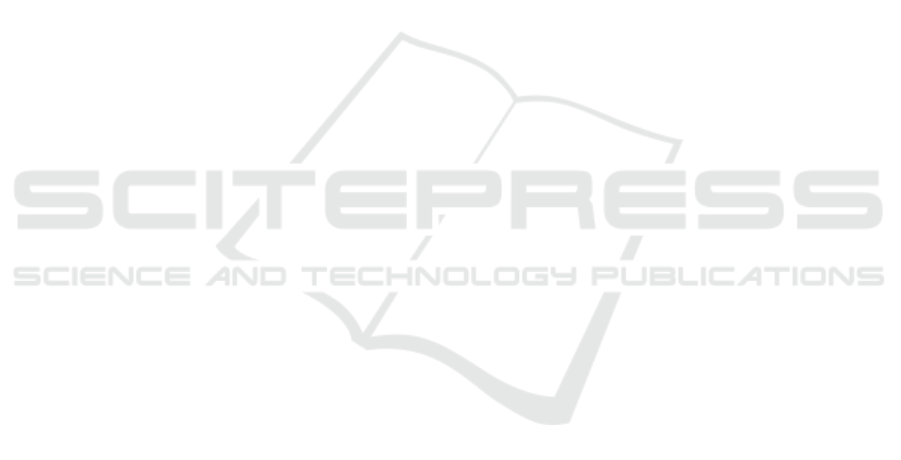
I., and Amodei, D. (2020). Language models are few-
shot learners. In Proceedings of the 34th Interna-
tional Conference on Neural Information Processing
Systems, NIPS ’20, Red Hook, NY, USA. Curran As-
sociates Inc.
Chapman, P. J., Rubio-Gonz
´
alez, C., and Thakur, A. V.
(2024). Interleaving static analysis and llm prompt-
ing. In Proceedings of the 13th ACM SIGPLAN In-
ternational Workshop on the State Of the Art in Pro-
gram Analysis, SOAP 2024, page 9–17, New York,
NY, USA. Association for Computing Machinery.
Chiang, C.-H., Chen, W.-C., Kuan, C.-Y., Yang, C., and
yi Lee, H. (2024). Large language model as an assign-
ment evaluator: Insights, feedback, and challenges in
a 1000+ student course.
Dong, G., Yuan, H., Lu, K., Li, C., Xue, M., Liu, D., Wang,
W., Yuan, Z., Zhou, C., and Zhou, J. (2024). How
abilities in large language models are affected by su-
pervised fine-tuning data composition. In Ku, L.-W.,
Martins, A., and Srikumar, V., editors, Proceedings
of the 62nd Annual Meeting of the Association for
Computational Linguistics (Volume 1: Long Papers),
pages 177–198, Bangkok, Thailand. Association for
Computational Linguistics.
Eniser, H. F., Zhang, H., David, C., Wang, M., Christakis,
M., Paulsen, B., Dodds, J., and Kroening, D. (2024).
Towards translating real-world code with llms: A
study of translating to rust.
Henkel, O., Hills, L., Boxer, A., Roberts, B., and Levo-
nian, Z. (2024). Can large language models make
the grade? an empirical study evaluating llms abil-
ity to mark short answer questions in k-12 education.
In Proceedings of the Eleventh ACM Conference on
Learning @ Scale, L@S ’24, page 300–304, New
York, NY, USA. Association for Computing Machin-
ery.
Huang, L., Zhao, H., Yang, K., Liu, Y., and Xiao, Z. (2018).
Learning outcomes-oriented feedback-response peda-
gogy in computer system course. In 2018 13th Inter-
national Conference on Computer Science & Educa-
tion (ICCSE), pages 1–4.
Hundhausen, C. D., Agrawal, A., and Agarwal, P. (2013).
Talking about code: Integrating pedagogical code re-
views into early computing courses. ACM Trans.
Comput. Educ., 13(3).
Jiang, X., Dong, Y., Wang, L., Fang, Z., Shang, Q., Li, G.,
Jin, Z., and Jiao, W. (2024). Self-planning code gener-
ation with large language models. ACM Trans. Softw.
