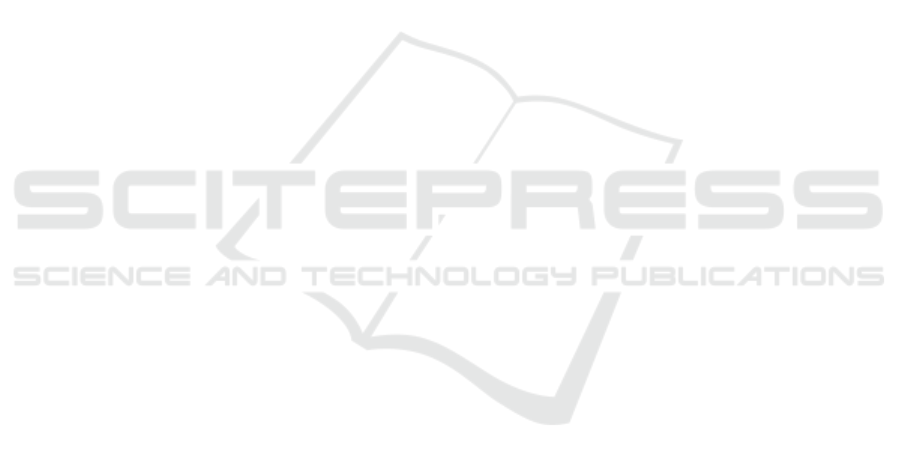
6 CONCLUSION
Recently, gamification has gained widespread atten-
tion across various fields, particularly in education, as
a means to boost user engagement and enhance learn-
ing outcomes. Designers employ a variety of gami-
fication mechanics, such as streaks, progress points,
and time limits, to motivate users to interact with the
game. However, the effectiveness of these mechan-
ics for individuals with different personality traits and
emotional states remains uncertain. To address this
gap, we introduce Gamify, an empirical study with
65 participants that explores how different gamifica-
tion mechanics—specifically rewarding and penaliz-
ing—interact with user profiles, including personal-
ity (based on the Big Five model), emotional state,
age group, field of study, and gender, influence user
experience. For this study, we developed a trivia
game where users solve riddles by choosing from four
possible answers. Our findings reveal that user ex-
perience with specific gamification mechanics vary
based on their profiles. For example, participants un-
der 18 showed enjoyed the experience when both re-
warding and penalizing mechanics were used. Older
age groups 25-34 and 35-44 showed negative over-
all experience when being exposed to a single gami-
fication mechanic, meanwhile male users enjoyed the
task performed in-game less when being penalized for
their incorrect choices. Using Gamify, we extended
tailored gamification literature by drawing findings on
the negative effects of gamification mechanics along
with positive ones (see literature review (Klock et al.,
2020)). Insights drawn from Gamify can help game
developers create personalized experiences that en-
gage users with different needs and characteristics
more effectively.
REFERENCES
Bolock, A. E., Kady, A. E., Herbert, C., and Abdennadher,
S. (2020). Towards a character-based meta recom-
mender for movies. In Alfred, R., Lim, Y., Havilud-
din, H., and On, C. K., editors, Computational Science
and Technology, pages 627–638, Singapore. Springer
Singapore.
Bradley, M. M. and Lang, P. J. (1994). Measuring emotion:
The self-assessment manikin and the semantic differ-
ential. In Journal of Behavior Therapy and Experi-
mental Psychiatry, volume 25, pages 49–59. Elsevier.
Carneiro, N., Miranda, D., Pereira, G., Mendonc¸a, G.,
and Darin, T. (2022). A systematic mapping on
player’s profiles: motivations, behavior, and person-
ality characteristics. Journal on Interactive Systems,
13(1):257–273.
El Bolock, A. (2020). What is character computing?
Springer.
Fischer, F. and Fischer, M. (2024). Personalized gamifica-
tion to promote user engagement: A case study. In
INTED2024 Proceedings, pages 3330–3340. IATED.
Hallifax, S., Lavou
´
e, E., and Serna, A. (2020). To Tailor or
Not to Tailor Gamification? An Analysis of the Impact
of Tailored Game Elements on Learners’ Behaviours
and Motivation, pages 216–227.
Hallifax, S., Serna, A., Marty, J.-C., Lavou
´
e, G., and
Lavou
´
e, E. (2019). Factors to consider for tailored
gamification. In Proceedings of the annual sympo-
sium on computer-human interaction in play, pages
559–572.
Hinderks, A., Schrepp, M., and Thomaschewski, J. (2018).
A benchmark for the short version of the user experi-
ence questionnaire. In WEBIST, pages 373–377.
Huang, Y.-M., Silitonga, L. M., Murti, A. T., and Wu, T.-T.
(2023). Learner engagement in a business simulation
game: Impact on higher-order thinking skills. Journal
of Educational Computing Research, 61(1):96–126.
John, O. P., Srivastava, S., et al. (1999). The big-five trait
taxonomy: History, measurement, and theoretical per-
spectives.
Khaleghi, A., Aghaei, Z., and Mahdavi, M. A. (2021). A
gamification framework for cognitive assessment and
cognitive training: Qualitative study. JMIR Serious
Games, 9(2):e21900.
Khodabandelou, R., Roghanian, P., Gheysari, H., and
Amoozegar, A. (2023). A systematic review of gami-
fication in organizational learning. The Learning Or-
ganization, 30(2):251–272.
Klock, A. C. T., Gasparini, I., Pimenta, M. S., and Hamari,
J. (2020). Tailored gamification: A review of litera-
ture. International Journal of Human-Computer Stud-
ies, 144:102495.
Lopez, C. E. and Tucker, C. S. (2021). Adaptive gam-
ification and its impact on performance. In Inter-
national conference on human-computer interaction,
pages 327–341. Springer.
McAdams, D. P. and Pals, J. L. (2006). A new big five: fun-
damental principles for an integrative science of per-
sonality. American psychologist, 61(3):204.
Mount, M. K. and Barrick, M. R. (1998). Five reasons why
the “big five” article has been frequently cited: The
big five personality dimensions and job performance:
A meta-analysis. Personnel psychology, 51(4):849–
857.
Ortiz-Rojas, M., Chiluiza, K., Valcke, M., and Bolanos-
Mendoza, C. (2025). How gamification boosts learn-
ing in stem higher education: a mixed methods study.
International Journal of STEM Education, 12(1):1.
Pessoa, M., Lima, M., Pires, F., Haydar, G., Melo, R.,
Rodrigues, L., Oliveira, D., Oliveira, E., Galv
˜
ao, L.,
Gadelha, B., et al. (2023). A journey to identify users’
classification strategies to customize game-based and
gamified learning environments. IEEE Transactions
on Learning Technologies.
Rodrigues, L., Palomino, P. T., Toda, A. M., Klock, A. C.,
Oliveira, W., Avila-Santos, A. P., Gasparini, I., and
Gamify: Towards Tailored Gamification Informed by Users’ Personality, Emotional State, and Demographics
743