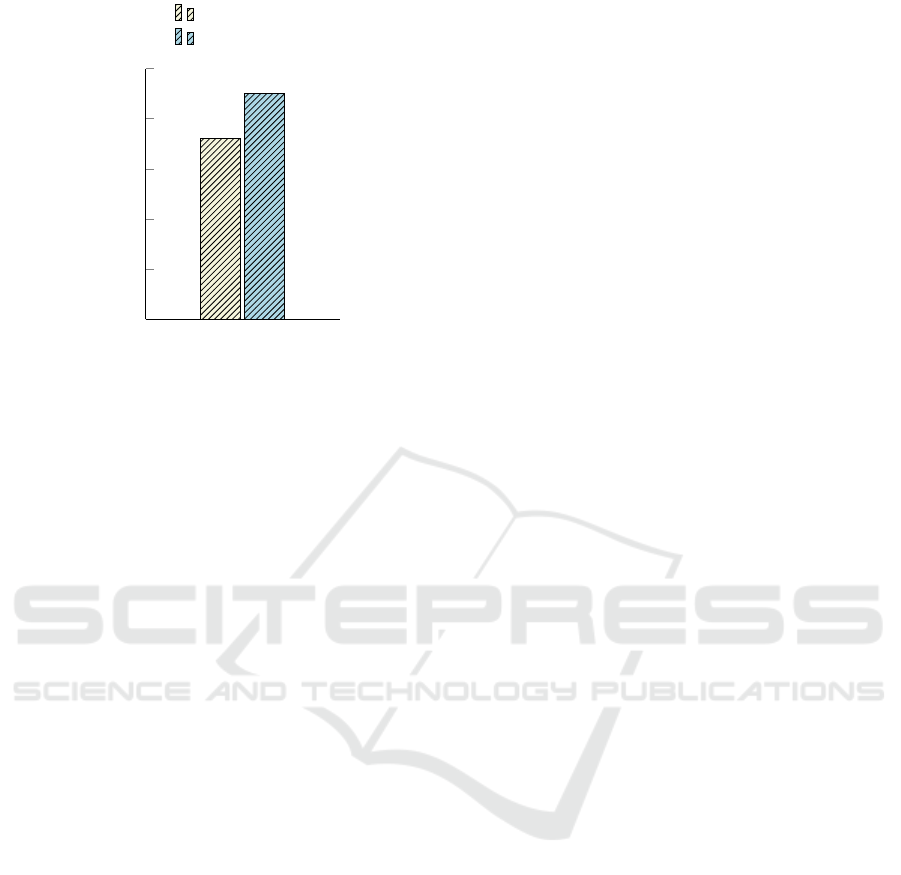
Models
0
10
20
30
40
50
36
45
Number of Correct Outcomes
GPT-3.5
GPT-4o
Figure 8: Comparison result between GPT-3.5 and GPT-4o.
LLM to generate thoughts and decide on actions, thus
integrating external tools to provide useful informa-
tion and compensate for the LLM’s knowledge gaps.
Our method generated 42 correct official organiza-
tions and 36 correct websites of policy evidence us-
ing GPT-3.5 in 50 experiments, significantly outper-
forming the CoT prompting approach, and the effec-
tiveness of the method improved when using a more
advanced LLM as base model. With our method, re-
searchers can efficiently collect evidence to support
policy analysis and make informed policy decisions.
REFERENCES
Brown, T., Mann, B., Ryder, N., Subbiah, M., Kaplan, J. D.,
Dhariwal, P., Neelakantan, A., Shyam, P., and et al.,
S. (2020). Language models are few-shot learners.
In Larochelle, H., Ranzato, M., Hadsell, R., Balcan,
M., and Lin, H., editors, Advances in Neural Infor-
mation Processing Systems, volume 33, pages 1877–
1901. Curran Associates, Inc.
Cobbe, K., Kosaraju, V., Bavarian, M., Chen, M., Jun,
H., Kaiser, L., Plappert, M., Tworek, J., Hilton, J.,
Nakano, R., Hesse, C., and Schulman, J. (2021).
Training verifiers to solve math word problems.
ArXiv, abs/2110.14168.
Devlin, J., Chang, M.-W., Lee, K., and Toutanova, K.
