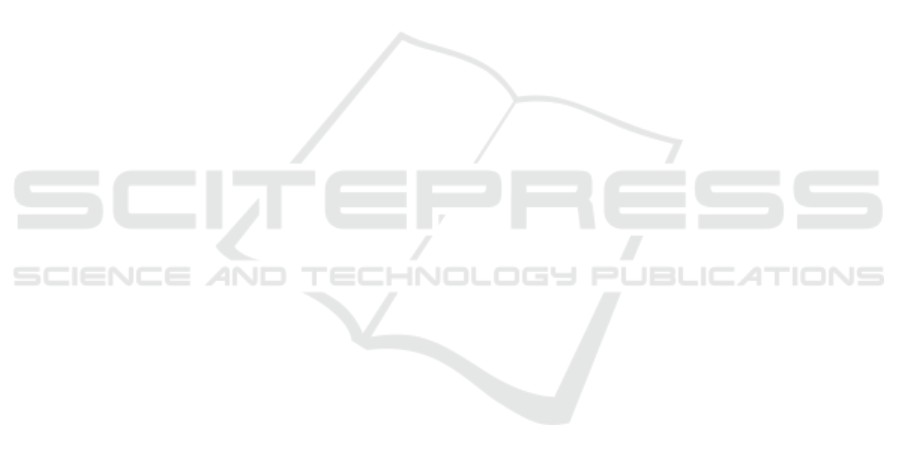
REFERENCES
Alves, P. and Cipriano, B. P. (2023). The centaur
programmer–How Kasparov’s Advanced Chess spans
over to the software development of the future. arXiv
preprint arXiv:2304.11172.
Babe, H. M., Nguyen, S., Zi, Y., Guha, A., Feldman, M. Q.,
and Anderson, C. J. (2023a). Studenteval: A bench-
mark of student-written prompts for large language
models of code. arXiv preprint arXiv:2306.04556.
Babe, H. M., Nguyen, S., Zi, Y., Guha, A., Feldman, M. Q.,
and Anderson, C. J. (2023b). StudentEval: A Bench-
mark of Student-Written Prompts for Large Language
Models of Code. arXiv:2306.04556 [cs].
Chen, L., Zaharia, M., and Zou, J. (2023). How is Chat-
GPT’s behavior changing over time? arXiv preprint
arXiv:2307.09009.
Cipriano, B. P. and Alves, P. (2024a). ”ChatGPT Is Here
to Help, Not to Replace Anybody” - An Evaluation
of Students’ Opinions On Integrating ChatGPT In CS
Courses. arXiv preprint arXiv:2404.17443.
Cipriano, B. P. and Alves, P. (2024b). LLMs Still
Can’t Avoid Instanceof: An investigation Into GPT-
3.5, GPT-4 and Bard’s Capacity to Handle Object-
Oriented Programming Assignments. In Proceedings
of the IEEE/ACM 46th International Conference on
Software Engineering: Software Engineering Educa-
tion and Training (ICSE-SEET).
Denny, P., Kumar, V., and Giacaman, N. (2023). Conversing
with Copilot: Exploring Prompt Engineering for Solv-
ing CS1 Problems Using Natural Language. In Pro-
ceedings of the 54th ACM Technical Symposium on
Computer Science Education V. 1, pages 1136–1142,
Toronto ON Canada. ACM. SIGCSE 2023.
Denny, P., Leinonen, J., Prather, J., Luxton-Reilly, A.,
Amarouche, T., Becker, B. A., and Reeves, B. N.
(2024). Prompt Problems: A New Programming Ex-
ercise for the Generative AI Era. In Proceedings of the
55th ACM Technical Symposium on Computer Science
Education V. 1, pages 296–302.
Destefanis, G., Bartolucci, S., and Ortu, M. (2023). A Pre-
liminary Analysis on the Code Generation Capabili-
ties of GPT-3.5 and Bard AI Models for Java Func-
tions. arXiv preprint arXiv:2305.09402.
Finnie-Ansley, J., Denny, P., Becker, B. A., Luxton-Reilly,
A., and Prather, J. (2022). The robots are com-
ing: Exploring the Implications of OpenAI Codex
on Introductory Programming. In Proceedings of the
24th Australasian Computing Education Conference,
pages 10–19.
