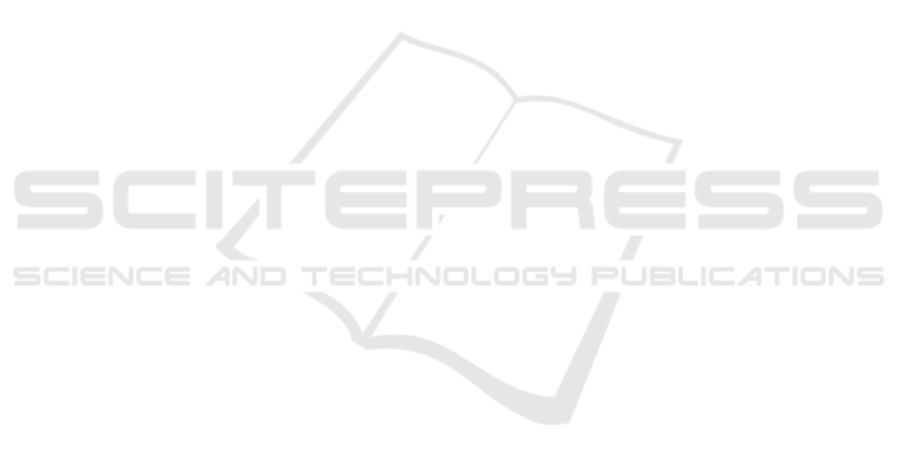
International Conference on Data Engineering Work-
shops (ICDEW), pages 74–78. ISSN: 2473-3490.
Fernandez, R. C., Elmore, A. J., Franklin, M. J., Krishnan,
S., and Tan, C. (2023). How Large Language Models
Will Disrupt Data Management. VLDB, 16(11):3302–
3309.
Halevy, A. and Dwivedi-Yu, J. (2023). Learnings from
Data Integration for Augmented Language Models.
arXiv:2304.04576 [cs].
Huang, L., Yu, W., Ma, W., Zhong, W., Feng, Z., Wang,
H., Chen, Q., Peng, W., Feng, X., Qin, B., and Liu, T.
(2024). A Survey on Hallucination in Large Language
Models: Principles, Taxonomy, Challenges, and Open
Questions. ACM Transactions on Information Sys-
tems, page 3703155.
H
¨
attasch, B., Truong-Ngoc, M., Schmidt, A., and Bin-
nig, C. (2022). It’s AI Match: A Two-Step Ap-
proach for Schema Matching Using Embeddings.
arXiv:2203.04366 [cs].
Loster, M., Koumarelas, I., and Naumann, F. (2021).
Knowledge Transfer for Entity Resolution with
Siamese Neural Networks. Journal of Data and In-
formation Quality, 13(1):1–25.
Mihindukulasooriya, N., Dash, S., and Bagchi, S. (2023).
Unleashing the Potential of Data Lakes with Seman-
tic Enrichment Using Foundation Models. In ISWC
Industry Track CEURWP, Athens, Greece.
Narayan, A., Chami, I., Orr, L., Arora, S., and R
´
e, C.
(2022). Can Foundation Models Wrangle Your Data?
arXiv:2205.09911 [cs].
Papadakis, G., Skoutas, D., Thanos, E., and Palpanas, T.
(2020). Blocking and Filtering Techniques for En-
tity Resolution: A Survey. ACM Comput. Surv.,
53(2):31:1–31:42.
Paulsen, D., Govind, Y., and Doan, A. (2023). Sparkly:
A Simple yet Surprisingly Strong TF/IDF Blocker for
Entity Matching. VLDB, 16(6):1507–1519.
Peeters, R. and Bizer, C. (2023). Using ChatGPT for Entity
Matching. arXiv:2305.03423 [cs].
Reimers, N. and Gurevych, I. (2019). Sentence-bert: Sen-
tence embeddings using siamese bert-networks. In
Proceedings of the 2019 Conference on Empirical
Methods in Natural Language Processing. Associa-
tion for Computational Linguistics.
Remadi, A., El Hage, K., Hobeika, Y., and Bugiotti, F.
(2024). To prompt or not to prompt: Navigating the
use of Large Language Models for integrating and
modeling heterogeneous data. Data & Knowledge En-
gineering, 152:102313.
Saeedi, A., David, L., and Rahm, E. (2021). Matching Enti-
ties from Multiple Sources with Hierarchical Agglom-
erative Clustering:. In Proceedings of the 13th IC3K,
pages 40–50. SciTePress.
Sheetrit, E., Brief, M., Mishaeli, M., and Elisha, O. (2024).
ReMatch: Retrieval Enhanced Schema Matching with
LLMs. arXiv:2403.01567 [cs].
Traeger, L., Behrend, A., and Karabatis, G. (2022). In-
teplato: Generating mappings of heterogeneous rela-
tional schemas using unsupervised learning. In 2022
CSCI, pages 426–431.
Traeger, L., Behrend, A., and Karabatis, G. (2024).
Scoping: Towards Streamlined Entity Collections
for Multi-Sourced Entity Resolution with Self-
Supervised Agents. pages 107–115.
Vogel, L., Bodensohn, J.-M., and Binnig, C. (2024).
WikiDBs: A Large-Scale Corpus Of Relational
Databases From Wikidata.
Zeakis, A., Papadakis, G., Skoutas, D., and Koubarakis, M.
(2023). Pre-Trained Embeddings for Entity Resolu-
tion: An Experimental Analysis. VLDB, 16(9):2225–
2238.
Zeng, X., Wang, P., Mao, Y., Chen, L., Liu, X., and Gao,
Y. (2024). MultiEM: Efficient and Effective Unsuper-
vised Multi-Table Entity Matching. pages 3421–3434.
IEEE Computer Society.
Zezhou Huang, Guo, Jia, and Wu, Eugene (2024). Trans-
form Table to Database Using Large Language Mod-
els. 2nd International Workshop TaDA@VLDB.
ICEIS 2025 - 27th International Conference on Enterprise Information Systems
50