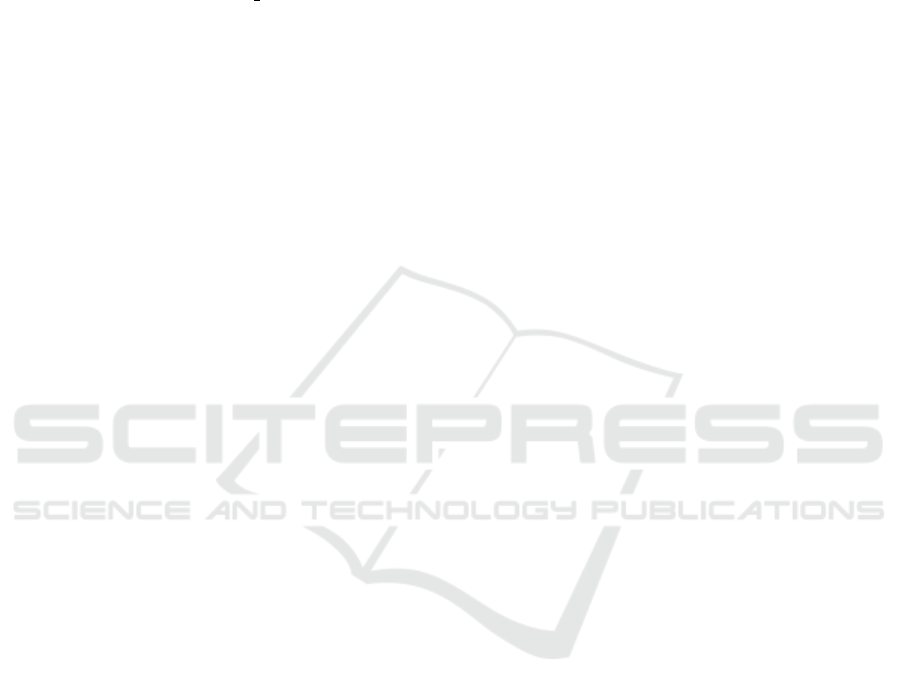
avenue for future research regarding the possibility of
automated optimization of segmentation models.
ACKNOWLEDGEMENT
The ASEAN IVO project
(http://www.nict.go.jp/en/asean ivo/index.html),
titled ‘Visual IoT Network for Environmental
Protection and Disaster Prevention,’ was
involved in the production of the contents of
this work and financially supported by NICT
(http://www.nict.go.jp/en/index.html).
REFERENCES
Barmpoutis, P., Papaioannou, P., Dimitropoulos, K., and
Grammalidis, N. (2020). A review on early forest
fire detection systems using optical remote sensing.
Sensors, 20(22).
Canny, J. (1986). A computational approach to edge
detection. IEEE Transactions on pattern analysis and
machine intelligence, (6):679–698.
Chapin III, F. S., Zavaleta, E., Eviner, V., Naylor, R.,
Vitousek, P., Reynolds, H., Hooper, D., Lavorel, S.,
Sala, O., Hobbie, S., Mack, M., and Diaz, S. (2000).
Consequences of changing biodiversity. Nature,
405:234–42.
Chaturvedi, S., Khanna, P., and Ojha, A. (2021).
Comparative analysis of traditional and deep
learning techniques for industrial and wildfire smoke
segmentation. In 2021 Sixth International Conference
on Image Information Processing (ICIIP), volume 6,
pages 326–331.
Chen, T. and Guestrin, C. (2016). Xgboost: A scalable tree
boosting system. KDD ’16, page 785–794, New York,
NY, USA. Association for Computing Machinery.
Dorogush, A. V., Ershov, V., and Gulin, A. (2018).
Catboost: gradient boosting with categorical features
support.
Gill, A. M., Stephens, S. L., and Cary, G. J. (2013).
The worldwide “wildfire” problem. Ecological
Applications, 23(2):438–454.
