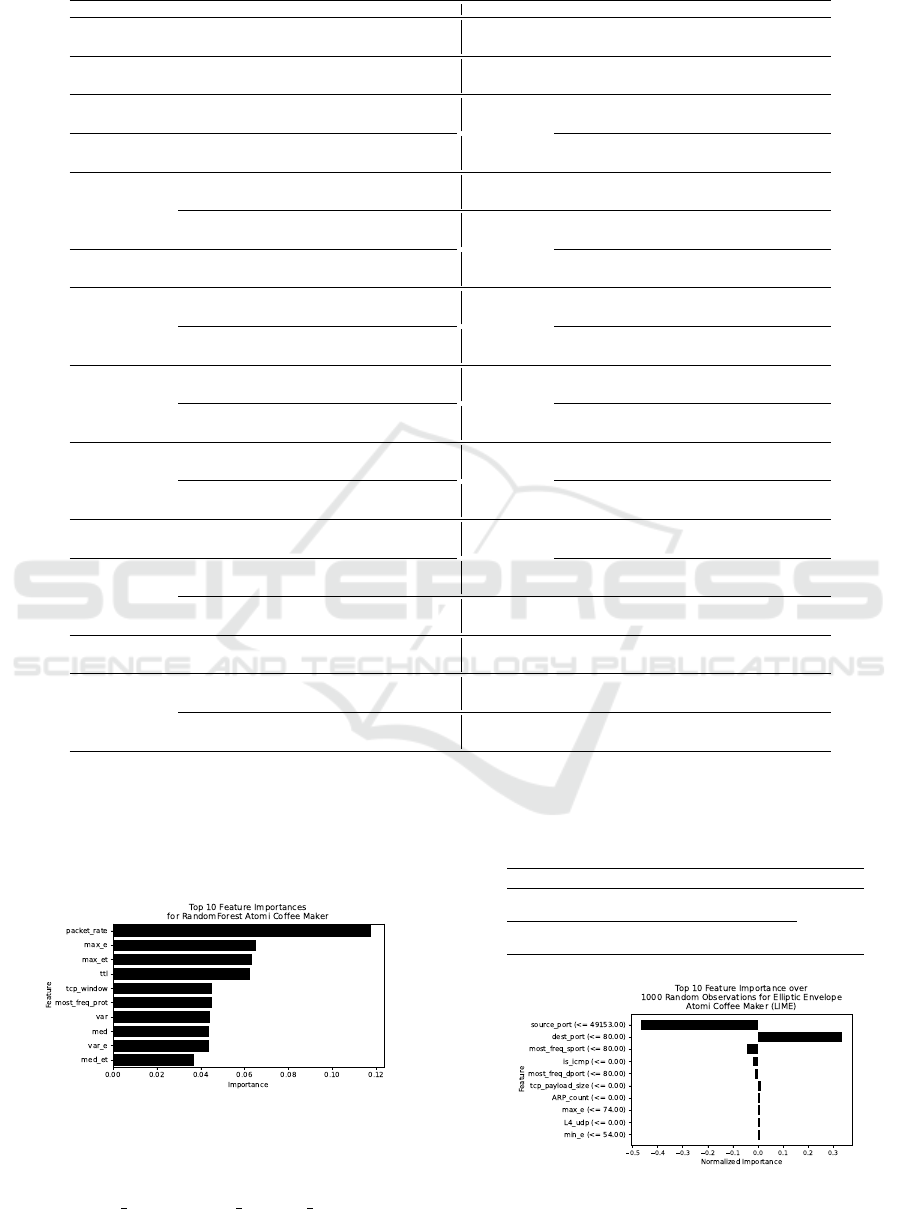
Table 4: Results of the experiments.
Device Scenarios Model Accuracy Precision Recall F-measure Device Scenarios Model Accuracy Precision Recall F-measure
Amazon Echo Dot 8
RF 0,985 0,985 0,985 0,985
HeimVision Lamp 20
RF 0,943 0,944 0,943 0,943
CB 0,981 0,981 0,981 0,981 CB 0,930 0,932 0,930 0,930
XGB 0,986 0,986 0,986 0,986 XGB 0,949 0,950 0,949 0,949
Amazon Echo Spot 8
RF 0,982 0,982 0,982 0,982
Home Eye Camera 6
RF 0,999 0,999 0,999 0,999
CB 0,979 0,979 0,979 0,979 CB 0,999 0,999 0,999 0,999
XGB 0,980 0,980 0,980 0,980 XGB 0,999 0,999 0,999 0,999
Amazon Echo Studio 8
RF 0,991 0,991 0,991 0,991
Luohe Camera
4
RF 0,999 0,999 0,999 0,999
CB 0,990 0,990 0,990 0,990 CB 0,999 0,999 0,999 0,999
XGB 0,989 0,989 0,989 0,989 XGB 0,999 0,999 0,999 0,999
Amazon Plug 8
RF 0,999 0,999 0,999 0,999
2
IF 0,944 0,952 0,944 0,945
CB 0,999 0,999 0,999 0,999 EE 0,935 0,946 0,935 0,936
XGB 0,999 0,999 0,999 0,999 1-SVM 0,936 0,946 0,936 0,937
Amcrest Camera
5
RF 0,999 0,999 0,999 0,999
Nest Camera 2
RF 0,922 0,922 0,922 0,922
CB 0,999 0,999 0,999 0,999 CB 0,927 0,927 0,927 0,927
XGB 0,999 0,999 0,999 0,999 XGB 0,881 0,881 0,881 0,881
2
IF 0,772 0,774 0,772 0,772
Netatmo Camera
5
RF 1,000 1,000 1,000 1,000
EE 0,639 0,689 0,639 0,613 CB 0,999 0,999 0,999 0,999
1-SVM 0,605 0,661 0,605 0,568 XGB 1,000 1,000 1,000 1,000
Arlo Basestation Camera 6
RF 0,996 0,996 0,996 0,996
2
IF 0,994 0,994 0,994 0,993
CB 0,997 0,997 0,997 0,997 EE 0,533 0,957 0,533 0,660
XGB 0,997 0,997 0,997 0,997 1-SVM 0,382 0,953 0,382 0,512
ArloQ Camera
9
RF 0,999 0,999 0,999 0,999
Philips Hue Bridge
11
RF 0,999 0,999 0,999 0,999
CB 0,999 0,999 0,999 0,999 CB 0,999 0,999 0,999 0,999
XGB 0,999 0,999 0,999 0,999 XGB 0,999 0,999 0,999 0,999
2
IF 0,977 0,978 0,977 0,976
2
IF 0,979 0,980 0,979 0,979
EE 0,704 0,892 0,704 0,753 EE 0,981 0,982 0,981 0,981
1-SVM 0,988 0,988 0,988 0,988 1-SVM 0,310 0,763 0,310 0,300
Atomi Coffee Maker
13
RF 0,994 0,994 0,994 0,994
Ring Basestation
11
RF 0,994 0,994 0,994 0,994
CB 0,993 0,993 0,993 0,993 CB 0,994 0,994 0,994 0,994
XGB 0,993 0,993 0,993 0,993 XGB 0,994 0,994 0,994 0,994
2
IF 0,960 0,962 0,960 0,959
2
IF 0,957 0,960 0,957 0,956
EE 0,976 0,977 0,976 0,976 EE 0,975 0,976 0,975 0,974
1-SVM 0,673 0,821 0,673 0,696 1-SVM 0,969 0,969 0,969 0,968
Borun Camera
7
RF 0,999 0,999 0,999 0,999
Roomba Vacuum
13
RF 0,997 0,997 0,997 0,997
