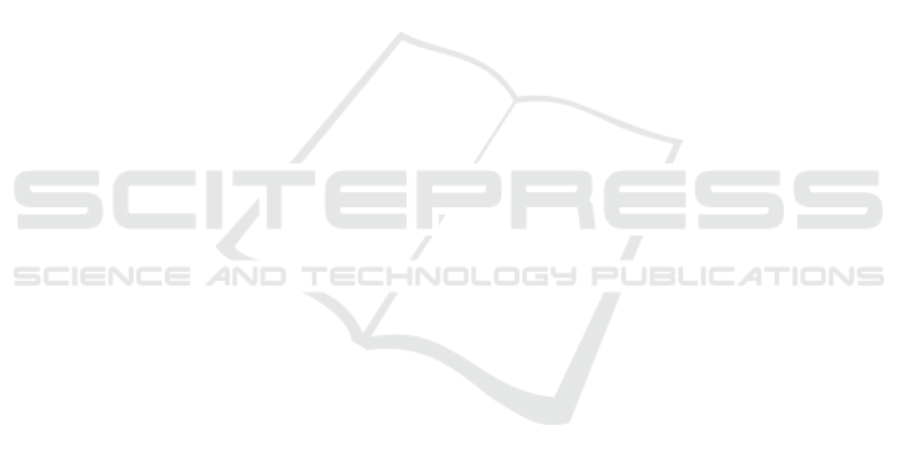
6 THREATS TO VALIDITY
Potential threats to the validity of this study include
the following:
First, we compared our results with the detection
accuracy reported in previous work(Minehisa et al.,
2022) without conducting replication experiments of
prior methods due to time constraints. While the pre-
vious research evaluated a total of 2,805 test data
points, our study was limited to 1,268 test cases that
could be aligned with corresponding source code.
This discrepancy in the evaluation dataset could po-
tentially affect the detection accuracy. Future work
should include replicating the prior methods and eval-
uating them using the exact same dataset to ensure a
fair comparison.
Second, the test data used in this study were la-
beled by the authors of prior research(Liu et al., 2019)
to indicate the presence or absence of naming bugs.
The accuracy of these labels cannot be guaranteed.
Additionally, the criteria for determining naming bugs
that we provided to ChatGPT may differ from those
used in the original labeling. Re-labeling the data
ourselves and aligning the labeling criteria with those
used for ChatGPT would enable a more equitable
comparison.
Lastly, due to time and resource constraints, only
GPT 3.5 Turbo and GPT 4o mini were used as Chat-
GPT models. Higher-performing models, such as
more advanced versions of GPT-4, may yield im-
proved results. Exploring other generative AI services
could also be beneficial. However, given the simplic-
ity of the tasks assigned in this study, it is possible
that the use of more advanced models would not sig-
nificantly affect the accuracy.
7 CONCLUSION
In this study, we used ChatGPT to detect naming
bugs and compared the detection accuracy with that
of conventional methods. In the experiment, the same
evaluation data as in previous research was used, and
in addition to the same detection method as in the
conventional method, we also evaluated cases where
the input was changed and cases where direct binary
classification was performed. In addition, both the
3.5 Turbo and the 4o mini ChatGPT models were
used. As a result, it was found that ChatGPT does
not greatly exceed the detection performance of exist-
ing machine learning models, but that it has detection
performance equivalent to existing methods without
special training, depending on how the information is
given. There was no difference in the ChatGPT mod-
els used, and there was also no significant difference
between the cases where the token sequences were
given to ChatGPT after AST analysis, as in the pre-
vious study, and the cases where the Java source code
was given as is. When ChatGPT was given method
names and processing content to directly detect nam-
ing bugs, it was found to perform at the same level as
previous research when given Java source code and
prompted to detect bugs using stricter criteria.
As a result, using ChatGPT to detect naming bugs
is effective in increasing developer convenience, and
it is possible that naming bugs can be pointed out and
fixed more easily.
Future issues include more detailed evaluation of
existing methods and expanding the naming bug data
used for evaluation. More specifically, comparisons
with recent related research(Wang et al., 2024) that
was not included in this experiment, and comparisons
with other methods that focus on the generation of
method names, are important issues for the future. It
is also possible to consider evaluating other LLMs or
expanding the evaluation to other programming lan-
guages besides Java.
ACKNOWLEDGEMENTS
This work was supported in part by JSPS KAKENHI
Grant number JP23K16863 and JP20H05706.
REFERENCES
Boswell, D. and Foucher, T. (2011). The Art of Readable
Code: Simple and Practical Techniques for Writing
Better Code. Oreilly & Associates.
Bsharat, S. M., Myrzakhan, A., and Shen, Z. (2024). Prin-
cipled instructions are all you need for questioning
llama-1/2, gpt-3.5/4.
Høst, E. W. and Østvold, B. M. (2009). Debugging method
names. In Proceedings of the 23rd European Con-
ference on ECOOP 2009 — Object-Oriented Pro-
gramming, Genoa, page 294–317, Berlin, Heidelberg.
Springer-Verlag.
Liu, K., Kim, D., Bissyand
´
e, T., Taeyoung, K., Kim, K.,
Koyuncu, A., Kim, S., and Le Traon, Y. (2019).
Learning to spot and refactor inconsistent method
names. In in Proc. 41st Int’l Conf. Softw. Eng., pages
1–12.
Martin, R. C. (2008). Clean Code: A Handbook of Agile
Software Craftsmanship. Prentice Hall.
McConnell, S. (2004). Code Complete, Second Edition. Mi-
crosoft Press, USA.
Minehisa, T., Aman, H., and Kawahara, M. (2022). Naming
bug detection using transformer-based method name
Evaluating ChatGPT’s Ability to Detect Naming Bugs in Java Methods
135