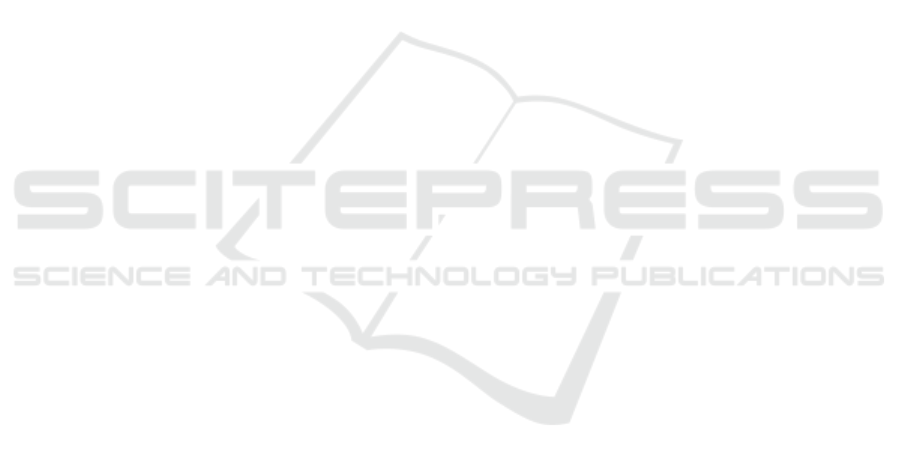
Budzinski, O., Noskova, V., and Zhang, X. (2019). The
brave new world of digital personal assistants: ben-
efits and challenges from an economic perspective.
Netnomics, 20(2-3):177–194.
Drawehn, J. and Pohl, V. (2023). Einsatz von KI mit Fokus
Kundenkommunikation.
Favre, B., Cheung, K., Kazemian, S., Lee, A., Liu, Y.,
Munteanu, C., Nenkova, A., Ochei, D., Penn, G.,
Tratz, S., et al. (2013). Automatic human utility eval-
uation of ASR systems: Does WER really predict per-
formance? In INTERSPEECH, pages 3463–3467.
Fraunhofer IAO (2024). DafNe: Digitaler Außendienst-
Assistent f
¨
ur Nebenzeitoptimierung. Retrieved De-
cember 10, 2024.
Fukuda, M., Nishizaki, H., Iribe, Y., Nishimura, R., and
Kitaoka, N. (2020). Improving Speech Recognition
for the Elderly: A New Corpus of Elderly Japanese
Speech and Investigation of Acoustic Modeling for
Speech Recognition. In Calzolari, N., B
´
echet, F.,
Blache, P., Choukri, K., Cieri, C., Declerck, T., Goggi,
S., Isahara, H., Maegaard, B., Mariani, J., Mazo,
H., Moreno, A., Odijk, J., and Piperidis, S., editors,
Proceedings of the Twelfth Language Resources and
Evaluation Conference, pages 6578–6585, Marseille,
France. European Language Resources Association.
Hannun, A. Y., Case, C., Casper, J., Catanzaro, B., Diamos,
G., Elsen, E., Prenger, R., Satheesh, S., Sengupta, S.,
Coates, A., and Ng, A. Y. (2014). Deep Speech: Scal-
ing up end-to-end speech recognition. arXiv (Cornell
University).
Hoy, M. B. (2018). Alexa, Siri, Cortana, and More: An
Introduction to Voice Assistants. Medical Reference
Services Quarterly, 37(1):81–88.
Huang, H., Tang, T., Zhang, D., Zhao, X., Song, T., Xia, Y.,
and Wei, F. (2023a). Not All Languages Are Created
Equal in LLMs: Improving Multilingual Capability
by Cross-Lingual-Thought Prompting. In Bouamor,
H., Pino, J., and Bali, K., editors, Findings of the Asso-
ciation for Computational Linguistics: EMNLP 2023,
pages 12365–12394, Singapore. Association for Com-
putational Linguistics.
Huang, Q., Tao, M., Zhang, C., An, Z., Jiang, C., Chen, Z.,
Wu, Z., and Feng, Y. (2023b). Lawyer llama technical
report.
Lee, T., Lee, M.-J., Kang, T. G., Jung, S., Kwon, M.,
Hong, Y., Lee, J., Woo, K.-G., Kim, H.-G., Jeong,
J., Lee, J., Lee, H., and Choi, Y. S. (2021). Adapt-
able Multi-Domain Language Model for Transformer
ASR. In ICASSP 2021 - 2021 IEEE International
Conference on Acoustics, Speech and Signal Process-
ing (ICASSP), pages 7358–7362.
Milde, B. and K
¨
ohn, A. (2018). Open source automatic
speech recognition for german.
Pedregosa, F., Varoquaux, G., Gramfort, A., Michel, V.,
Thirion, B., Grisel, O., Blondel, M., Prettenhofer,
P., Weiss, R., Dubourg, V., Vanderplas, J., Passos,
A., Cournapeau, D., Brucher, M., Perrot, M., and
Duchesnay, E. (2011). Scikit-learn: Machine learning
in Python. Journal of Machine Learning Research,
12:2825–2830.
Pereira, R., Lima, C., Pinto, T., and Reis, A. (2023). Vir-
tual Assistants in Industry 4.0: A Systematic Litera-
ture Review. Electronics, 12(19).
Pfeiffer, M. (2024). Comparison of existing technologies
and models for the design of an AI-supported digital
assistance system.
Radford, A., Kim, J. W., Xu, T., Brockman, G., McLeavey,
C., and Sutskever, I. (2022). Robust Speech Recogni-
tion via Large-Scale Weak Supervision.
Trabelsi, A., Warichet, S., Aajaoun, Y., and Soussilane, S.
(2022). Evaluation of the efficiency of state-of-the-art
Speech Recognition engines. Procedia Computer Sci-
ence, 207:2242–2252. Knowledge-Based and Intel-
ligent Information & Engineering Systems: Proceed-
ings of the 26th International Conference KES2022.
Yang, Q., Wang, R., Chen, J., Su, R., and Tan, T. (2024).
Fine-tuning medical language models for enhanced
long-contextual understanding and domain expertise.
Effectiveness of Whisper’s Fine-Tuning for Domain-Specific Use Cases in the Industry
1403