable AI for Strategic HR Decision-
Making. 11(22):4677.
Morelli, C., Fusai, G., and Zenti, R. (2024). Who and
why will leave me? Utilizing Machine Learning-
Based Models to Anticipate and Manage Employee
Turnover. (4744130).
Ozdemir, F., Coskun, M., Gezer, C., and Gungor, V. C.
(2020). Assessing employee attrition using classifi-
cations algorithms. In Proceedings of the 2020 the 4th
International Conference on Information System and
Data Mining, ICISDM ’20, page 118–122, New York,
NY, USA. Association for Computing Machinery.
Park, J., Feng, Y., and Jeong, S.-P. (2024). Developing an
advanced prediction model for new employee turnover
intention utilizing machine learning techniques. Sci-
entific Reports, 14(1):1221.
Tsaousoglou, K. (2021). Using psychometrics in
Human Resource Management in tourist accom-
modation: Employees’ predisposition for organi-
zational turnover. PhD thesis, Πανεπιστ
´
ηµιo
Πατρ
´
ων. Σχoλ
´
η ∆ιo
´
ικησης και Oικoνoµ
´
ιας.
Tµ
´
ηµα ∆ιo
´
ικησης . . . .
Veglio, V., Romanello, R., and Pedersen, T. (2024). Em-
ployee turnover in multinational corporations: A su-
pervised machine learning approach.
Vieira, A. G., de Jesus, A. C. C., da Assunc¸
˜
ao Almeida de
Lima, J., Mariano, R. V. R., de Castro, G. Z., and
Brand
˜
ao, W. C. (2023). A probabilistic mapping ap-
proach to assess the employee behavior profile. pages
1–8. ISSN: 2378-1971.
Yi
˘
git,
˙
I. O. and Shourabizadeh, H. (2017). An approach
for predicting employee churn by using data mining.
In 2017 International Artificial Intelligence and Data
Processing Symposium (IDAP), pages 1–4.
ICEIS 2025 - 27th International Conference on Enterprise Information Systems
592