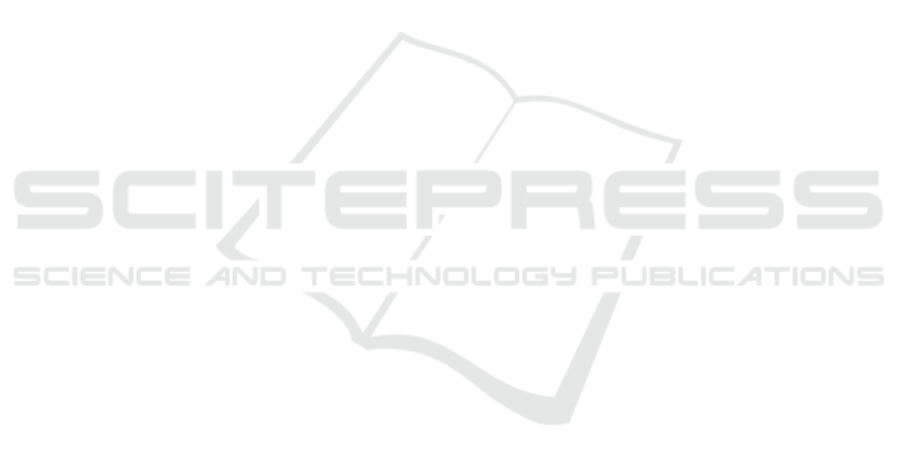
7 CONCLUSIONS AND FUTURE
WORK
This paper presents the motivation and methodology
for the initial version of a technology designed to ad-
dress the gaps identified in a SMS (De Souza et al.,
2024; Mariano et al., 2024). The SMS identifies a lack
of evaluation techniques for text-based chatbot, par-
ticularly to assess aspects of hedonic UX and qualita-
tive data collection. Based on these findings, we de-
veloped GAHAC, a technology that includes 75 qual-
itative guidelines covering 31 aspects of hedonic UX.
In a preliminary evaluation, two experts analyzed
GAHAC and provided key insights. They empha-
sized the importance of analyzing “hallucinations” in
chatbots, a phenomenon involving fabricated or incor-
rect responses that can impact trust and user experi-
ence. E1 emphasized the importance of addressing
this issue in future versions of GAHAC to increase
the trustworthiness of chatbot technologies. In addi-
tion, the experts recommended refining the language
of the guidelines to ensure clarity for evaluators. E2
specifically suggested tailoring the language to eval-
uators rather than end users. Cultural considerations
were also highlighted as crucial for broad applicabil-
ity; E2 highlighted the importance of avoiding region-
alisms and adapting the guidelines to diverse cultural
contexts. Finally, they emphasized the need to verify
that all relevant hedonic aspects are included, with E2
asking for clarification on whether the GAHAC was
designed for specific application contexts and ensur-
ing comprehensive coverage of hedonic dimensions.
The research team will conduct two additional
studies with GAHAC. In the first study, we will
work with HCI and chatbot experts to evaluate GA-
HAC. Experts will participate in an initial orientation
meeting with the researcher. After reviewing GA-
HAC, they will join a follow-up meeting for a semi-
structured interview to discuss their perceptions of the
guidelines. The team will use the findings from this
evaluation to create a revised version of GAHAC.
The second study will test GAHAC’s feasibil-
ity. The researchers will divide a graduate class into
two groups: one group will use GAHAC to iden-
tify hedonic UX issues, while the other will use the
U2CHATBOT (Mafra et al., 2024) checklist for the
same purpose. Both groups will use the ChatGPT
application, following specific instructions to iden-
tify hedonic aspects of UX issues and record their
findings in a spreadsheet. Afterward, participants
will complete a post-assessment questionnaire based
on TAM (Technology Acceptance Model) (Venkatesh
and Bala, 2008), covering Ease of Use, Perceived
Usefulness, and Intention to Use in the Future. The
questionnaire also includes open-ended questions for
detailed feedback and improvement suggestions
We also intend to address the limitations of this
study by conducting a correlation analysis between
the aspects to gain deeper insights into their interde-
pendencies. Additionally, we aim to further explore
the overlaps identified among certain aspects, which
may share attributes or evaluation criteria.
ACKNOWLEDGEMENTS
This research was funded Coordination for the Im-
provement of Higher Education Personnel (CAPES)
- Program of Academic Excellence (PROEX).
REFERENCES
Adamopoulou, E. and Moussiades, L. (2020). An overview
of chatbot technology. In Maglogiannis, I., Iliadis,
L., and Pimenidis, E., editors, Artificial Intelligence
Applications and Innovations, pages 373–383, Cham.
Springer International Publishing.
Al-Amin, M., Ali, M. S., Salam, A., Khan, A., Ali, A., Ul-
lah, A., Alam, M. N., and Chowdhury, S. K. (2024).
History of generative artificial intelligence (ai) chat-
bots: past, present, and future development. arXiv.
Submitted on 4 Feb 2024.
Barbosa, S. D. J., da Silva, B. S., Silveira, M. S., Gasparini,
I., Darin, T., and Barbosa, G. D. J. (2021). Interac¸
˜
ao
Humano-Computador e Experi
ˆ
encia do Usu
´
ario. El-
sevier.
Brown, T. B., Mann, B., Ryder, N., Subbiah, M., Kaplan, J.,
Dhariwal, P., Neelakantan, A., Shyam, P., Sastry, G.,
Askell, A., Agarwal, S., Herbert-Voss, A., Krueger,
G., Henighan, T., Child, R., Ramesh, A., Ziegler,
D. M., Wu, J., Winter, C., Hesse, C., Chen, M., Sigler,
E., Litwin, M., Gray, S., Chess, B., Clark, J., Berner,
C., McCandlish, S., Radford, A., Sutskever, I., and
Amodei, D. (2020). Language models are few-shot
learners. arXiv preprint arXiv:2005.14165.
Candello, H. and Pinhanez, C. (2016). Designing conver-
sational interfaces. Simp
´
osio Brasileiro sobre Fatores
Humanos em Sistemas Computacionais.
Chitturi, R., Raghunathan, R., and Mahajan, V. (2008). De-
light by design: The role of hedonic versus utilitarian
benefits. Journal of marketing, 72(3):48–63.
De Souza, A. C. R., Mariano, P. A. D. L., Guerino, G. C.,
Chaves, A. P., and Valentim, N. M. C. (2024). Tech-
nologies for hedonic aspects evaluation in text-based
chatbots: A systematic mapping study. In Proceed-
ings of the XXII Brazilian Symposium on Human Fac-
tors in Computing Systems, IHC ’23, New York, NY,
USA. Association for Computing Machinery.
Demand Sage (2024). ChatGPT Statistics (November 2024)
– 200 Million Active Users. Accessed: 18 November
2024.
ICEIS 2025 - 27th International Conference on Enterprise Information Systems
550