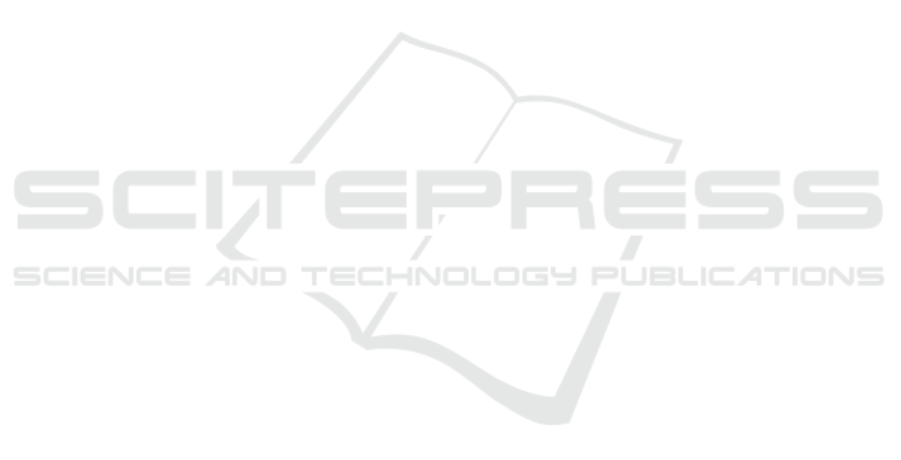
monitoring of complex system requirements for au-
tonomous driving functions.
Hampel, F. O., Morast, A., Nießen, N., and Schindler, C.
(2021). Automatischer Eisenbahnverkehr: Aufgaben
des Triebfahrzeugf
¨
uhrers am Beispiel der Strecken-
beobachtung. In Proceedings of the 3rd International
Railway Symposium Aachen, page 367.
Haxthausen, A. E. and Fantechi, A. (2023). Compositional
verification of railway interlocking systems. Formal
Aspects of Computing, 35(1):1–46.
Hayward, J. C. (1972). Near-miss determination through
use of a scale of danger. Highway Research Record.
HENSOLDT (2023). Simsphere train. Accessed
April 11, 2023. https://www.hensoldt.net/stories/
training-train-drivers-etcs-app-by-hensoldt/.
H
¨
olscher, C. (2023). Zusi bahnsimulatoren. Accessed April
11, 2023. https://www.zusi.de.
Issad, M., Kloul, L., and Rauzy, A. (2018). Scenario-
oriented reverse engineering of complex railway sys-
tem specifications. 21(2):91–104.
Jansson, J. (2005). Collision avoidance theory: With appli-
cation to automotive collision mitigation. PhD Thesis,
Link
¨
oping University.
Kalisvaart, S., Slavik, Z., and Op den Camp, O. (2020). Us-
ing scenarios in safety validation of automated sys-
tems. In Validation and Verification of Automated Sys-
tems. Springer.
Kearney, J., Willemsen, P., Donikian, S., and Devillers, F.
(1999). Scenario languages for driving simulation. In
Driving Simulation Conference, DSC’99.
Klamann, B., Lippert, M., Amersbach, C., and Winner, H.
(2019). Defining Pass-/Fail-Criteria for Particular
Tests of Automated Driving Functions. Pages: 174.
Koopman, P. and Wagner, M. (2018). Toward a framework
for highly automated vehicle safety validation. In
WCX World Congress Experience. SAE International.
Kramer, B., Neurohr, C., B
¨
uker, M., B
¨
ode, E., Fr
¨
anzle, M.,
and Damm, W. (2020). Identification and quantifica-
tion of hazardous scenarios for automated driving. In
International symposium on model-based safety and
assessment, pages 163–178. Springer.
Krichen, M., Mihoub, A., Alzahrani, M. Y., Adoni, W.