Finnie-Ansley, J., Denny, P., Luxton-Reilly, A., Santos,
E. A., Prather, J., and Becker, B. A. (2023). My ai
wants to know if this will be on the exam: Testing
openai’s codex on cs2 programming exercises. In Pro-
ceedings of the 25th Australasian Computing Educa-
tion Conference, pages 97–104.
Hellas, A., Leinonen, J., Sarsa, S., Koutcheme, C., Ku-
janp
¨
a
¨
a, L., and Sorva, J. (2023). Exploring the Re-
sponses of Large Language Models to Beginner Pro-
grammers’ Help Requests.
Kazemitabaar, M., Hou, X., Henley, A., Ericson, B. J.,
Weintrop, D., and Grossman, T. (2023). How novices
use llm-based code generators to solve cs1 coding
tasks in a self-paced learning environment.
Lau, S. and Guo, P. (2023). From ”Ban it till we understand
it” to ”Resistance is futile”: How university program-
ming instructors plan to adapt as more students use AI
code generation and explanation tools such as Chat-
GPT and GitHub Copilot.
Leinonen, J., Denny, P., MacNeil, S., Sarsa, S., Bernstein,
S., Kim, J., Tran, A., and Hellas, A. (2023). Compar-
ing Code Explanations Created by Students and Large
Language Models. arXiv preprint arXiv:2304.03938.
Liffiton, M., Sheese, B., Savelka, J., and Denny, P.
(2023). CodeHelp: Using Large Language Models
with Guardrails for Scalable Support in Programming
Classes.
Naumova, E. N. (2023). A mistake-find exercise: a
teacher’s tool to engage with information innovations,
ChatGPT, and their analogs. Journal of Public Health
Policy, 44(2):173–178.
OpenAI (2023). How can educators respond to students
presenting ai-generated content as their own? https://
shorturl.at/rUDlh. [Online; last accessed 03-October-
2023].
Ouh, E. L., Gan, B. K. S., Shim, K. J., and Wlodkowski,
S. (2023). Chatgpt, can you generate solutions for my
coding exercises? an evaluation on its effectiveness
in an undergraduate java programming course. arXiv
preprint arXiv:2305.13680.
Prasad, S., Greenman, B., Nelson, T., and Krishnamurthi,
S. (2023). Generating Programs Trivially: Student
Use of Large Language Models. In Proceedings of the
ACM Conference on Global Computing Education Vol
1, pages 126–132, Hyderabad India. ACM.
Prather, J., Reeves, B. N., Denny, P., Becker, B. A.,
Leinonen, J., Luxton-Reilly, A., Powell, G., Finnie-
Ansley, J., and Santos, E. A. (2023). “It’s Weird That
it Knows What I Want”: Usability and Interactions
with Copilot for Novice Programmers. ACM Transac-
tions on Computer-Human Interaction, 31(1):1–31.
Reeves, B., Sarsa, S., Prather, J., Denny, P., Becker, B. A.,
Hellas, A., Kimmel, B., Powell, G., and Leinonen, J.
(2023). Evaluating the performance of code genera-
tion models for solving parsons problems with small
prompt variations. In Proceedings of the 2023 Confer-
ence on Innovation and Technology in Computer Sci-
ence Education V. 1, pages 299–305.
Savelka, J., Agarwal, A., An, M., Bogart, C., and Sakr, M.
(2023). Thrilled by Your Progress! Large Language
Models (GPT-4) No Longer Struggle to Pass Assess-
ments in Higher Education Programming Courses.
Sridhar, P., Doyle, A., Agarwal, A., Bogart, C., Savelka, J.,
and Sakr, M. (2023). Harnessing llms in curricular
design: Using gpt-4 to support authoring of learning
objectives.
Treude, C. (2023). Navigating Complexity in Software En-
gineering: A Prototype for Comparing GPT-n Solu-
tions. arXiv:2301.12169 [cs].
Xu, F. F., Alon, U., Neubig, G., and Hellendoorn, V. J.
(2022). A Systematic Evaluation of Large Language
Models of Code. In Proceedings of the 6th ACM
SIGPLAN International Symposium on Machine Pro-
gramming, pages 1–10, San Diego CA USA. ACM.
"Give Me the Code": Log Analysis of First-Year CS Students’ Interactions with GPT
207