CB 0,999 0,999 0,999 0,999
CB 0,996 0,996 0,996 0,996
XGB 0,999 0,999 0,999 0,999 XGB 0,996 0,996 0,996 0,996
2
IF 0,616 0,807 0,616 0,644
2
IF 0,961 0,963 0,961 0,960
EE 0,976 0,977 0,976 0,976 EE 0,971 0,972 0,971 0,971
1-SVM 0,193 0,046 0,193 0,075 1-SVM 0,833 0,866 0,833 0,840
DLink Camera 3
RF 1,000 1,000 1,000 1,000
SimCam
6
RF 0,999 0,999 0,999 0,999
CB 1,000 1,000 1,000 1,000 CB 0,999 0,999 0,999 0,999
XGB 1,000 1,000 1,000 1,000 XGB 0,999 0,999 0,999 0,999
Globe Lamp
21
RF 0,992 0,992 0,992 0,992
2
IF 0,977 0,978 0,977 0,976
CB 0,991 0,991 0,991 0,991 EE 0,988 0,988 0,988 0,988
XGB 0,992 0,993 0,992 0,992 1-SVM 0,253 0,792 0,253 0,293
2
IF 0,939 0,945 0,939 0,938
Smart Board 3
RF 1,000 1,000 1,000 1,000
EE 0,379 0,172 0,379 0,232 CB 0,999 0,999 0,999 0,999
1-SVM 0,346 0,211 0,346 0,229 XGB 0,999 0,999 0,999 0,999
Google Nest Mini 8
RF 0,998 0,998 0,998 0,998
Sonos One Speaker 4
RF 0,999 0,999 0,999 0,999
CB 0,997 0,997 0,997 0,997 CB 0,999 0,999 0,999 0,999
XGB 0,971 0,971 0,971 0,971 XGB 0,999 0,999 0,999 0,999
HeimVision Camera
8
RF 0,999 0,999 0,999 0,999
Tekin Plug 10
RF 0,957 0,957 0,957 0,957
CB 0,999 0,999 0,999 0,999 CB 0,952 0,953 0,952 0,952
XGB 0,999 0,999 0,999 0,999 XGB 0,825 0,834 0,825 0,824
2
IF 0,982 0,982 0,982 0,981
Yutron Plug 10
RF 0,926 0,926 0,926 0,926
EE 0,988 0,988 0,988 0,988 CB 0,908 0,910 0,908 0,908
1-SVM 0,876 0,913 0,876 0,888 XGB 0,918 0,920 0,918 0,918
investigated its efficiency in distinguishing legitimate
network events from malicious. It can be noted, that
all legitimate network events are identified without er-
rors. For malicious data, 3870 events were incorrectly
distinguished as legitimate.
Figure 2: Atomi Coffee Maker: top 10 features for RF.
The results of the feature importance analysis us-
ing LIME are presented in Figure 3. Among the top
10 features, is icmp and tcp payload size are intro-
duced by us, while other features were available in
the initial version of the dataset.
Table 5: Atomi Coffee Maker: classification report for EE.
Class Precision Recall F-measure Support Accuracy
normal 0,970 1,000 0,985 124001
0,976
abnormal 1,000 0,903 0,949 40000
macro avg 0,985 0,952 0,967 164001
weighted avg 0,977 0,976 0,976 164001
Figure 3: Atomi Coffee Maker: top 10 features for EE.
Exploring Efficiency of Machine Learning in Profiling of Internet of Things Devices for Malicious Activity Detection
281