Y. H., and Nahhal, T. (2022). Are formal methods
applicable to machine learning and artificial intelli-
gence? In 2nd International Conference of Smart Sys-
tems and Emerging Technologies (SMARTTECH).
Leitner, A. (2020). Enable-s3: Project introduction. In
Validation and Verification of Automated Systems.
Springer.
Menzel, T., Bagschik, G., and Maurer, M. (2018). Scenarios
for development, test and validation of automated ve-
hicles. In 2018 IEEE Intelligent Vehicles Symposium
(IV), pages 1821–1827. IEEE.
M
¨
uller Systemtechnik (2023). Mst trieb-
fahrzeug simulator. Accessed April 11,
2023. https://muellersystemtechnik.de/
mst-triebfahrzeug-simulator.
Neurohr, C., Westhofen, L., Butz, M., Bollmann, M. H.,
Eberle, U., and Galbas, R. (2021). Criticality analysis
for the verification and validation of automated vehi-
cles. 9:18016–18041.
Neurohr, C., Westhofen, L., Henning, T., de Graaff, T.,
M
¨
ohlmann, E., and B
¨
ode, E. (2020). Fundamental
considerations around scenario-based testing for au-
tomated driving. In 2020 IEEE Intelligent Vehicles
Symposium (IV), pages 121–127. IEEE.
Rauschert, A. and Amid, G. (2024a). ASAM OpenSCE-
NARIO DSL 2.1.0: Release presentation. Presenta-
tion. Last accessed on 2024-05-08.
Rauschert, A. and Amid, G. (2024b). ASAM OpenSCE-
NARIO XML 1.3.0: Release presentation. Presenta-
tion. Last accessed on 2024-05-08.
Riedmaier, S., Ponn, T., Ludwig, D., Schick, B., and Dier-
meyer, F. (2020). Survey on scenario-based safety as-
sessment of automated vehicles. 8:87456–87477.
Schwenzer, M., Ay, M., Bergs, T., and Abel, D. (2021).
Review on model predictive control: An engineering
perspective. The International Journal of Advanced
Manufacturing Technology, 117(5):1327–1349.
Sch
¨
afer, S., Greiner-Fuchs, L., Hofmeier, T., Koch, P., and
Cichon, M. (2023). Virtual validation method of au-
tomated on-sight driving systems for shunting opera-
tions.
Sobieszczanski-Sobieski, J. (1990). Sensitivity of complex,
internally coupled systems. AIAA journal, 28(1):153–
160.
Tagiew, R., Leinhos, D., von der Haar, H., Klotz, C., Sprute,
D., Ziehn, J., Schmelter, A., Witte, S., and Klasek, P.
(2022). Onboard sensor systems for automatic train
operation. In Dependable Computing – EDCC 2022
Workshops, volume 1656 of Communications in Com-
puter and Information Science. Springer.
Virtanen, P., Gommers, R., Oliphant, T. E., Haberland, M.,
Reddy, T., Cournapeau, D., Burovski, E., Peterson,
P., Weckesser, W., Bright, J., et al. (2020). Scipy
1.0: fundamental algorithms for scientific computing
in python. Nature methods, 17(3):261–272.
Westhofen, L., Neurohr, C., Koopmann, T., Butz, M.,
Sch
¨
utt, B., Utesch, F., Neurohr, B., Gutenkunst, C.,
and B
¨
ode, E. (2022). Criticality metrics for automated
driving: A review and suitability analysis of the state
of the art.
Wild, M., Becker, J. S., Ehmen, G., and M
¨
ohlmann, E.
(2023). Towards scenario-based certification of highly
automated railway systems. In Reliability, Safety, and
Security of Railway Systems, LNCS. Springer.
Yusuf, M., MacDonald, A., Stuart, R., and Miyazaki, H.
(2020). Heavy haul freight transportation system: Au-
tohaul. Technical report.
A Scenario-Based Simulation Framework for Testing of Highly Automated Railway Systems
99