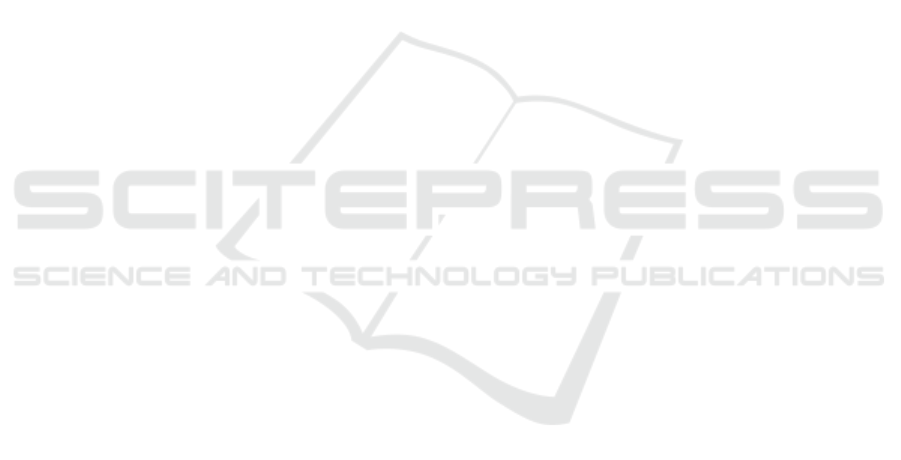
Arppe, D. F., Zaman, L., Pazzi, R. W., and El-Khatib, K.
(2020). Uninet: A mixed reality driving simulator.
In Proceedings of Graphics Interface 2020, GI 2020,
pages 37 – 55. Canadian Human-Computer Commu-
nications Society / Soci
´
et
´
e canadienne du dialogue
humain-machine.
Dosovitskiy, A., Ros, G., Codevilla, F., Lopez, A., and
Koltun, V. (2017). CARLA: An open urban driving
simulator. In Proceedings of the 1st Annual Confer-
ence on Robot Learning, pages 1–16.
Edge, D., Trinh, H., Cheng, N., Bradley, J., Chao, A., Mody,
A., Truitt, S., and Larson, J. (2024). From local to
global: A graph rag approach to query-focused sum-
marization.
Ehrlinger, L. and W
¨
oß, W. (2016). Towards a definition
of knowledge graphs. SEMANTiCS (Posters, Demos,
SuCCESS), 48(1-4):2.
Elmaaroufi, K., Shanker, D., Cismaru, A., Vazquez-
Chanlatte, M., Sangiovanni-Vincentelli, A., Zaharia,
M., and Seshia, S. A. (2024). ScenicNL: Generat-
ing probabilistic scenario programs from natural lan-
guage. In First Conference on Language Modeling.
Feng, J., Du, Y., Liu, T., Guo, S., Lin, Y., and Li, Y. (2024a).
Citygpt: Empowering urban spatial cognition of large
language models.
Feng, J., Zhang, J., Yan, J., Zhang, X., Ouyang, T., Liu,
T., Du, Y., Guo, S., and Li, Y. (2024b). Citybench:
Evaluating the capabilities of large language model as
world model.
Fremont, D. J., Kim, E., Dreossi, T., Ghosh, S., Yue,
X., Sangiovanni-Vincentelli, A. L., and Seshia, S. A.
(2023). Scenic: a language for scenario speci-
fication and data generation. Machine Learning,
112(10):3805–3849.
Gajananan, K., Doirado, E., Nakasone, A., Cuba, P.,
Prendinger, H., and Miska, M. (2011). Creating in-
teractive driver experiences with the scenario markup
language. In Proceedings of the 8th International
Conference on Advances in Computer Entertainment
Technology, ACE ’11, New York, NY, USA. Associa-
tion for Computing Machinery.
Gambi, A., Mueller, M., and Fraser, G. (2019a). Asfault:
Testing self-driving car software using search-based
procedural content generation. In 2019 Ieee/Acm 41st
International Conference On Software Engineering:
Companion Proceedings, pages 27–30. IEEE.
Gambi, A., Mueller, M., and Fraser, G. (2019b). Automat-
ically Testing Self-Driving Cars with Search-Based
Procedural Content Generation, page 318–328. As-
sociation for Computing Machinery, New York, NY,
USA.