Eng. Methodol., 33(7).
Lewis, P., Perez, E., Piktus, A., Petroni, F., Karpukhin,
V., Goyal, N., K
¨
uttler, H., Lewis, M., Yih,
W.-t., Rockt
¨
aschel, T., Riedel, S., and Kiela,
D. (2020). Retrieval-augmented generation for
knowledge-intensive nlp tasks. In Proceedings of the
34th International Conference on Neural Information
Processing Systems, NIPS ’20, Red Hook, NY, USA.
Curran Associates Inc.
Liffiton, M., Sheese, B. E., Savelka, J., and Denny, P.
(2024). Codehelp: Using large language models
with guardrails for scalable support in programming
classes. In Proceedings of the 23rd Koli Calling In-
ternational Conference on Computing Education Re-
search, Koli Calling ’23, New York, NY, USA. Asso-
ciation for Computing Machinery.
Liu, C., Hoang, L., Stolman, A., and Wu, B. (2024). Hita: A
rag-based educational platform that centers educators
in the instructional loop. In Olney, A. M., Chounta,
I.-A., Liu, Z., Santos, O. C., and Bittencourt, I. I., ed-
itors, Artificial Intelligence in Education, pages 405–
412, Cham. Springer Nature Switzerland.
Mahbub, T., Dghaym, D., Shankarnarayanan, A., Syed, T.,
Shapsough, S., and Zualkernan, I. (2024). Can gpt-4
aid in detecting ambiguities, inconsistencies, and in-
completeness in requirements analysis? a comprehen-
sive case study. IEEE Access, pages 1–1.
Meta (2024). https://ai.meta.com/blog/meta-llama-3/ - last
access 28th october 2024.
OpenAI (2022). https://platform.openai.com/docs/models/
gpt-3-5-turbo - last access 28th october 2024.
OpenAI (2024). https://platform.openai.com/docs/models/
gpt-4o - last access 28th october 2024.
Piscitelli, A., Costagliola, G., De Rosa, M., and Fuccella,
V. (2024). Influence of large language models on pro-
gramming assignments – a user study. In ICETC 2024.
ACM.
Prather, J., Reeves, B. N., Denny, P., Becker, B. A.,
Leinonen, J., Luxton-Reilly, A., Powell, G., Finnie-
Ansley, J., and Santos, E. A. (2023). “it’s weird that
it knows what i want”: Usability and interactions with
copilot for novice programmers. 31(1). Place: New
York, NY, USA Publisher: Association for Comput-
ing Machinery.
Qi, J. Z.-P. L., Hartmann, B., and Norouzi, J. D. N. (2023).
Conversational programming with llm-powered inter-
active support in an introductory computer science
course.
Tabari, P., Piscitelli, A., Costagliola, G., and Rosa, M. D.
(2025). Assessing the potential of an llm-powered sys-
tem for enhancing fhir resource validation. In Studies
in Health Technology and Informatics.
Wang, L., Xu, W., Lan, Y., Hu, Z., Lan, Y., Lee, R. K.-W.,
and Lim, E.-P. (2023). Plan-and-solve prompting: Im-
proving zero-shot chain-of-thought reasoning by large
language models. In Rogers, A., Boyd-Graber, J., and
Okazaki, N., editors, Proceedings of the 61st Annual
Meeting of the Association for Computational Lin-
guistics (Volume 1: Long Papers), pages 2609–2634,
Toronto, Canada. Association for Computational Lin-
guistics.
Wei, J., Wang, X., Schuurmans, D., Bosma, M., Ichter, B.,
Xia, F., Chi, E. H., Le, Q. V., and Zhou, D. (2024).
Chain-of-thought prompting elicits reasoning in large
language models. In Proceedings of the 36th Interna-
tional Conference on Neural Information Processing
Systems, NIPS ’22, Red Hook, NY, USA. Curran As-
sociates Inc.
CSEDU 2025 - 17th International Conference on Computer Supported Education
544