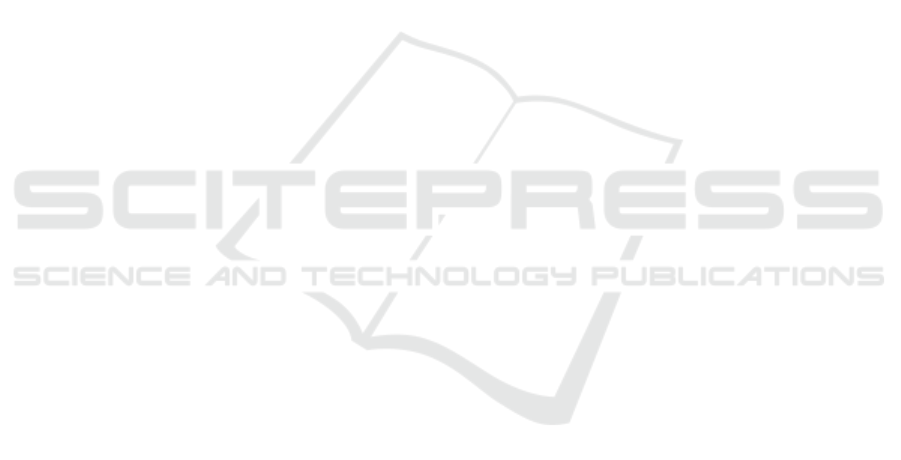
learning with reasoning engines to create a hybrid
system. Machine learning detects patterns, while rea-
soning engines refine predictions using rules or ontol-
ogy-based logic. This improves accuracy and explain-
ability. For example, in a tutoring system, it predicts
student knowledge gaps and adjusts recommenda-
tions based on prerequisite rules. 2. buildDynamic-
Knowledge() keeps the knowledge base up to date by
integrating new data and insights. The system contin-
uously monitors input, updates validated information,
and ensures consistency. In healthcare, it adds new
treatments and diseases dynamically, ensuring diag-
nostic models stay current. 3. scaleReasoning() en-
hances the system’s ability to process large and com-
plex datasets efficiently. Optimization techniques,
such as heuristic algorithms and parallel computing,
improve reasoning performance. In urban planning,
this method analyzes traffic data from sensor net-
works to suggest optimal routes. 4. integrateNeu-
roSymbolicApproaches() combines neural networks
with symbolic reasoning for better decision-making.
Neural networks handle unstructured data, while sym-
bolic reasoning refines predictions for more reliable
results. In legal document analysis, it classifies docu-
ments with AI and validates classifications using le-
gal rules. These methods make the LearningRea-
soningSystem more adaptive, scalable, and capable
of delivering precise and interpretable decisions.
5 CONCLUSION
The CWM framework offers a standardized approach
to metadata management, yet its limited semantic
expressiveness restricts its applicability in complex,
heterogeneous environments. This paper proposes
an extended CWM framework that incorporates on-
tologies, machine learning, and knowledge reinjec-
tion policies to address these limitations. By in-
troducing new layers and components, such as the
”Learning Package” and advanced knowledge map-
ping mechanisms, the framework enhances the se-
mantic interoperability, adaptability, and usability of
data warehouse systems. The research explores the
integration of hybrid AI systems, combining induc-
tive and deductive techniques, to improve knowl-
edge discovery and decision-making. With the ex-
tension of the CWM mentioned in (Ralaivao et al.,
2024) and the present extension, we obtain an ex-
tended CWM framework capable of discovering and
managing knowledge. As a way forward, we pro-
pose experimentation and benchmarking on simple
and knowledge-based queries. Future work will fo-
cus on refining the reinjection mechanism with adap-
tive learning techniques and evaluating its applica-
bility across various domains, such as education and
learning, healthcare, finance, and smart cities.
REFERENCES
Antunes, A. L., Cardoso, E., and Barateiro, J. (2022). In-
corporation of ontologies in data warehouse/business
intelligence systems-a systematic literature review.
2(2):100131.
da Silva, J., de Oliveira, A. G., do Nascimento Fidalgo,
R., Salgado, A. C., and Times, V. C. Modelling and
querying geographical data warehouses. 35:592–614.
Falquet, G., M
´
etral, C., Teller, J., Tweed, C., Roussey, C.,
Pinet, F., Kang, M. A., and Corcho, O. (2011). An
introduction to ontologies and ontology engineering.
Ontologies in Urban development projects, pages 9–
38.
Ghidalia, S., Narsis, O. L., Bertaux, A., and Nicolle,
C. (2024). Combining machine learning and ontol-
ogy: A systematic literature review. arXiv preprint
arXiv:2401.07744.
Gomes, P., Farinha, J. T., and Trigueiros, M. J. A data qual-
ity metamodel extension to cwm. In Asia-Pacific Con-
ference on Conceptual Modelling.
Midouni, S. A. D., Darmont, J., and Bentayeb, F. Approche
de mod
´
elisation multidimensionnelle des donn
´
ees
complexes : application aux donn
´
ees m
´
edicales,. In
Journ
´
ees Francophones sur les Entrep
ˆ
ots de Donn
´
ees
et l’Analyse en ligne.
Olsina, L. (2021). The foundational ontology thingfo: Ar-
chitectural aspects, concepts, and applicability. In In-
ternational Joint Conference on Knowledge Discov-
ery, Knowledge Engineering, and Knowledge Man-
agement, pages 73–99. Springer.
Ralaivao, C., Razafindraibe, F., Raherinirina, A., and Rako-
tonirainy, H. (2024). Cwm extensions for knowl-
edge and metadata integration for complex data ware-
house and big data. In Proceedings of the 26th
International Conference on Enterprise Information
Systems, Angers, France, volume 1, pages 329–336.
SCITEPRESS - Science and Technology Publications.
Tavac, M. and Tavac, V. The general algorithm for the de-
sign of the mda transformation models. pages 171–
176.
Thavornun, V. (2015). Metadata management for knowl-
edge discovery. Master’s thesis.
Zayakin, V. S., Lyadova, L. N., Lanin, V. V., Zamyatina,
E. B., and Rabchevskiy, E. A. (2021). An ontology-
driven approach to the analytical platform develop-
ment for data-intensive domains. In International
Joint Conference on Knowledge Discovery, Knowl-
edge Engineering, and Knowledge Management, vol-
ume 1718, pages 129–149. Springer, Springer Inter-
national Publishing.
ICEIS 2025 - 27th International Conference on Enterprise Information Systems
308