Haralick, R. M., Shanmugam, K., and Dinstein, I. (1973).
Textural features for image classification. IEEE
Transactions on Systems, Man, and Cybernetics,
SMC-3(6):610–621.
He, K., Zhang, X., Ren, S., and Sun, J. (2015). Deep
residual learning for image recognition.
Jocher, G. and Qiu, J. (2024). Ultralytics yolo11.
Ke, G., Meng, Q., Finley, T., Wang, T., Chen, W., Ma, W.,
Ye, Q., and Liu, T.-Y. (2017). Lightgbm: A highly
efficient gradient boosting decision tree. Advances in
neural information processing systems, 30.
Khan, S., Muhammad, K., Hussain, T., Ser, J. D., Cuzzolin,
F., Bhattacharyya, S., Akhtar, Z., and de Albuquerque,
V. H. C. (2021). Deepsmoke: Deep learning model
for smoke detection and segmentation in outdoor
environments. Expert Systems with Applications,
182:115125.
Meena, D. (2021). A study on the importance of wildlife.
8.
Natekin, A. and Knoll, A. (2013). Gradient boosting
machines, a tutorial. Frontiers in Neurorobotics, 7.
Pan, S. J. and Yang, Q. (2010). A survey on transfer
learning. IEEE Transactions on Knowledge and Data
Engineering, 22(10):1345–1359.
Pedregosa, F., Varoquaux, G., Gramfort, A., Michel, V.,
Thirion, B., Grisel, O., Blondel, M., M
¨
uller, A.,
Nothman, J., Louppe, G., Prettenhofer, P., Weiss, R.,
Dubourg, V., Vanderplas, J., Passos, A., Cournapeau,
D., Brucher, M., Perrot, M., and
´
Edouard Duchesnay
(2018). Scikit-learn: Machine learning in python.
Pornpholkullapat, N., Phankrawee, W., Boondet, P.,
Thein, T. L. L., Siharath, P., Cruz, J. D., Marata,
K. T., Tungpimolrut, K., and Karnjana, J. (2023).
Firespot: A database for smoke detection in early-
stage wildfires. In 2023 18th International Joint
Symposium on Artificial Intelligence and Natural
Language Processing (iSAI-NLP), pages 1–6. IEEE.
Redmon, J., Divvala, S., Girshick, R., and Farhadi, A.
(2016). You only look once: Unified, real-time object
detection.
Stricker, M. A. and Orengo, M. (1995). Similarity of color
images. In Niblack, W. and Jain, R. C., editors,
Storage and Retrieval for Image and Video Databases
III, volume 2420, pages 381 – 392. International
Society for Optics and Photonics, SPIE.
Sukitpaneenit, M. and Kim Oanh, N. T. (2014). Satellite
monitoring for carbon monoxide and particulate
matter during forest fire episodes in northern
thailand. Environmental Monitoring and Assessment,
186(4):2495–2504.
Swain, M. J. and Ballard, D. H. (1991). Color indexing.
International Journal of Computer Vision, 7:11–32.
Tan, M. and Le, Q. V. (2019). Efficientnet: Rethinking
model scaling for convolutional neural networks.
ArXiv, abs/1905.11946.
Voulodimos, A., Doulamis, N., Doulamis, A., and
Protopapadakis, E. (2018). Deep learning for
computer vision: A brief review. Computational
Intelligence and Neuroscience, 2018(1):7068349.
Zhao, X., Ding, W., An, Y., Du, Y., Yu, T., Li, M., Tang, M.,
and Wang, J. (2023). Fast segment anything.
Zhou, Z., Shi, Y., Gao, Z., and Li, S. (2016). Wildfire smoke
detection based on local extremal region segmentation
and surveillance. Fire Safety Journal, 85:50–58.
IoTBDS 2025 - 10th International Conference on Internet of Things, Big Data and Security
140