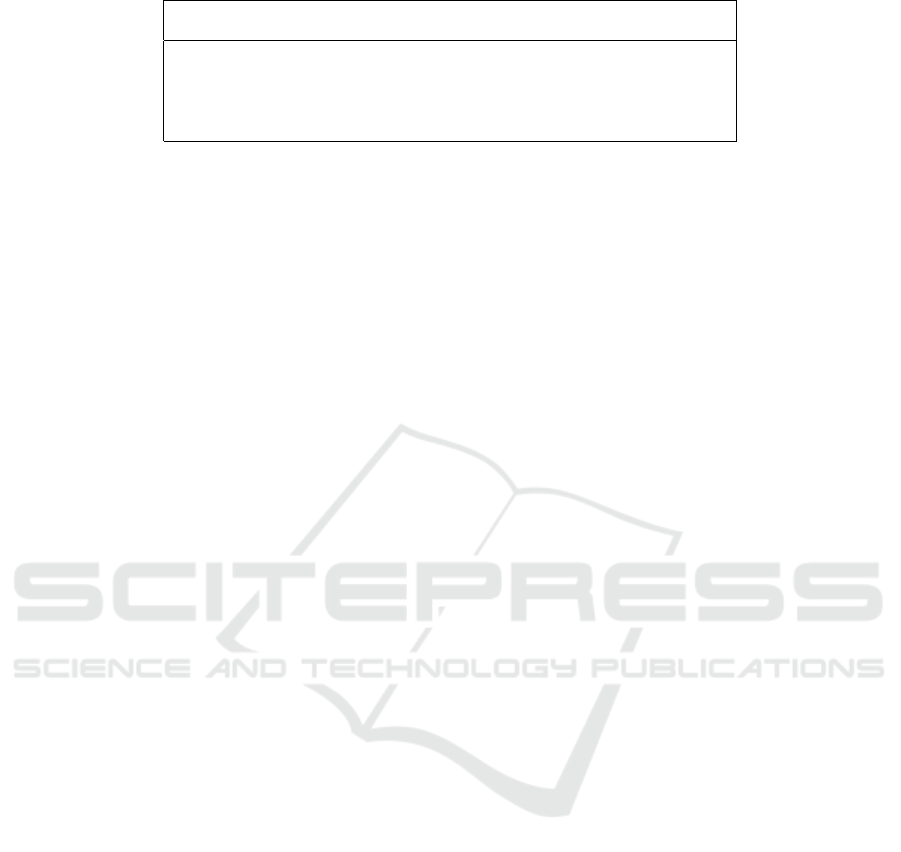
Table 9: Confidence intervals.
HYPOTHESIS Original Sample Bias 2.5% 97.5% Decision
sample mean
H1 0.477 0.475 -0.002 0.303 0.618 SUPPORTED
H2 0.053 0.053 -0.000 -0.104 0.194 NOT SUPPORTED
H3 0.175 0.174 -0.001 0.011 0.347 SUPPORTED
H4 0.019 0.018 -0.001 -0.053 0.087 NOT SUPPORTED
H5 0.899 0.898 -0.000 0.856 0.936 SUPPORTED
Performance Expectancy, Effort Expectancy, Social
Influence, and Behavioral Intention, we gain insights
into the factors impacting the acceptance and utiliza-
tion of AI technologies in educational settings. The
analysis provides a comprehensive understanding of
the attitudes, perceptions, and intentions of educa-
tors and students towards AI integration in education.
By validating hypotheses and interpreting path coeffi-
cients, we can ascertain the significance of these fac-
tors in driving the adoption of AI technologies. Ulti-
mately, this analytical approach enables us to draw
conclusions about the readiness and propensity of
educational stakeholders to embrace AI innovations,
thereby informing strategies for successful implemen-
tation and integration of AI technologies in educa-
tional practices.
In conclusion, the UTAUT model highlights Be-
havioral Intention as a strong predictor of Use Be-
havior, driven mainly by Performance Expectancy
and Social Influence. Effort Expectancy and Facil-
itating Conditions show no significant impact. The
significant indirect effect of Performance Expectancy
on Use Behavior underscores the importance of per-
ceived performance benefits.
Teachers are more likely to adopt AI if they be-
lieve it enhances job performance, such as making
lessons engaging or reducing workload. Clear ben-
efits are essential for AI adoption in education.
Another important finding is that when teachers
express a desire or intention to use AI, they are very
likely to actually use it. This shows that developing a
positive attitude toward AI early on is key because
once teachers decide they want to use it, they will
probably follow through.
Social pressure from colleagues or administrators
has some influence but isn’t as important. While it
can help to have others around them encouraging AI
use, teachers are mostly driven by their own beliefs
about the technology’s benefits.
Finally, having access to resources and support
does not strongly affect teachers’ decision to adopt
AI. While it helps, what really matters is whether
teachers see value in using AI. Schools should focus
more on showing the practical benefits of AI rather
than just providing resources.
REFERENCES
Abbad, M. M. (2021). Using the utaut model to understand
students’ usage of e-learning systems in developing
countries. Education and information technologies,
26(6):7205–7224.
Almaiah, M. A., Alamri, M. M., and Al-Rahmi, W. (2019).
Applying the utaut model to explain the students’ ac-
ceptance of mobile learning system in higher educa-
tion. Ieee Access, 7:174673–174686.
Chiu, T. K. (2023). The impact of generative ai (genai) on
practices, policies and research direction in education:
A case of chatgpt and midjourney. Interactive Learn-
ing Environments, pages 1–17.
Davis, F. D. (1989). Perceived usefulness, perceived ease of
use, and user acceptance of information technology.
MIS quarterly, pages 319–340.
Grani
´
c, A. (2022). Educational technology adoption: A sys-
tematic review. Education and Information Technolo-
gies, 27(7):9725–9744.
Hair, J. and Alamer, A. (2022). Partial least squares struc-
tural equation modeling (pls-sem) in second language
and education research: Guidelines using an applied
example. Research Methods in Applied Linguistics,
1(3):100027.
Marikyan, M. and Papagiannidis, P. (2021). Unified the-
ory of acceptance and use of technology. TheoryHub
book.
Marques, B., Villate, J., and Carvalho, C. V. (2010). Tech-
nology acceptance on higher education: The case of
an engineer’s school. In ICERI2010 Proceedings,
pages 5094–5102. IATED.
Raffaghelli, J. E., Rodr
´
ıguez, M. E., Guerrero-Rold
´
an, A.-
E., and Ba
˜
neres, D. (2022). Applying the utaut model
to explain the students’ acceptance of an early warning
system in higher education. Computers & Education,
182:104468.
Smiderle, R., Rigo, S. J., Marques, L. B., Pec¸anha de Mi-
randa Coelho, J. A., and Jaques, P. A. (2020). The
impact of gamification on students’ learning, engage-
ment and behavior based on their personality traits.
Smart Learning Environments, 7(1):3.
Venkatesh, V., Morris, M. G., Davis, G. B., and Davis, F. D.
(2003). User acceptance of information technology:
Toward a unified view. MIS quarterly, pages 425–478.
Xue, L., Rashid, A. M., and Ouyang, S. (2024). The unified
theory of acceptance and use of technology (utaut) in
higher education: A systematic review. SAGE Open,
14(1):21582440241229570.
CSEDU 2025 - 17th International Conference on Computer Supported Education
198