Gao, C., Lan, X., Li, N., Yuan, Y., Ding, J., Zhou, Z., Xu, F.,
and Li, Y. (2024). Large language models empowered
agent-based modeling and simulation: a survey and
perspectives. Humanities and Social Sciences Com-
munications, 11(1):1259.
Gieseke, L., Asente, P., M
ˇ
ech, R., Benes, B., and Fuchs,
M. (2021). A survey of control mechanisms for cre-
ative pattern generation. Computer Graphics Forum,
40(2):585–609.
Johansson, R., Williams, D., Berglund, A., and Nugues, P.
(2004). Carsim: A system to visualize written road ac-
cident reports as animated 3d scenes. In Proceedings
of the 2nd Workshop on Text Meaning and Interpreta-
tion, TextMean ’04, USA. Association for Computa-
tional Linguistics.
Li, Q., Peng, Z., Feng, L., Zhang, Q., Xue, Z., and Zhou, B.
(2022). Metadrive: Composing diverse driving sce-
narios for generalizable reinforcement learning. IEEE
Transactions on Pattern Analysis and Machine Intel-
ligence.
Li, Q., Peng, Z., Zhang, Q., Qiu, C., Liu, C., and Zhou,
B. (2020). Improving the generalization of end-to-end
driving through procedural generation. arXiv preprint
arXiv:2012.13681.
Li, S., Azfar, T., and Ke, R. (2024). Chatsumo: Large lan-
guage model for automating traffic scenario genera-
tion in simulation of urban mobility.
Lopez, P. A., Behrisch, M., Bieker-Walz, L., Erdmann, J.,
Fl
¨
otter
¨
od, Y.-P., Hilbrich, R., L
¨
ucken, L., Rummel,
J., Wagner, P., and Wiessner, E. (2018). Microscopic
traffic simulation using sumo. In 2018 21st Interna-
tional Conference on Intelligent Transportation Sys-
tems (ITSC), pages 2575–2582.
Ollama (2024). Get up and running with large language
models. https://github.com/ollama/ollama. Accessed:
2024-10-20.
OpenDRIVE, A. (2024). Asam opendrive. https://www.
asam.net/standards/detail/opendrive. Accessed: 2024-
10-26.
OpenStreetMap (2024). Openstreetmap. https://www.
openstreetmap.org/about. Accessed: 2024-10-26.
pandas (2024). pandas - python data analysis library. https:
//pandas.pydata.org. Accessed: 2024-10-20.
Ramesh, M. and Flohr, F. B. (2024). Walk-the-talk: Llm
driven pedestrian motion generation. In 2024 IEEE In-
telligent Vehicles Symposium (IV), pages 3057–3062.
IEEE.
Risi, S. and Togelius, J. (2020). Increasing generality in ma-
chine learning through procedural content generation.
Nature Machine Intelligence, 2(8):428–436.
Ruan, B.-K., Tsui, H.-T., Li, Y.-H., and Shuai, H.-H. (2024).
Traffic scene generation from natural language de-
scription for autonomous vehicles with large language
model. arXiv preprint arXiv:2409.09575.
Sun, X., Zhang, Y., and Zhou, W. (2021). Building narrative
scenarios for human-autonomous vehicle interaction
research in simulators. In Cassenti, D. N., Scataglini,
S., Rajulu, S. L., and Wright, J. L., editors, Advances
in Simulation and Digital Human Modeling, pages
150–156, Cham. Springer International Publishing.
ˇ
St’ava, O., Bene
ˇ
s, B., M
ˇ
ech, R., Aliaga, D. G., and Kri
ˇ
stof,
P. (2010). Inverse procedural modeling by automatic
generation of l-systems. Computer Graphics Forum,
29(2):665–674.
Zhang, J., Xu, C., and Li, B. (2024). Chatscene:
Knowledge-enabled safety-critical scenario genera-
tion for autonomous vehicles. In 2024 IEEE/CVF
Conference on Computer Vision and Pattern Recog-
nition (CVPR), pages 15459–15469.
GRAPP 2025 - 20th International Conference on Computer Graphics Theory and Applications
346