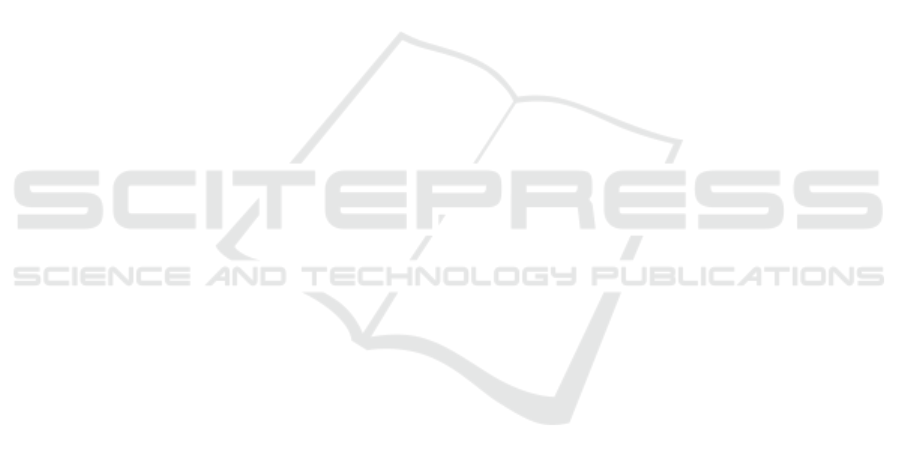
Datta, P., Kaur, A., Sassi, N., Gulzar, Y., and Jaziri, W.
(2024). An evaluation of intelligent and immersive
digital applications in eliciting cognitive states in hu-
mans through the utilization of emotiv insight. Meth-
odsX, 12:102748.
Duricica, T., M
¨
ullnera, P., Weidingera, N., ElSayeda, N.,
Kowalda, D., and Veasa, E. (2024). Ai-powered im-
mersive assistance for interactive task execution in in-
dustrial environments. environment, 28:2.
Dyulicheva, Y. Y. and Glazieva, A. O. (2021). Game
based learning with artificial intelligence and immer-
sive technologies: An overview. CS&SE@ SW, pages
146–159.
Garramone, M., Tonelli, E., Scaioni, M., et al. (2022). A
multi-scale bim/gis framework for railways asset man-
agement. International Archives of the Photogramme-
try, Remote Sensing and Spatial Information Sciences,
46(W1):95–102.
Garz
´
on, J. (2021). An overview of twenty-five years of aug-
mented reality in education. Multimodal Technologies
and Interaction, 5(7):37.
Han, X., Zhang, Z., Ding, N., Gu, Y., Liu, X., Huo, Y., Qiu,
J., Yao, Y., Zhang, A., Zhang, L., et al. (2021). Pre-
trained models: Past, present and future. AI Open,
2:225–250.
Hussein, M. A. H. and Savas¸, S. (2024). Lstm-based text
generation: A study on historical datasets. arXiv
preprint arXiv:2403.07087.
Ibne Akhtar, N., Mohimenul Islam Shazol, K., Rahman, R.,
and Abu Yousuf, M. (2021). Bangla text generation
using bidirectional optimized gated recurrent unit net-
work. In Proceedings of International Conference on
Trends in Computational and Cognitive Engineering:
Proceedings of TCCE 2020, pages 103–112. Springer.
Karaaslan, E., Bagci, U., and Catbas, F. N. (2019). Ar-
tificial intelligence assisted infrastructure assessment
using mixed reality systems. Transportation Research
Record, 2673(12):413–424.
Koumou, K. O. and Isafiade, O. (2024). Asset management
trends in diverse settings involving immersive tech-
nology: A systematic literature review. IEEE Access,
12:141785–141813.
Koumou, K. O., Isafiade, O., Kotze, R. C., and Ekpo,
O. E. (2023). Fostering research asset management
and collaboration using publish-subscribe and im-
mersive technologies. In Southern Africa Telecom-
munication Networks and Applications Conference
(SATNAC) 2023, pages 119–124.
Li, L. and Zhang, T. (2021). Research on text generation
based on lstm. International Core Journal of Engi-
neering, 7(5):525–535.
Limna, P., Jakwatanatham, S., Siripipattanakul, S., Kaew-
puang, P., and Sriboonruang, P. (2022). A review of
artificial intelligence (ai) in education during the digi-
tal era. Advance Knowledge for Executives, 1(1):1–9.
Linowes, J. and Babilinski, K. (2017). Augmented real-
ity for developers: Build practical augmented reality
applications with unity, ARCore, ARKit, and Vuforia.
Packt Publishing Ltd. ISBN-13: 978-1787286436.
Lipton, Z. C. (2015). A critical review of recurrent neu-
ral networks for sequence learning. arXiv Preprint,
CoRR, abs/1506.00019, pages 1–38.
Ma, Y., Hu, Z., Liu, Y., Xie, T., and Liu, X. (2018). Aladdin:
Automating release of deep-link apis on android. In
Proceedings of the 2018 World Wide Web Conference,
pages 1469–1478.
Manith, E., Park, C., and Yoo, K.-H. (2019). A hierar-
chical structure for representing 3d respiration organ
models. In Big Data Applications and Services 2017:
The 4th International Conference on Big Data Appli-
cations and Services 4, pages 23–36. Springer.
¨
Ozg
¨
ur, A. and Nar, F. (2020). Effect of dropout layer on
classical regression problems. In 2020 28th Signal
Processing and Communications Applications Con-
ference (SIU), pages 1–4. IEEE.
Ozturkcan, S. (2021). Service innovation: Using augmented
reality in the ikea place app. Journal of Information
Technology Teaching Cases, 11(1):8–13.
Rampini, L., Cecconi, F. R., et al. (2022). Artificial intel-
ligence in construction asset management: A review
of present status, challenges and future opportunities.
Journal of Information Technology in Construction,
27:884–913.
Ren, J., Xia, F., Chen, X., Liu, J., Hou, M., Shehzad, A.,
Sultanova, N., and Kong, X. (2021). Matching al-
gorithms: Fundamentals, applications and challenges.
IEEE Transactions on Emerging Topics in Computa-
tional Intelligence, 5(3):332–350.
Sepasgozar, S. M. (2020). Digital twin and web-based vir-
tual gaming technologies for online education: A case
of construction management and engineering. Applied
Sciences, 10(13):4678.
Suh, A. and Prophet, J. (2018). The state of immersive tech-
nology research: A literature analysis. Computers in
Human behavior, 86:77–90.
Wang, X., Love, P. E., Kim, M. J., Park, C.-S., Sing, C.-
P., and Hou, L. (2013). A conceptual framework for
integrating building information modeling with aug-
mented reality. Automation in construction, 34:37–44.
Zhang, C. and Lu, Y. (2021). Study on artificial intelligence:
The state of the art and future prospects. Journal of
Industrial Information Integration, 23:100224.
Dynamic Integration of 3D Augmented Reality Features with AI-Based Contextual and Personalized Overlays in Asset Management
435