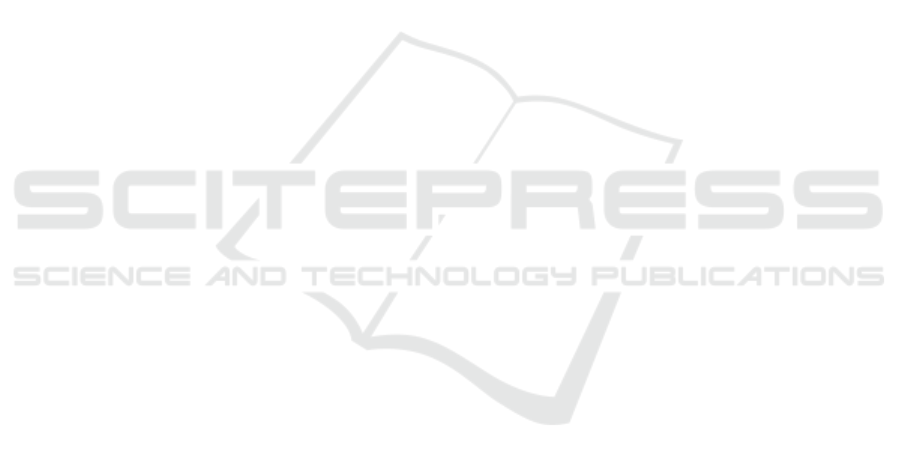
5 LIMITATIONS AND FUTURE
WORK
Our implementation is limited by the lack of high-
quality reflection feedback data for training and fine-
tuning. Enlisting trained human graders to provide
gold-standard data would help establish a stronger
benchmark for LLM-generated feedback.
Another challenge is the use of OpenAI’s GPT
model, which, while powerful, operates as a black-
box system. Unlike rule-based AI, its feedback gen-
eration lacks transparency, making theoretical sound-
ness difficult to verify. As such, it is best suited for
formative feedback, complemented by human review.
Lastly, using a third-party LLM raises privacy
concerns. To mitigate risks, we restricted reflec-
tions to coursework-related content, excluding per-
sonal data. However, one student still cited privacy
concerns as a reason for avoiding the tool.
6 CONCLUSIONS
By leveraging LLMs, we developed an automated
feedback system that provided students with timely,
personalized insights on their reflections. Findings
from the CS4350 course study show that the sys-
tem significantly improved reflection quality, with
students appreciating its concrete suggestions and
specific feedback. While some noted minor draw-
backs—such as occasional rigidity and formulaic re-
sponses—the overall reception was highly positive,
reinforcing its value in the learning process.
Survey results further highlight the benefits of
reflective writing, with students reporting increased
self-awareness, improved teamwork, better project
management, and enhanced technical skills. These
findings affirm the value of reflective learning in
project based courses. Integrating automated feed-
back can further enrich student learning, provided
that reflective tasks are scheduled thoughtfully to en-
sure meaningful engagement without adding exces-
sive workload.
REFERENCES
Bhojan, A. and Hu, Y. (2024). Play testing and reflective
learning ai tool for creative media courses. In Pro-
ceedings of the 16th CSEDU - Volume 1, pages 146–
158. INSTICC, SciTePress.
Boud, D., Keogh, R., and Walker, D. (1985). Promoting
reflection in learning: A model. In Reflection: Turning
Reflection into Learning. Routledge, London.
Bourner, T. (2003). Assessing reflective learning. Educa-
tion + Training, 45(5):267–272.
Chan, C. and Lee, K. (2021). Reflection literacy: A
multilevel perspective on the challenges of using re-
flections in higher education through a comprehen-
sive literature review. Educational Research Review,
32:100376.
Dewey, J. (1933). How We Think: A Restatement of the Re-
lation of Reflective Thinking to the Educative Process.
D.C. Heath and Company, New York.
Fleck, R. and Fitzpatrick, G. (2010). Reflecting on reflec-
tion: framing a design landscape. In Proceedings of
the 22nd Conference of the Computer-Human Interac-
tion Special Interest Group of Australia on Computer-
Human Interaction, OZCHI ’10, page 216–223, New
York, NY, USA. Association for Computing Machin-
ery.
Gibbs, G. and Unit, G. B. F. E. (1988). Learning by Doing:
A Guide to Teaching and Learning Methods. FEU.
Gibson, A., Aitken, A., and et al. (2017). Reflective writ-
ing analytics for actionable feedback. In Proceed-
ings of the Seventh International Learning Analytics
& Knowledge Conference, LAK ’17, page 153–162,
New York, NY, USA. Association for Computing Ma-
chinery.
Han, J., Yoo, H., Myung, J., and et al. (2024). Llm-as-a-
tutor in efl writing education: Focusing on evaluation
of student-llm interaction. KAIST, South Korea.
Knight, S., Shibani, and et al. (2020). Acawriter: A learn-
ing analytics tool for formative feedback on academic
writing. Journal of Writing Research, 12:141–186.
Kolb, D. A. (1984). Experiential Learning: Experience as
the Source of Learning and Development. FT Press.
Mezirow, J. (1991). Transformative Dimensions of Adult
Learning. Jossey-Bass, San Francisco.
Rolfe, G., Freshwater, D., and Jasper, M. (2001). Critical
Reflection for Nursing and the Helping Professions: A
User’s Guide. Palgrave MacMillan.
Schön, D. A. (1984). The Reflective Practitioner: How
Professionals Think in Action, volume 5126. Basic
Books.
Solopova, V., Rostom, E., and et al. (2023). Papagai: Au-
tomated feedback for reflective essays. In Seipel, D.
and Steen, A., editors, KI 2023: Advances in Artificial
Intelligence, pages 198–206, Cham. Springer Nature
Switzerland.
Stahl, M., Biermann, L., Nehring, A., and Wachsmuth, H.
(2024). Exploring llm prompting strategies for joint
essay scoring and feedback generation. Leibniz Uni-
versity Hannover.
Tan, K. Q. J. (2022). Playtesting and reflective learning tool
for creative media courses. B. Comp. Dissertation,
Project Number H1352060, 2021/2022.
Tsingos, C., Bosnic-Anticevich, S., Lonie, J. M., and Smith,
L. (2015). A model for assessing reflective practices
in pharmacy education. American Journal of Pharma-
ceutical Education, 79:124.
Yuan, W., Liu, P., and Gallé, M. (2024). Llmcrit: Teaching
large language models to use criteria.
iReflect: Enhancing Reflective Learning with LLMs: A Study on Automated Feedback in Project Based Courses
403