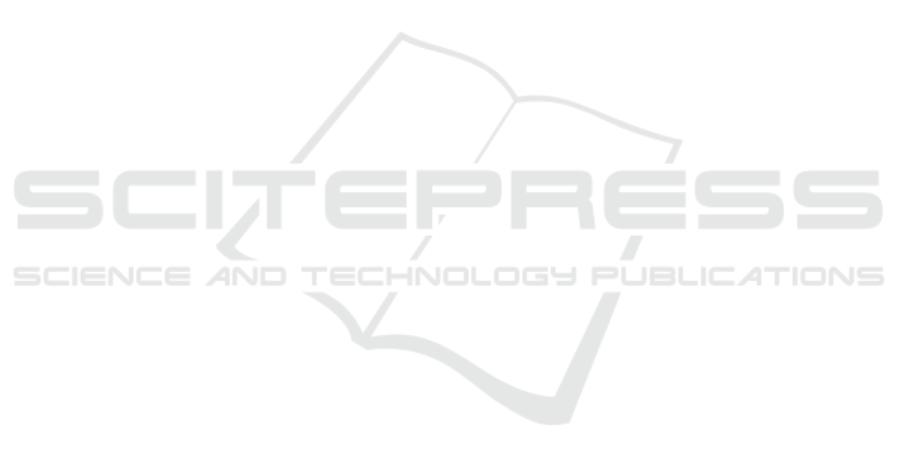
latter can be more competitive with the no augmenta-
tion implementation.
In essence, the potential of GANs to compensate
for dataset difficulties, including or not XAI methods,
is not very present here, and this can be for a variety
of reasons, including the necessity of more intensive
training in the generating phase. Nevertheless, this
work is significant for the fractal features and histo-
logical images classification research, as it presents
the results performance of using handcrafted features
in histological images. These findings provide guide-
lines for researchers and experts interested in develop-
ing artificial augmentation techniques for histopatho-
logical datasets, and what to expect on the perfor-
mance of these methods. The association of hand-
crafted features and data augmentation in histological
images does not present itself in these cases, but since
it should improve the results, there are a few lines to
go from here.
In future work, we should evaluate different GAN
training methods combined with handcrafted features.
There’s potential for augmentation to yield better
results with more intensive training, compared to
transformer and convolutional counterparts (Rozendo
et al., 2024). While this might seem like a draw-
back, the time invested in fractal geometry analysis —
which can be significantly shorter than more complex
implementations in some cases — offsets this con-
cern. Furthermore, the potential for combining frac-
tal geometry analysis with other advanced machine
learning techniques could lead to more robust and ac-
curate classification models for histological images.
As the field continues to evolve, it will be crucial to
explore these hybrid approaches to maximize the ben-
efits of both handcrafted features and new techniques.
ACKNOWLEDGEMENTS
This research was funded in part by the Coordenac¸
˜
ao
de Aperfeic¸oamento de Pessoal de N
´
ıvel Supe-
rior—Brasil (CAPES)—Finance Code 001; National
Council for Scientific and Technological Develop-
ment CNPq (Grants #132940/2019-1, #313643/2021-
0 and #311404/2021-9); the State of Minas Gerais Re-
search Foundation—FAPEMIG (Grant #APQ-00578-
18); S
˜
ao Paulo Research Foundation—FAPESP
(Grant #2022/03020-1).
REFERENCES
Alajaji, S. A., Khoury, Z. H., Elgharib, M., Saeed, M.,
Ahmed, A. R., Khan, M. B., Tavares, T., Jessri, M.,
Puche, A. C., Hoorfar, H., et al. (2023). Generative
adversarial networks in digital histopathology: current
applications, limitations, ethical considerations, and
future directions. Modern Pathology, page 100369.
Battalapalli, D., Vidyadharan, S., Prabhakar Rao, B., Yo-
geeswari, P., Kesavadas, C., and Rajagopalan, V.
(2023). Fractal dimension: analyzing its potential
as a neuroimaging biomarker for brain tumor diagno-
sis using machine learning. Frontiers in Physiology,
14:1201617.
Brancati, N. and Frucci, M. (2024). Improving breast tumor
multi-classification from high-resolution histological
images with the integration of feature space data aug-
mentation. Information, 15(2):98.
Gulrajani, I., Ahmed, F., Arjovsky, M., Dumoulin, V., and
Courville, A. (2017). Improved training of wasser-
stein gans.
Hechtlinger, Y. (2016). Interpretation of prediction models
using the input gradient.
Ivanovici, M. and Richard, N. (2010). Fractal dimension
of color fractal images. IEEE Transactions on Image
Processing, 20(1):227–235.
Japkowicz, N. and Shah, M. (2011). Evaluating learning
algorithms: a classification perspective. Cambridge
University Press.
Jiang, L., Huang, S., Luo, C., Zhang, J., Chen, W., and Liu,
Z. (2023). An improved multi-scale gradient gener-
ative adversarial network for enhancing classification
of colorectal cancer histological images. Frontiers in
Oncology, 13:1240645.
Jolicoeur-Martineau, A. (2018). The relativistic discrimina-
tor: a key element missing from standard gan.
Roberto, G. F., Lumini, A., Neves, L. A., and do Nasci-
mento, M. Z. (2021). Fractal neural network: A new
ensemble of fractal geometry and convolutional neu-
ral networks for the classification of histology images.
Expert Systems with Applications, 166:114103.
Rozendo, G. B., Garcia, B. L. d. O., Borgue, V. A. T.,
Lumini, A., Tosta, T. A. A., Nascimento, M. Z. d.,
and Neves, L. A. (2024). Data augmentation in
histopathological classification: An analysis explor-
ing gans with xai and vision transformers. Applied
Sciences, 14(18):8125.
Ryspayeva, M. (2023). Generative adversarial network as
data balance and augmentation tool in histopathology
of breast cancer. In 2023 IEEE International Confer-
ence on Smart Information Systems and Technologies
(SIST), pages 99–104. IEEE.
Shrikumar, A., Greenside, P., and Kundaje, A. (2019).
Learning important features through propagating ac-
tivation differences.
Simonyan, K., Vedaldi, A., and Zisserman, A. (2014).
Deep inside convolutional networks: Visualising im-
age classification models and saliency maps.
ICEIS 2025 - 27th International Conference on Enterprise Information Systems
762