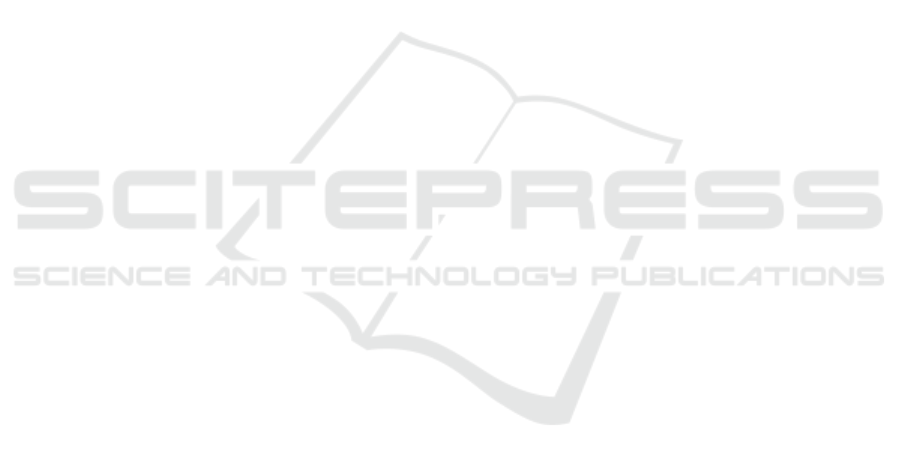
REFERENCES
Benmimoun, M. and Eckstein, L. (2014). Detection of criti-
cal driving situations for naturalistic driving studies by
means of an automated process. Journal of Intelligent
Transportation and Urban Planning, 2(1):11–21.
Caesar, H., Bankiti, V., Lang, A. H., Vora, S., Liong, V. E.,
Xu, Q., Krishnan, A., Pan, Y., Baldan, G., and Bei-
jbom, O. (2020). nuscenes: A multimodal dataset for
autonomous driving. In Proceedings of the IEEE/CVF
conference on computer vision and pattern recogni-
tion, pages 11621–11631.
Epple, N., Hankofer, T., and Riener, A. (2020). Sce-
nario classes in naturalistic driving: Autoencoder-
based spatial and time-sequential clustering of sur-
rounding object trajectories. In 2020 IEEE 23rd In-
ternational Conference on Intelligent Transportation
Systems (ITSC), pages 1–6. IEEE.
Go, K. and Carroll, J. M. (2004). The blind men and the
elephant: Views of scenario-based system design. in-
teractions, 11(6):44–53.
Hauer, F., Gerostathopoulos, I., Schmidt, T., and Pretschner,
A. (2020). Clustering traffic scenarios using mental
models as little as possible. In 2020 IEEE Intelligent
Vehicles Symposium (IV), pages 1007–1012. IEEE.
Kruber, F., Wurst, J., and Botsch, M. (2018). An unsu-
pervised random forest clustering technique for au-
tomatic traffic scenario categorization. In 2018 21st
International conference on intelligent transportation
systems (ITSC), pages 2811–2818. IEEE.
Lee, Y., Jeon, J., Yu, J., and Jeon, M. (2020). Context-aware
multi-task learning for traffic scene recognition in au-
tonomous vehicles. In 2020 IEEE Intelligent Vehicles
Symposium (IV), pages 723–730. IEEE.
Lu, J., Yang, S., Zhang, B., and Cao, Y. (2023). A bev
scene classification method based on historical loca-
tion points and unsupervised learning. In 2023 7th
CAA International Conference on Vehicular Control
and Intelligence (CVCI), pages 1–6. IEEE.
Ma, Y., Yang, S., Lu, J., Feng, X., Yin, Y., and Cao, Y.
(2022). Analysis of autonomous vehicles accidents
based on dmv reports. In 2022 China Automation
Congress (CAC), pages 623–628. IEEE.
Menzel, T., Bagschik, G., and Maurer, M. (2018). Scenarios
for development, test and validation of automated ve-
hicles. In 2018 IEEE Intelligent Vehicles Symposium
(IV), pages 1821–1827. IEEE.
Nitsche, P., Thomas, P., Stuetz, R., and Welsh, R. (2017).
Pre-crash scenarios at road junctions: A clustering
method for car crash data. Accident Analysis & Pre-
vention, 107:137–151.
Ren, H., Gao, H., Chen, H., and Liu, G. (2022). A survey of
autonomous driving scenarios and scenario databases.
In 2022 9th International Conference on Dependable
Systems and Their Applications (DSA), pages 754–
762. IEEE.
Rsener, C., Fahrenkrog, F., Uhlig, A., et al. (2016). A
scenario-based assessment approach for automated
driving by using time series classification of human-
driving behaviour [c]. In IEEE, pages 35–42.
Sun, C., ShangGuan, W., and Chai, L. (2020). Vehicle be-
havior recognition and prediction method for intelli-
gent driving in highway scene. In 2020 Chinese Au-
tomation Congress (CAC), pages 555–560. IEEE.
Ulbrich, S., Menzel, T., Reschka, A., Schuldt, F., and Mau-
rer, M. (2015). Defining and substantiating the terms
scene, situation, and scenario for automated driving.
In 2015 IEEE 18th international conference on intel-
ligent transportation systems, pages 982–988. IEEE.
Yang, S., Chen, Y., Cao, Y., Wang, R., Shi, R., and Lu, J.
(2022). Lane change trajectory prediction based on
spatiotemporal attention mechanism. In 2022 IEEE
25th International Conference on Intelligent Trans-
portation Systems (ITSC), pages 2366–2371. IEEE.
Zhu, B., Zhang, P.-x., Zhao, J., Chen, H., Xu, Z., Zhao, X.,
and Deng, W. (2019). Review of scenario-based vir-
tual validation methods for automated vehicles. China
Journal of Highway and Transport, 32(6):1–19.
Recognition of Typical Highway Driving Scenarios for Intelligent Connected Vehicles Based on Long Short-Term Memory Network
33