(2019). Bert: Pre-training of deep bidirectional trans-
formers for language understanding. In Burstein, J.,
Doran, C., and Solorio, T., editors, Proceedings of
the 2019 Conference of the North American Chap-
ter of the Association for Computational Linguistics:
Human Language Technologies, Volume 1 (Long and
Short Papers), pages 4171–4186, Minneapolis, Min-
nesota. Association for Computational Linguistics.
Franc¸oise, M., Frambourt, C., Goodwin, P., et al. (2022).
Evidence based policy making during times of uncer-
tainty through the lens of future policy makers: four
recommendations to harmonise and guide health pol-
icy making in the future. Archives of Public Health,
80:140.
Hooper, R., Goyal, N., Blok, K., and Scholten, L. (2023). A
semi-automated approach to policy-relevant evidence
synthesis: Combining natural language processing,
causal mapping, and graph analytics for public policy.
Research Square.
Lee, C., Roy, R., Xu, M., Raiman, J., Shoeybi, M., Catan-
zaro, B., and Ping, W. (2024). Nv-embed: Improved
techniques for training llms as generalist embedding
models. ArXiv, abs/2405.17428.
Lee, K., Ippolito, D., Nystrom, A., Zhang, C., Eck, D.,
Callison-Burch, C., and Carlini, N. (2022). Dedupli-
cating training data makes language models better. In
Muresan, S., Nakov, P., and Villavicencio, A., editors,
Proceedings of the 60th Annual Meeting of the Associ-
ation for Computational Linguistics (Volume 1: Long
Papers), pages 8424–8445, Dublin, Ireland. Associa-
tion for Computational Linguistics.
Lewis, P., Perez, E., Piktus, A., Petroni, F., Karpukhin,
V., Goyal, N., K
¨
uttler, H., Lewis, M., Yih,
W.-t., Rockt
¨
aschel, T., Riedel, S., and Kiela,
D. (2020). Retrieval-augmented generation for
knowledge-intensive nlp tasks. In Larochelle, H.,
Ranzato, M., Hadsell, R., Balcan, M., and Lin, H.,
editors, Advances in Neural Information Processing
Systems, volume 33, pages 9459–9474. Curran Asso-
ciates, Inc.
Lin, S., Hilton, J., and Evans, O. (2022). TruthfulQA:
Measuring how models mimic human falsehoods. In
Muresan, S., Nakov, P., and Villavicencio, A., editors,
Proceedings of the 60th Annual Meeting of the Associ-
ation for Computational Linguistics (Volume 1: Long
Papers), pages 3214–3252, Dublin, Ireland. Associa-
tion for Computational Linguistics.
Ling, W., Yogatama, D., Dyer, C., and Blunsom, P. (2017).
Program induction by rationale generation: Learn-
ing to solve and explain algebraic word problems.
In Barzilay, R. and Kan, M.-Y., editors, Proceedings
of the 55th Annual Meeting of the Association for
Computational Linguistics (Volume 1: Long Papers),
pages 158–167, Vancouver, Canada. Association for
Computational Linguistics.
Narayanan Venkit, P., Gautam, S., Panchanadikar, R.,
Huang, T.-H., and Wilson, S. (2023). Nationality bias
in text generation. In Vlachos, A. and Augenstein, I.,
editors, Proceedings of the 17th Conference of the Eu-
ropean Chapter of the Association for Computational
Linguistics, pages 116–122, Dubrovnik, Croatia. As-
sociation for Computational Linguistics.
Onoe, Y., Zhang, M., Choi, E., and Durrett, G. (2022).
Entity cloze by date: What LMs know about unseen
entities. In Carpuat, M., de Marneffe, M.-C., and
Meza Ruiz, I. V., editors, Findings of the Association
for Computational Linguistics: NAACL 2022, pages
693–702, Seattle, United States. Association for Com-
putational Linguistics.
Ouyang, L., Wu, J., Jiang, X., Almeida, D., Wainwright,
C. L., Mishkin, P., Zhang, C., Agarwal, S., Slama, K.,
Ray, A., Schulman, J., Hilton, J., Kelton, F., Miller,
Application of Large Language Models and ReAct Prompting in Policy Evidence Collection
973