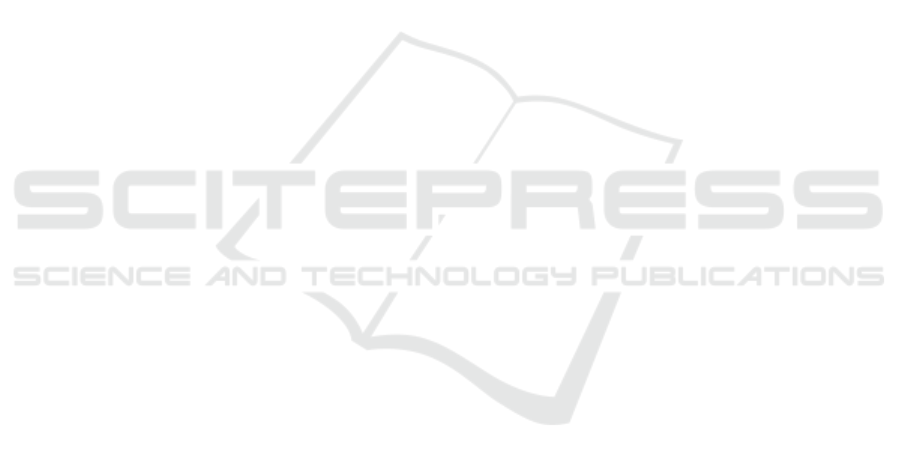
Further limitations come down to the sensitiv-
ity of EEG and EDA data to movement-based arti-
facts. Whilst the sensor set up from Plux biosignals
takes measures to remove movement-based artifacts
through the use of additional sensors and software-
based pattern recognition in post-processing, further
research could be undertaken in order to investigate
how to reduce movement artifacts in VR and MR
EEG or EDA signal requisition.
Future studies should broaden the range of tasks
focus effects are applied to and measured within, as
well as approach novel ways of inducing high focus
states of person through the integration of artificial
intelligence assistance, such as through Meta’s voice
SDK suite or take experimental measures to integrate
real-world use cases into task environments in order
to provide tangible benefits to work in the field. De-
velopment of a modern framework for cognitive load
assessment could assist in opening new avenues of
novel research, now that a variety of qualitative and
quantitative measures exist with efficacy in examin-
ing cognitive load.
7 CONCLUSION AND FUTURE
WORK
This work has examined the effectiveness of visual
and haptic interventions as a method of reducing
cognitive load in mixed reality tasks and environ-
ments. Using combined qualitative and quantitative
data gathering, we measured cognitive load and effec-
tively reduced that load in MR through the combined
use of targeted blurring, directed shadows, and light-
ing and vibration haptics.
Investigation into the individual efficacy of singu-
lar effect based interventions show positive reductions
in cognitive load for directed shadow and lighting as
well as vibration haptics, indicating that these are ap-
propriate methods in reducing the cognitive burden
experienced by MR users in complex tasks. How-
ever, in isolation, the usage of targeted blurring in MR
has been shown to be an area that requires further re-
search, with results suggesting mild quantitative ef-
ficacy but with results suggesting that participants
feel cognitively overwhelmed qualitatively. Results
indicate that whilst mixed reality users experience a
higher cognitive load comparative to fully immersive
VR users, that there are means to mitigate and reduce
this load with the correct software and hardware fo-
cus enhancing measures, usage of targeted shadow
and lighting effects, combined with vibration based
haptics and blurring of non focused objects leads to
a reduced cognitive load in mixed reality propriocep-
tion tasks, leading to experiences with enhanced task
performance for participants as well as offer a more
comfortable and easier to engage with environment.
These findings give room for further investigation. In-
vestigations into the efficacy of visual and haptic in-
terventions can be extended to different types of tasks
such as precision based tasks, memory, engagement
or retention based tasks or similar appropriate objec-
tives.
In future work our research team expects to obtain
the answer to such questions; if reductions in cogni-
tive load are able to be replicated in other types of
task, then there is benefit to improving and imple-
menting focus-enhancing effects within a variety of
potential use cases across the industry.
REFERENCES
Ahmadi, M., Bai, H., Chatburn, A., Najatabadi, M. A.,
W
¨
unsche, B. C., and Billinghurst, M. (2023). Com-
parison of physiological cues for cognitive load mea-
sures in vr. In 2023 IEEE Conference on Virtual Real-
ity and 3D User Interfaces Abstracts and Workshops
(VRW), pages 837–838.
Armougum, A., Orriols, E., Gaston-Bellegarde, A., Marle,
C. J. L., and Piolino, P. (2019). Virtual reality:
A new method to investigate cognitive load during
navigation. Journal of Environmental Psychology,
65:101338.
Azmandian, M., Hancock, M., Benko, H., Ofek, E., and
Wilson, A. D. (2016). Haptic retargeting: Dynamic
repurposing of passive haptics for enhanced virtual re-
ality experiences.
Belani, M., Singh, H. V., Parnami, A., and Singh, P. (2023).
Investigating spatial representation of learning content
in virtual reality learning environments.
Buchwald, M., Kupi
´
nski, S., Bykowski, A., Marcinkowska,
J., Ratajczyk, D., and Jukiewicz, M. (2019). Elec-
trodermal activity as a measure of cognitive load: a
methodological approach. In 2019 Signal Processing:
Algorithms, Architectures, Arrangements, and Appli-
cations (SPA), pages 175–179.
Dan, A. and Reiner, M. (2017). Eeg-based cognitive load
of processing events in 3d virtual worlds is lower than
processing events in 2d displays. International Jour-
nal of Psychophysiology, 122:75–84. Neural Patterns
of Learning, Cognitive Enhancement and Affect.
Gabriel, P., Furtado, F., Hirashima, T., and Hayashi, Y.
(2018). Reducing cognitive load during closed con-
cept map construction and consequences on reading
comprehension and retention.
Hart, S. G. (1988). Development of a multi-dimensional
workload rating scale : Results of empirical and theo-
retical research. Human Mental Workload, 1:39–183.
Huang, C., Luo, Y.-F., Yang, S., Lu, C., and Chen, A.-
S. (2019). Influence of students’ learning style,
HUCAPP 2025 - 9th International Conference on Human Computer Interaction Theory and Applications
516