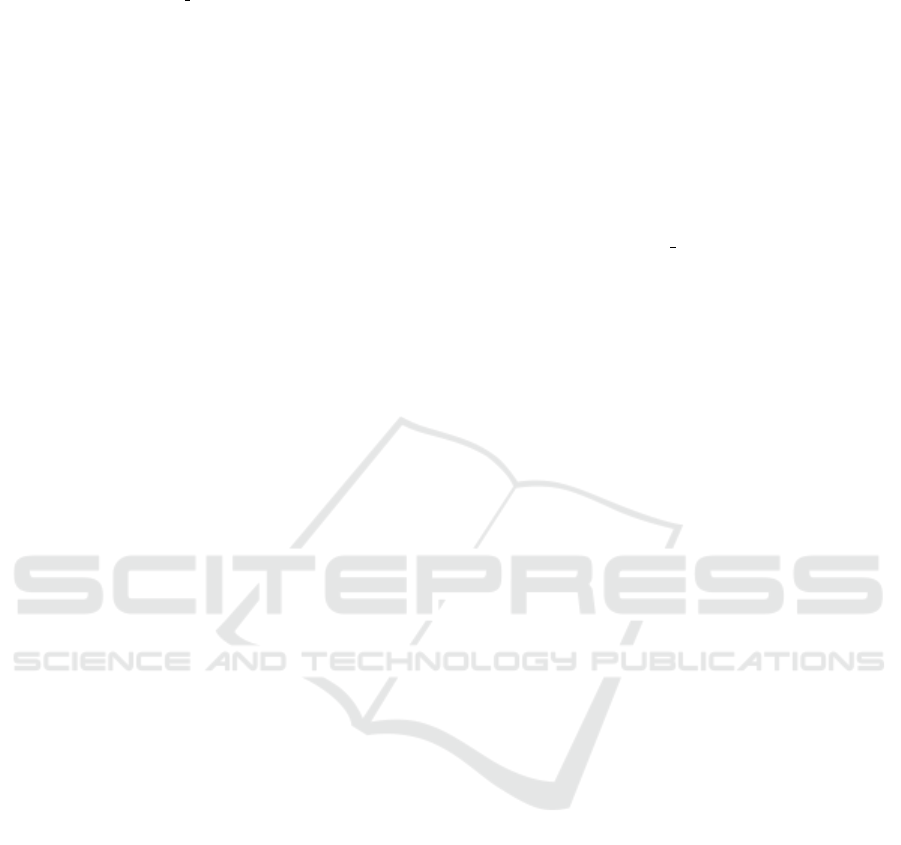
Alsaedi, A., Moustafa, N., Tari, Z., Mahmood, A., and An-
war, A. (2020). Ton iot telemetry dataset: A new gen-
eration dataset of iot and iiot for data-driven intrusion
detection systems. IEEE Access, 8.
ARBAOUI, M., BRAHMIA, M.-E.-A., and RAHMOUN,
A. (2022). Towards secure and reliable aggregation
for federated learning protocols in healthcare appli-
cations. In 2022 Ninth International Conference on
Software Defined Systems (SDS), pages 1–3.
Arbaoui, M., Brahmia, M.-e.-A., Rahmoun, A., and Zghal,
M. (2024). Federated learning survey: A multi-level
taxonomy of aggregation techniques, experimental in-
sights, and future frontiers. ACM Transactions on In-
telligent Systems and Technology.
Brahmia, M.-e.-A., Babouche, S., Ouchani, S., and Zghal,
M. (2022). An adaptive attack prediction framework
in cyber-physical systems. In 2022 Ninth Interna-
tional Conference on Software Defined Systems (SDS),
pages 1–7.
Ferrag, M. A., Friha, O., Hamouda, D., Maglaras, L., and
Janicke, H. (2022). Edge-iiotset: A new comprehen-
sive realistic cyber security dataset of iot and iiot ap-
plications for centralized and federated learning. IEEE
Access, 10:40281–40306.
Ferrag, M. A., Friha, O., Maglaras, L., and Janicke, H.
(2021). Privacy-preserving schemes for fog-enabled
iot: A comprehensive survey. IEEE Internet of Things
Journal, 8(23):16749–16782.
