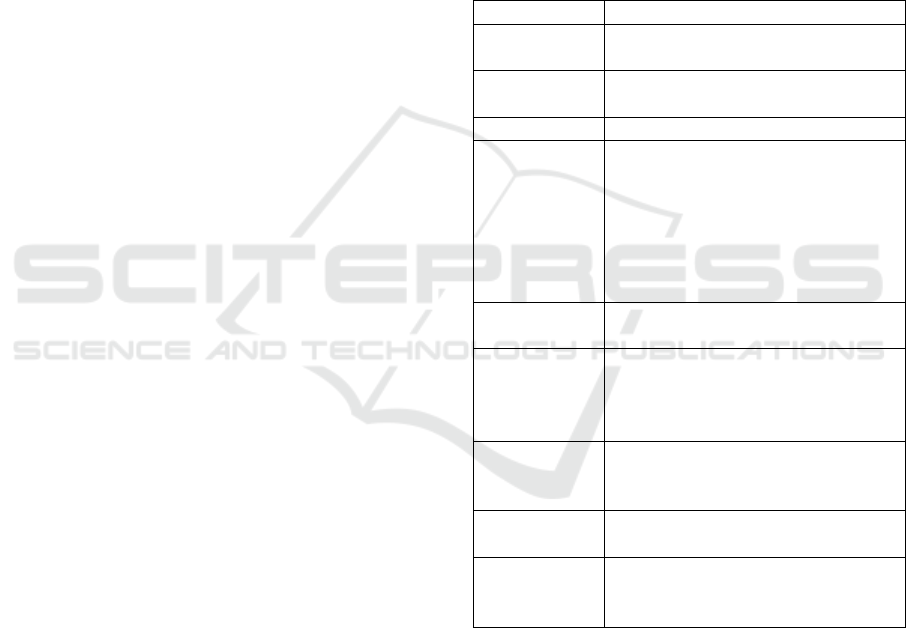
DuBay, W. H. (2007). Smart Language: Readers, Read-
ability, and the Grading of Text. ERIC.
Dunkl, M. (2015). Verst
¨
andlichkeit, pages 41–88. Springer
Fachmedien Wiesbaden, Wiesbaden.
Flesch, R. F. (1948). A new readability yardstick. The Jour-
nal of applied psychology, 32 3:221–33.
Flitcroft, M. A., Sheriff, S. A., Wolfrath, N., Maddula, R.,
McConnell, L., Xing, Y., Haines, K. L., Wong, S. L.,
and Kothari, A. N. (2024). Performance of artificial
intelligence content detectors using human and artifi-
cial intelligence-generated scientific writing. Annals
of Surgical Oncology, pages 1–7.
Foster, A. (2023). Can gpt-4 fool turnitin? testing the limits
of ai detection with prompt engineering.
GPTZero (2024). https://gptzero.me.
Gunning, R. (1952). The Technique of Clear Writing.
McGraw-Hill.
Klare, G. R. (2000). The measurement of readability: use-
ful information for communicators. ACM J. Comput.
Doc., 24(3):107–121.
Krishna, K., Song, Y., Karpinska, M., Wieting, J., and
Iyyer, M. (2023). Paraphrasing evades detectors of
ai-generated text, but retrieval is an effective defense.
In Oh, A., Naumann, T., Globerson, A., Saenko, K.,
Hardt, M., and Levine, S., editors, Advances in Neu-
ral Information Processing Systems, volume 36, pages
27469–27500. Curran Associates, Inc.
Kumarage, T., Sheth, P., Moraffah, R., Garland, J., and
Liu, H. (2023). How reliable are ai-generated-text de-
tectors? an assessment framework using evasive soft
prompts. arXiv preprint arXiv:2310.05095.
Park, C., Kim, H. J., Kim, J., Kim, Y., Kim, T., Cho, H., Jo,
H., Lee, S.-g., and Yoo, K. M. (2024). Investigating
the influence of prompt-specific shortcuts in ai gener-
ated text detection. arXiv preprint arXiv:2406.16275.
Sadasivan, V. S., Kumar, A., Balasubramanian, S., Wang,
W., and Feizi, S. (2023). Can ai-generated text be re-
liably detected? arXiv preprint arXiv:2303.11156.
Singh, A. (2023). A comparison study on ai language de-
tector. In 2023 IEEE 13th Annual Computing and
Communication Workshop and Conference (CCWC),
pages 0489–0493. IEEE.
Spencer, M., Gilmour, A. F., Miller, A. C., Emerson, A. M.,
Saha, N. M., and Cutting, L. E. (2019). Understanding
the influence of text complexity and question type on
reading outcomes. Reading and Writing, 32:603–637.
Taguchi, K., Gu, Y., and Sakurai, K. (2024). The impact of
prompts on zero-shot detection of ai-generated text.
arXiv preprint arXiv:2403.20127.
Tekfi, C. (1987). Readability formulas: An overview. Jour-
nal of documentation, 43(3):261–273.
Thomas, D. G., Hartley, R. D., and Kincaid, J. P. (1975).
Test-retest and inter-analyst reliability of the auto-
mated readability index, flesch reading ease score, and
the fog count. Journal of Reading Behavior, 7(2):149–
154.
Tian, E. and Cui, A. (2024). Gptzero: Towards detection
of ai-generated text using zero-shot and supervised
methods.
Wang, S., Liu, X., and Zhou, J. (2022). Readability is de-
creasing in language and linguistics. Scientometrics,
127:4697–4729.
Weber-Wulff, D., Anohina-Naumeca, A., Bjelobaba, S.,
Folt
`
ynek, T., Guerrero-Dib, J., Popoola, O.,
ˇ
Sigut, P.,
and Waddington, L. (2023). Testing of detection tools
for ai-generated text (arxiv: 2306.15666). arxiv.
Zakaluk, B. L. and Samuels, S. J. (1988). Readability: Its
Past, Present, and Future. ERIC.
ZeroGPT (2024). https://www.zerogpt.com.
APPENDIX
Table 3: Used wordings for the prompt characteristics.
Characteristic Wording
Scientific
language
Write the text in neutral and
factual language
Avoid AI
patterns
generated text is not recognized
by AI detectors
Mistakes insert a few grammatical errors
Explicit
naming of
AI patterns
to be avoided
Avoid typical AI patterns such as
uniform sentence structure,
consistent word choice, perfectly
flowing transitions and
grammatical correctness. Make
your text varied, “imperfect” and a
little less stringent.
Structure omit all headings, introduction
and conclusion/summary
Student
perspective
Master’s student, using natural
language as a person in their
mid-twenties with a Bachelor’s
degree
Stylistic
devices
Occasionally use stylistic devices
such as rhetorical questions,
comparisons or metaphors
Continuous
text
Write a continuous text
Short text Focus only on necessary
information to answer the question
and leave out everything else
You Can’t Detect Me! Using Prompt Engineering to Generate Undetectable Student Answers
311