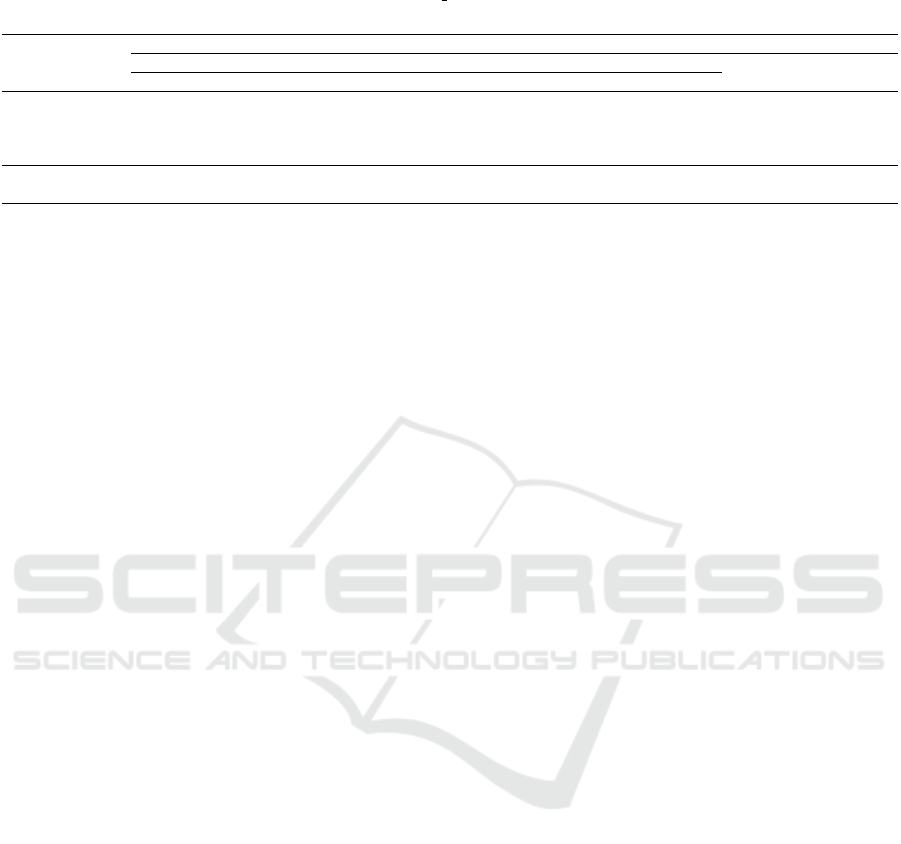
Table 5: The AP and the number of parameters are represented by different design considerations of GIFF. Aggregation Op-
eration represents the “AGG” featured in Eq. \ref{aggregate features}. “Depths” represents the feature map dimensionality
reduction to compute the attention weights. w/IAtten and w/o represent the AP with and without iterative attention.
Experiment No.
AP@IoU=0.5 AP@IoU=0.7 No. of Parameters (M)
w/ IAtten w/o IAtten w/ IAtten w/o IAtten
w/ IAtten w/o IAtten
w/o RSU w/RSU w/o RSU w/RSU w/o RSU w/RSU w/o RSU w/RSU
1 71.28 77.26 68.56 71.81 66.85 73.29 62.88 65.86 16.10 15.39
2 (Default) 73.62 78.93 68.97 72.96 68.37 75.82 63.48 65.94 16.12 15.98
3 68.53 74.33 68.14 71.61 62.76 67.42 62.36 64.41 16.89 16.20
4 67.15 72.14 68.50 72.25 64.32 68.46 63.32 63.74 16.92 16.21
5 68.56 70.05 67.53 70.0 62.68 71.14 61.55 63.52 17.06 16.78
6 69.93 72.78 68.46 70.94 63.12 68.37 63.10 63.10 17.13 16.85
7 CONCLUSION AND FUTURE
WORK
This paper presents GIFF, a graph iterative attention-
based network designed to address collaborative per-
ception challenges in multi-agent systems. GIFF ef-
fectively facilitates multi-agent collaboration by intel-
ligently fusing perceptual information received from
collaborators. It achieves this by learning the relative
importance of collaborators and identifying the spa-
tial regions within the received semantic information
that require higher attention. The iterative attention
mechanism further enhances the refinement of the
attention-learning process. GIFF achieves superior
performance on the object detection task, as demon-
strated on standard benchmarks such as V2XSim
and OPV2V. Despite these promising results, the ap-
proach has significant potential for future improve-
ments. As part of future work, we aim to address the
impact of transmission delays caused by communica-
tion network characteristics, which hinder the perfor-
mance of collaborative perception.
