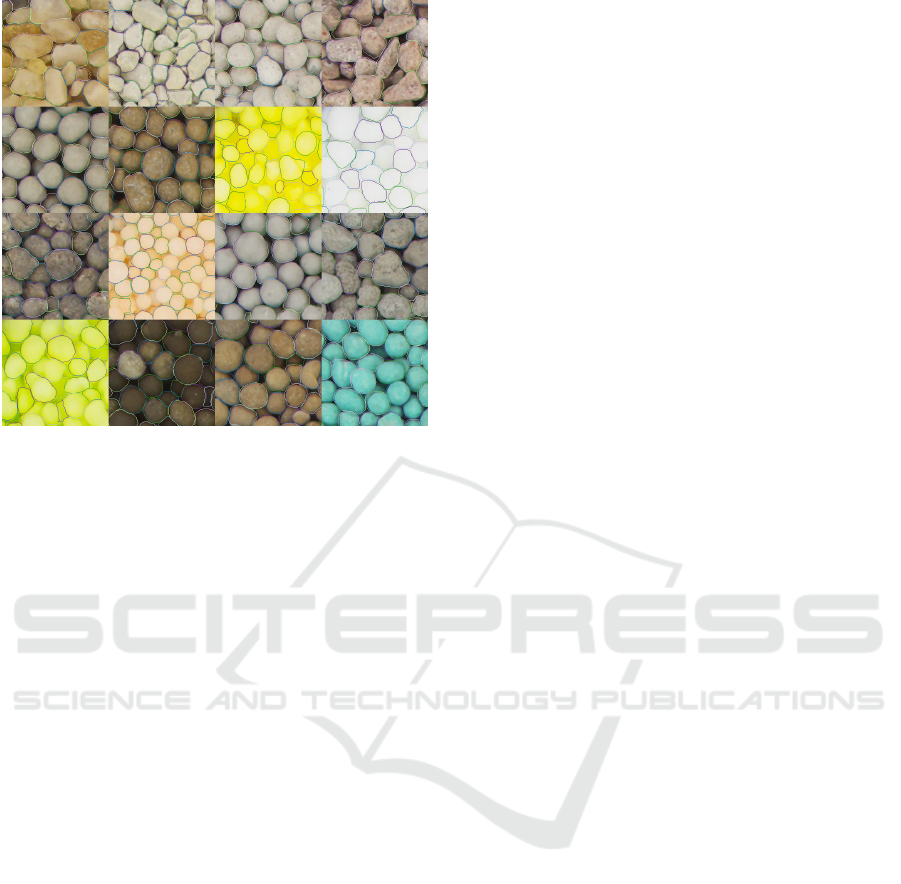
Figure 13: Cuttings from images of the 16 fertilizers used
in the project.
47736 contours of which 47693 were correctly rec-
ognized yielding a recognition rate of 99.901%. Pro-
vided the photo box presented in this paper is used in
similar situations, this result can be considered reli-
able for a real-world application too.
Future work will now involve further analysis of
the resulting contours in terms of size, shape and sta-
tistical distribution of grain sizes.
4 CONCLUSIONS
We presented a CV-ML pipeline composed of state-
of-the-art tools, namely YOLO11 and SAM2, for the
detection and classification of fertilizer grains. Using
a Raspberry Pi HQ Camera mounted in a purpose-
built imaging box with a simple diffuse light source,
the system setup is fairly simple. Training the detec-
tor and classifier in an active learning approach from
only a few manually labeled images resulted in a test
accuracy of 99.996%, despite the simplicity of our
proposed setup. Although our focus was the classi-
fication of fertilizer as part of a bigger system, our
proposed setup and pipeline can also serve as a tem-
plate for other similar applications.
ACKNOWLEDGEMENTS
This work is supported by the Federal Ministry
for Economic Affairs and Climate Action, ZIM,
KK5243405LF3.
REFERENCES
Adhinata, F. D., Wahyono, and Sumiharto, R. (2024). A
comprehensive survey on weed and crop classification
using machine learning and deep learning. Artificial
Intelligence in Agriculture, 13:45–63.
Akyon, F. C., Onur Altinuc, S., and Temizel, A. (2022).
Slicing aided hyper inference and fine-tuning for small
object detection. In 2022 IEEE International Con-
ference on Image Processing (ICIP), pages 966–970.
IEEE.
Alif, M. A. R. and Hussain, M. (2024). Yolov1 to yolov10:
A comprehensive review of yolo variants and their ap-
plication in the agricultural domain.
Canny, J. (1986). A computational approach to edge de-
tection. IEEE Transactions on Pattern Analysis and
Machine Intelligence, PAMI-8(6):679–698.
Dhanya, V. G., Subeesh, A., Kushwaha, N. L., Vish-
wakarma, D. K., Nagesh Kumar, T., Ritika, G., and
Singh, A. N. (2022). Deep learning based computer
vision approaches for smart agricultural applications.
Artificial Intelligence in Agriculture, 6:211–229.
Ghazal, S., Munir, A., and Qureshi, W. S. (2024). Com-
puter vision in smart agriculture and precision farm-
ing: Techniques and applications. Artificial Intelli-
gence in Agriculture, 13:64–83.
Heikkila, J. and Silven, O. (1997). A four-step camera cal-
ibration procedure with implicit image correction. In
Proceedings of IEEE Computer Society Conference
on Computer Vision and Pattern Recognition, pages
1106–1112. IEEE Comput. Soc.
Light, R. A. (2017). Mosquitto: server and client imple-
mentation of the mqtt protocol. The Journal of Open
Source Software, 2(13):265.
MQTT.org (2024). Mqtt: The standard for iot messaging.
Ravi, N., Gabeur, V., Hu, Y.-T., Hu, R., Ryali, C., Ma,
T., Khedr, H., R
¨
adle, R., Rolland, C., Gustafson, L.,
Mintun, E., Pan, J., Alwala, K. V., Carion, N., Wu,
C.-Y., Girshick, R., Doll
´
ar, P., and Feichtenhofer, C.
(2024). Sam 2: Segment anything in images and
videos. arXiv preprint arXiv:2408.00714.
Redmon, J., Divvala, S., Girshick, R., and Farhadi, A.
(2015). You only look once: Unified, real-time ob-
ject detection.
Sarkar, C., Gupta, D., Gupta, U., and Hazarika, B. B.
(2023). Leaf disease detection using machine learning
and deep learning: Review and challenges. Applied
Soft Computing, 145:110534.
Xie, C., Cang, Y., Lou, X., Xiao, H., Xu, X., Li, X., and
Zhou, W. (2024). A novel approach based on a modi-
fied mask r-cnn for the weight prediction of live pigs.
Artificial Intelligence in Agriculture, 12:19–28.
Zhang, Z. (2000). A flexible new technique for camera cal-
ibration. IEEE Transactions on Pattern Analysis and
Machine Intelligence, 22(11):1330–1334.
A Computer Vision Approach to Fertilizer Detection and Classification
569