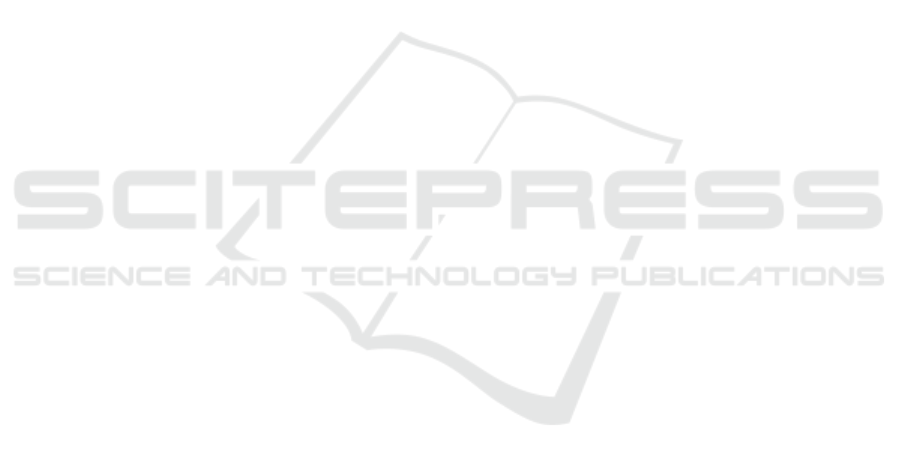
Lykourentzou, I., Antoniou, A., Naudet, Y., and Dow,
S. P. (2016). Personality matters: Balancing for
personality types leads to better outcomes for crowd
teams. In Proceedings of the 19th ACM Conference
on Computer-Supported Cooperative Work and Social
Computing, pages 260–273. Association for Comput-
ing Machinery.
Martinez-Maldonado, R. (2019). A handheld classroom
dashboard: Teachers’ perspectives on the use of real-
time collaborative learning analytics. International
Journal of Computer-Supported Collaborative Learn-
ing, 14:383–411.
Martinez-Maldonado, R., Echeverria, V., Fernandez Nieto,
G., and Buckingham Shum, S. (2020). From data to
insights: A layered storytelling approach for multi-
modal learning analytics. In Proceedings of the 2020
CHI Conference on Human Factors in Computing Sys-
tems, page 1–15, New York, NY, USA. Association
for Computing Machinery.
Martinez-Maldonado, R., Yacef, K., and Kay, J. (2015).
Tscl: A conceptual model to inform understanding
of collaborative learning processes at interactive table-
tops. International Journal of Human-Computer Stud-
ies, 83:62–82.
Menzel, L., Gombert, S., Weidlich, J., Fink, A., Frey,
A., and Drachsler, H. (2023). Why you should give
your students automatic process feedback on their
collaboration: Evidence from a randomized experi-
ment. In European Conference on Technology En-
hanced Learning ECTEL, pages 198–212. Springer.
Neumayr, T. and Augstein, M. (2020). A systematic re-
view of personalized collaborative systems. Frontiers
in Computer Science, 2:562679.
Page, M., McKenzie, J., Bossuyt, P., Boutron, I., and Hoff-
mann, T. (2021). The prisma 2020 statement: an up-
dated guideline for reporting systematic reviews. Sys-
tematic reviews, 10(1):1–11.
Praharaj, S., Scheffel, M., Schmitz, M., Specht, M., and
Drachsler, H. (2022). Towards collaborative conver-
gence: Quantifying collaboration quality with auto-
mated co-located collaboration analytics. In 12th In-
ternational Learning Analytics and Knowledge Con-
ference, LAK22, page 358–369, New York, NY, USA.
Association for Computing Machinery.
Rau, M. A., Bowman, H. E., and Moore, J. W. (2017). An
adaptive collaboration script for learning with multi-
ple visual representations in chemistry. Computers
and Education, 109:38–55.
Saqr, M., Fors, U., Tedre, M., and Nouri, J. (2018). How
social network analysis can be used to monitor online
collaborative learning and guide an informed interven-
tion. PLOS ONE, 13(3):1–22.
Serrano Iglesias, S., Spikol, D., Bote Lorenzo, M. L., and
Ouhaichi, H. (2021). Adaptable smart learning en-
vironments supported by multimodal learning analyt-
ics. In Proceedings of the LA4SLE 2021 Workshop:
Learning Analytics for Smart Learning Environments,
pages 24–30.
Som, A., Kim, S., Lopez-Prado, B., Dhamija, S., Alozie, N.,
and Tamrakar, A. (2021). Automated student group
collaboration assessment and recommendation system
using individual role and behavioral cues. Frontiers in
Computer Science, 3:16.
Splichal, J. M., Oshima, J., and Oshima, R. (2018). Regu-
lation of collaboration in project-based learning medi-
ated by cscl scripting reflection. Computers and Edu-
cation, 125:132–145.
Strauss, S., Tunnigkeit, I., Eberle, J., vom Bovert, L.,
Avdullahu, A., Schmittchen, M., and Rummel, N.
(2023). Differential effects of a script and a group
awareness tool on the acquisition of collaboration
skills. In Proceedings of the 16th International Con-
ference on Computer-Supported Collaborative Learn-
ing CSCL, pages 75–82. International Society of the
Learning Sciences.
Tegos, S., Demetriadis, S., and Karakostas, A. (2015). Pro-
moting academically productive talk with conversa-
tional agent interventions in collaborative learning set-
tings. Computers and Education, 87:309–325.
Tomic, B. B., Kijevcanin, A. D., sevarac, Z. V., and Jo-
vanovic, J. M. (2023). An ai-based approach for grad-
ing students’ collaboration. IEEE Transactions on
Learning Technologies, 16(3):292–305.
Vatrapu, R., Teplovs, C., Fujita, N., and Bull, S. (2011). To-
wards visual analytics for teachers’ dynamic diagnos-
tic pedagogical decision-making. In Proceedings of
the 1st international conference on learning analytics
and knowledge, pages 93–98. Association for Com-
puting Machinery.
Venegas-Reynoso, A., Gaytan-Lugo, L. S., and Martinez-
Maldonado, R. (2018). From touches to teamwork
constructs: Towards automatically visualising collab-
oration processes. In Proceedings of the 7th Mexican
Conference on Human-Computer Interaction, New
York, NY, USA. Association for Computing Machin-
ery.
Vogel, F., Wecker, C., Kollar, I., and Fischer, F. (2017).
Socio-cognitive scaffolding with computer-supported
collaboration scripts: A meta-analysis. Educational
Psychology Review, 29:477–511.
Yang, K. B., Echeverria, V., Lu, Z., Mao, H., Holstein, K.,
Rummel, N., and Aleven, V. (2023). Pair-up: Pro-
totyping human-ai co-orchestration of dynamic tran-
sitions between individual and collaborative learning
in the classroom. In Proceedings of the 2023 CHI
Conference on Human Factors in Computing Systems,
number 453 in CHI ’23, New York, NY, USA. Asso-
ciation for Computing Machinery.
Zamecnik, A., Kovanovic, V., Grossmann, G., Joksimovic,
S., Jolliffe, G., Gibson, D., and Pardo, A. (2022).
Team interactions with learning analytics dashboards.
Computers and Education, 185:104514.
Zheng, L., Fan, Y., Gao, L., Huang, Z., Chen, B., and Long,
M. (2024). Using ai-empowered assessments and per-
sonalized recommendations to promote online collab-
orative learning performance. Journal of Research on
Technology in Education, pages 1–27.
CSEDU 2025 - 17th International Conference on Computer Supported Education
916