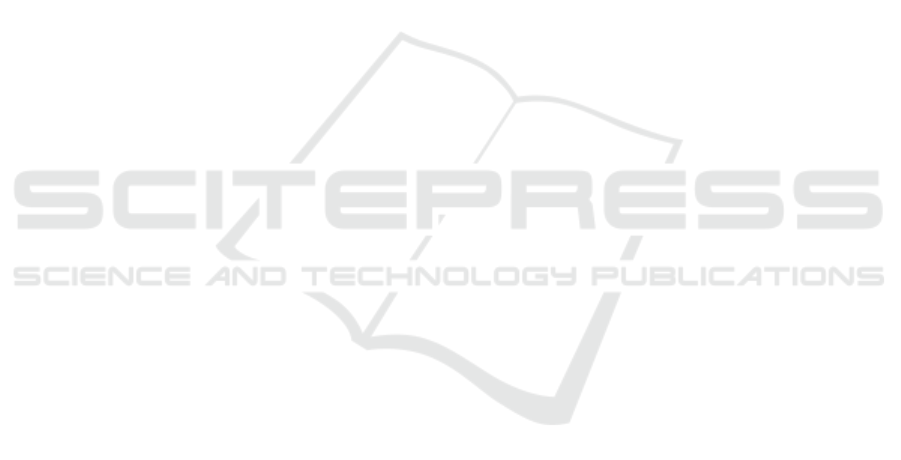
contributing to the development of smart cities. Fur-
thermore, future research could also explore the appli-
cation of the modular detection system beyond traffic
monitoring, such as pedestrian flow analysis, public
safety enhancement or intelligent parking detection.
In conclusion, the modular detection system de-
veloped in this work advances the development of
smart cities by providing a scalable, flexible and
adaptable solution for traffic monitoring. This ap-
proach not only meets current urban mobility de-
mands but also sets a strong foundation for future in-
novations in the intelligent infrastructures field, en-
suring that cities can address the emerging challenges
in traffic safety, efficiency and sustainability.
ACKNOWLEDGEMENTS
This research was funded by MCIN/AEI/10.13039/
501100011033 grant numbers PID2021-124335OB-
C21, PID2022-140554OB-C32 and PDC2022-
133684-C31.
REFERENCES
Arnold, E., Al-Jarrah, O. Y., Dianati, M., Fallah, S., Ox-
toby, D., and Mouzakitis, A. (2019). A survey on
3d object detection methods for autonomous driving
applications. IEEE Transactions on Intelligent Trans-
portation Systems, 20(10):3782–3795.
Borau Bernad, J. (2024). RoadVision3D. https://github.
com/jborau/RoadVision3D.
Borau Bernad, J., Ramajo-Ballester,
´
A., and Armin-
gol Moreno, J. M. (2024). Three-dimensional vehicle
detection and pose estimation in monocular images
for smart infrastructures. Mathematics, 12(13):2027.
Geiger, A., Lenz, P., and Urtasun, R. (2012). Are we ready
for autonomous driving? the kitti vision benchmark
suite. 2012 IEEE Conference on Computer Vision and
Pattern Recognition, pages 3354–3361.
Jain, N. K., Saini, R., and Mittal, P. (2019). A review
on traffic monitoring system techniques. Soft com-
puting: Theories and applications: Proceedings of
SoCTA 2017, pages 569–577.
Lang, A. H., Vora, S., Caesar, H., Zhou, L., Yang, J., and
Beijbom, O. (2019). Pointpillars: Fast encoders for
object detection from point clouds. In Proceedings
of the IEEE/CVF conference on computer vision and
pattern recognition, pages 12697–12705.
Li, Z., Jia, J., and Shi, Y. (2024). Monolss: Learnable
sample selection for monocular 3d detection. In 2024
International Conference on 3D Vision (3DV), pages
1125–1135.
Liu, X., Xue, N., and Wu, T. (2022). Learning auxiliary
monocular contexts helps monocular 3d object detec-
tion. In Proceedings of the AAAI Conference on Arti-
ficial Intelligence, volume 36, pages 1810–1818.
Liu, Z., Wu, Z., and T’oth, R. (2020). Smoke: Single-stage
monocular 3d object detection via keypoint estima-
tion. 2020 IEEE/CVF Conference on Computer Vision
and Pattern Recognition (CVPR), pages 4289–4298.
MMDetection3D Contributors (2020). MMDetection3D:
OpenMMLab next-generation platform for general
3D object detection. https://github.com/open-mmlab/
mmdetection3d.
Owais, M. (2024). Deep learning for integrated ori-
gin–destination estimation and traffic sensor location
problems. IEEE Transactions on Intelligent Trans-
portation Systems, 25(7):6501–6513.
Owais, M. and Shahin, A. I. (2022). Exact and heuris-
tics algorithms for screen line problem in large size
networks: Shortest path-based column generation ap-
proach. IEEE Transactions on Intelligent Transporta-
tion Systems, 23(12):24829–24840.
Qian, R., Lai, X., and Li, X. (2022). 3d object detection for
autonomous driving: A survey. Pattern Recognition,
130:108796.
Sindagi, V. A., Zhou, Y., and Tuzel, O. (2019). Mvx-net:
Multimodal voxelnet for 3d object detection. In 2019
International Conference on Robotics and Automation
(ICRA), pages 7276–7282.
Wang, T., Xinge, Z., Pang, J., and Lin, D. (2022). Proba-
bilistic and geometric depth: Detecting objects in per-
spective. In Conference on Robot Learning, pages
1475–1485. PMLR.
Wang, Y., Mao, Q., Zhu, H., Deng, J., Zhang, Y., Ji, J., Li,
H., and Zhang, Y. (2023). Multi-modal 3d object de-
tection in autonomous driving: a survey. International
Journal of Computer Vision, 131(8):2122–2152.
Yan, Y., Mao, Y., and Li, B. (2018). Second:
Sparsely embedded convolutional detection. Sensors,
18(10):3337.
Yu, F., Wang, D., Shelhamer, E., and Darrell, T. (2018).
Deep layer aggregation. In Proceedings of the IEEE
conference on computer vision and pattern recogni-
tion, pages 2403–2412.
Yu, H., Luo, Y., Shu, M., Huo, Y., Yang, Z., Shi, Y., Guo,
Z., Li, H., Hu, X., Yuan, J., and Nie, Z. (2022). Dair-
v2x: A large-scale dataset for vehicle-infrastructure
cooperative 3d object detection. In Proceedings of the
IEEE/CVF Conference on Computer Vision and Pat-
tern Recognition (CVPR), pages 21361–21370.
Zanella, A., Bui, N., Castellani, A., Vangelista, L., and
Zorzi, M. (2014). Internet of things for smart cities.
IEEE Internet of Things journal, 1(1):22–32.
Zhang, Y., Carballo, A., Yang, H., and Takeda, K. (2023).
Perception and sensing for autonomous vehicles under
adverse weather conditions: A survey. ISPRS Journal
of Photogrammetry and Remote Sensing, 196:146–
177.
Zhou, X., Wang, D., and Kr
¨
ahenb
¨
uhl, P. (2019). Objects as
points. arXiv preprint arXiv:1904.07850.
Zhou, Y. and Tuzel, O. (2018). Voxelnet: End-to-end learn-
ing for point cloud based 3d object detection. In Pro-
ceedings of the IEEE Conference on Computer Vision
and Pattern Recognition (CVPR).
A Modular Detection System for Smart Cities: Integrating Monocular and LiDAR Solutions for Scalable Traffic Monitoring
333