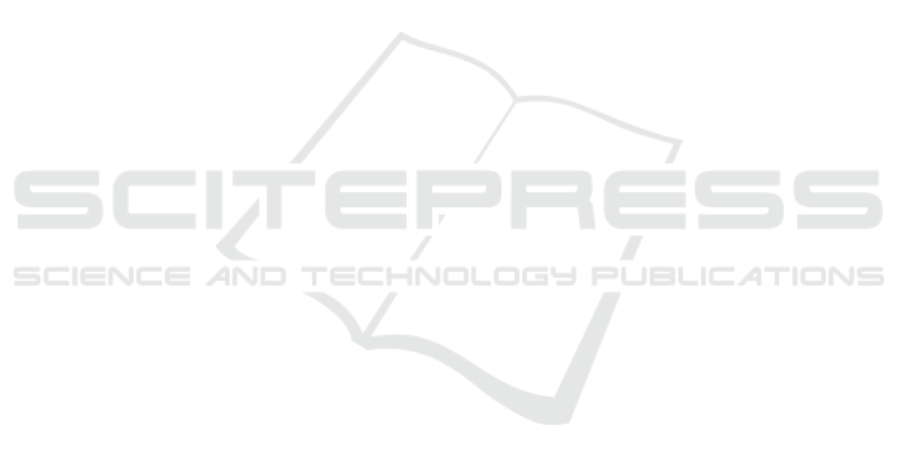
7 CONCLUSION AND FUTURE
WORK
This paper addresses a critical challenge in verifiable
autonomous decision-making: bridging the semantic
gap between program execution and model check-
ing in vGOAL. We have made three key contribu-
tions: (1) identifying and analyzing the semantic gap
in vGOAL, (2) developing an improved interpreter that
aligns the implementation of the next program state,
and (3) demonstrating how stepwise refinement can
effectively address potential efficiency issues.
Our case study of an autonomous logistics sys-
tem with three mobile robots validates our approach.
The results show that while our improved interpreter
may introduce some execution overhead, the step-
wise refinement successfully reduces the execution
time for move actions by 34.6%. Although the re-
finement process increased the state space and model-
checking time, the additional computational cost re-
mained manageable, demonstrating the practicality of
our approach.
Future work will focus on two directions: (1)
extending experimental validation across a broader
range of multi-agent scenarios and real-world envi-
ronments, and (2) conducting comprehensive scal-
ability analysis with increasing system complexity.
These extensions will further validate and enhance
our approach to developing reliable autonomous
decision-making systems.
ACKNOWLEDGEMENTS
This research is partially funded by the Research
Fund KU Leuven.
REFERENCES
Bordini, R. H., Fisher, M., Pardavila, C., and Wooldridge,
M. (2003). Model checking AgentSpeak. In Pro-
ceedings of the second international joint conference
on Autonomous agents and multiagent systems, pages
409–416.
Bordini, R. H. and H
¨
ubner, J. F. (2005). BDI agent program-
ming in AgentSpeak using Jason. In International
workshop on computational logic in multi-agent sys-
tems, pages 143–164. Springer.
Dastani, M., Tinnemeier, N. A., and Meyer, J.-J. C. (2009).
A programming language for normative multi-agent
systems. In Handbook of Research on Multi-Agent
Systems: semantics and dynamics of organizational
models, pages 397–417. IGI Global.
Dennis, L. A. (2018). The mcapl framework including the
agent infrastructure layer and agent java pathfinder.
The Journal of Open Source Software.
Dennis, L. A., Fisher, M., and Webster, M. (2018). Two-
stage agent program verification. Journal of Logic and
Computation, 28(3):499–523.
Dennis, L. A., Fisher, M., Webster, M. P., and Bordini,
R. H. (2012). Model checking agent programming
languages. Automated software engineering, 19(1):5–
63.
Hindriks, K. V. (2009). Programming rational agents in
GOAL. In Multi-agent programming, pages 119–157.
Springer, Berlin, Heidelberg.
Hindriks, K. V., De Boer, F. S., Van der Hoek, W., and
Meyer, J.-J. C. (1999). Agent programming in 3apl.
Autonomous Agents and Multi-Agent Systems, 2:357–
401.
Holzmann, G. J. (1997). The model checker spin. IEEE
Transactions on software engineering, 23(5):279–
295.
Jongmans, S. (2010). Model checking GOAL agents.
Jongmans, S.-S. T., Hindriks, K. V., and Van Riemsdijk,
M. B. (2010). Model checking agent programs by us-
ing the program interpreter. In Computational Logic
in Multi-Agent Systems: 11th International Workshop,
CLIMA XI, Lisbon, Portugal, August 16-17, 2010.
Proceedings 11, pages 219–237. Springer.
Kwiatkowska, M., Norman, G., and Parker, D. (2011).
Prism 4.0: Verification of probabilistic real-time sys-
tems. In Computer Aided Verification: 23rd Interna-
tional Conference, CAV 2011, Snowbird, UT, USA,
July 14-20, 2011. Proceedings 23, pages 585–591.
Springer.
Luckcuck, M., Farrell, M., Dennis, L. A., Dixon, C., and
Fisher, M. (2019). Formal specification and verifica-
tion of autonomous robotic systems: A survey. ACM
Computing Surveys (CSUR), 52(5):1–41.
Winikoff, M. (2007). Implementing commitment-based in-
teractions. In Proceedings of the 6th international
joint conference on Autonomous agents and multia-
gent systems, pages 1–8.
Yang, Y. (2024). Supplementary Documents. https://github.
com/yiyangvGOAL/vGOALICAART2025.git.
Yang, Y. and Holvoet, T. (2023a). vGOAL: a GOAL-based
specification language for safe autonomous decision-
making. In Engineering Multi-Agent Systems: 11th
International Workshop, EMAS 2023, London, UK,
29-30 May 2023, Revised Selected Papers.
Yang, Y. and Holvoet, T. (2023b). Making model checking
feasible for goal. Annals of Mathematics and Artificial
Intelligence.
Yang, Y. and Holvoet, T. (2023c). Safe autonomous
decision-making with vGOAL. In Advances in
Practical Applications of Agents, Multi-Agent Sys-
tems, and Cognitive Mimetics. The PAAMS Collec-
tion. Guimar
˜
aes, Portugal.
Yang, Y. and Holvoet, T. (2024). Model Checking of
vGOAL. arXiv, Preprint. Preprint.
Bridging the Semantic Gap in vGOAL for Verifiable Autonomous Decision-Making
587