Ghalmane, Z., Brahmia, M. E. A., Zghal, M., and Cher-
ifi, H. (2022). A stochastic approach for extracting
community-based backbones. In International Con-
ference on Complex Networks and Their Applications,
pages 55–67. Springer International Publishing.
Ghalmane, Z., Cherifi, C., Cherifi, H., and El Hassouni,
M. (2019a). Centrality in complex networks with
overlapping community structure. Scientific reports,
9(1):10133.
Ghalmane, Z., Cherifi, C., Cherifi, H., and El Hassouni, M.
(2020). Exploring hubs and overlapping nodes inter-
actions in modular complex networks. IEEE Access,
8:79650–79683.
Ghalmane, Z., Cherifi, C., Cherifi, H., and El Hassouni,
M. (2021). Extracting modular-based backbones in
weighted networks. Information Sciences, 576:454–
474.
Ghalmane, Z., El Hassouni, M., Cherifi, C., and Cherifi, H.
(2018a). K-truss decomposition for modular central-
ity. In 2018 9th International Symposium on Signal,
Image, Video and Communications, pages 241–248.
IEEE.
Ghalmane, Z., El Hassouni, M., and Cherifi, H. (2018b).
Betweenness centrality for networks with non-
overlapping community structure. In 2018 IEEE
workshop on complexity in engineering (COMPENG),
pages 1–5. IEEE.
Ghalmane, Z., El Hassouni, M., and Cherifi, H. (2019b).
Immunization of networks with non-overlapping com-
munity structure. Social Network Analysis and Min-
ing, 9:1–22.
Hamizeh, H., Mugellini, E., Abou Khaled, O., and Cudr
´
e-
Mauroux, P. (2017). Socialmatching++: A novel
approach for interlinking user profiles on social net-
works. Proceedings of the PROFILES@ ISWC 2017:
Sementic web conferene, 22 october 2017, Vienna,
Austria.
Hazimeh, H., Mugellini, E., Ruffieux, S., Khaled, O. A.,
and Cudr
´
e-Mauroux, P. (2018). Automatic embed-
ding of social network profile links into knowledge
graphs. In Proceedings of the 9th international sympo-
sium on information and communication technology,
pages 16–23.
Moustafa, N., Keshky, M. E., Debiez, E., and Janicke, H.
(2020). Federated ton iot windows datasets for evalu-
ating ai-based security applications.
Moustafa, N. and Slay, J. (2015). Unsw-nb15: A compre-
hensive data set for network intrusion detection sys-
tems. In Military Communications and Information
Systems Conference, pages 1–6.
Nukavarapu, S., Ayyat, M., and Nadeem, T. (2022). Mi-
ragenet - towards a gan-based framework for syn-
thetic network traffic generation. Proceedings of IEEE
GLOBECOM, 2022:3089–3095.
Sarhan, M., Layeghy, S., and Portmann, M. (2022). Evalu-
ating standard feature sets towards increased general-
isability and explainability of ml-based network intru-
sion detection. Big Data Research, 30:100359.
Sharafaldin, I., Lashkari, A. H., and Ghorbani, A. A.
(2018). Toward generating a new intrusion detec-
tion dataset and intrusion traffic characterization. In
ICISSP, pages 108–116.
Termos, M., Ghalmane, Z., Fadlallah, A., Jaber, A., and
Zghal, M. (2023). Intrusion detection system for iot
based on complex networks and machine learning. In
2023 IEEE Intl Conf on Dependable, Autonomic and
Secure Computing, pages 471–477. IEEE.
Termos, M., Ghalmane, Z., Fadlallah, A., Jaber, A., and
Zghal, M. (2024). Gdlc: A new graph deep learn-
ing framework based on centrality measures for in-
trusion detection in iot networks. Internet of Things,
26:101214.
Vaccari, I., Chiola, G., Aiello, M., Mongelli, M., and Cam-
biaso, E. (2020). Mqttset, a new dataset for machine
learning techniques on mqtt. Sensors.
IoTBDS 2025 - 10th International Conference on Internet of Things, Big Data and Security
328