REFERENCES
Ahmed, A. N., Anwar, A., Mercelis, S., Latr
´
e, S., and
Hellinckx, P. (2021). Ff-gat: Feature fusion using
graph attention networks. In IECON 2021–47th An-
nual Conference of the IEEE Industrial Electronics
Society, pages 1–6. IEEE.
Ahmed, A. N., Mercelis, S., and Anwar, A. (2024a). Col-
labgat: Collaborative perception using graph attention
network. IEEE Access.
Ahmed, A. N., Mercelis, S., and Anwar, A. (2024b). Graph
attention based feature fusion for collaborative per-
ception. In 2024 IEEE Intelligent Vehicles Symposium
(IV), pages 2317–2324. IEEE.
Ahmed, A. N., Ravijts, I., de Hoog, J., Anwar, A., Mer-
celis, S., and Hellinckx, P. (2022). A joint perception
scheme for connected vehicles. In 2022 IEEE Sensors,
pages 1–4. IEEE.
Ball
´
e, J., Minnen, D., Singh, S., Hwang, S. J., and Johnston,
N. (2018). Variational image compression with a scale
hyperprior. arXiv preprint arXiv:1802.01436.
Chen, H., Wang, H., Liu, Z., Gu, D., and Ye, W.
(2024). Hp3d-v2v: High-precision 3d object detection
vehicle-to-vehicle cooperative perception algorithm.
Sensors, 24(7):2170.
Chen, Q., Ma, X., Tang, S., Guo, J., Yang, Q., and Fu,
S. (2019). F-cooper: Feature based cooperative per-
ception for autonomous vehicle edge computing sys-
tem using 3d point clouds. In Proceedings of the 4th
ACM/IEEE Symposium on Edge Computing, pages
88–100.
Dosovitskiy, A., Ros, G., Codevilla, F., Lopez, A., and
Koltun, V. (2017). CARLA: An open urban driving
simulator. In Proceedings of the 1st Annual Confer-
ence on Robot Learning, pages 1–16.
Guo, M.-H., Xu, T.-X., Liu, J.-J., Liu, Z.-N., Jiang, P.-T.,
Mu, T.-J., Zhang, S.-H., Martin, R. R., Cheng, M.-M.,
and Hu, S.-M. (2022). Attention mechanisms in com-
puter vision: A survey. Computational visual media,
8(3):331–368.
Han, Y., Zhang, H., Li, H., Jin, Y., Lang, C., and Li, Y.
(2023). Collaborative perception in autonomous driv-
ing: Methods, datasets, and challenges. IEEE Intelli-
gent Transportation Systems Magazine.
Hu, Y., Fang, S., Lei, Z., Zhong, Y., and Chen, S. (2022).
Where2comm: Communication-efficient collabora-
tive perception via spatial confidence maps. Advances
in neural information processing systems, 35:4874–
4886.
Jaderberg, M., Simonyan, K., Zisserman, A., et al. (2015).
Spatial transformer networks. Advances in neural in-
formation processing systems, 28.
Kenney, J. B. (2011). Dedicated short-range communica-
tions (dsrc) standards in the united states. Proceedings
of the IEEE, 99(7):1162–1182.
Krajzewicz, D., Erdmann, J., Behrisch, M., and Bieker,
L. (2012). Recent development and applications of
sumo-simulation of urban mobility. International
journal on advances in systems and measurements,
5(3&4).
Li, Y., Ma, D., An, Z., Wang, Z., Zhong, Y., Chen, S.,
and Feng, C. (2022). V2x-sim: Multi-agent col-
laborative perception dataset and benchmark for au-
tonomous driving. IEEE Robotics and Automation
Letters, 7(4):10914–10921.
VISAPP 2025 - 20th International Conference on Computer Vision Theory and Applications
828