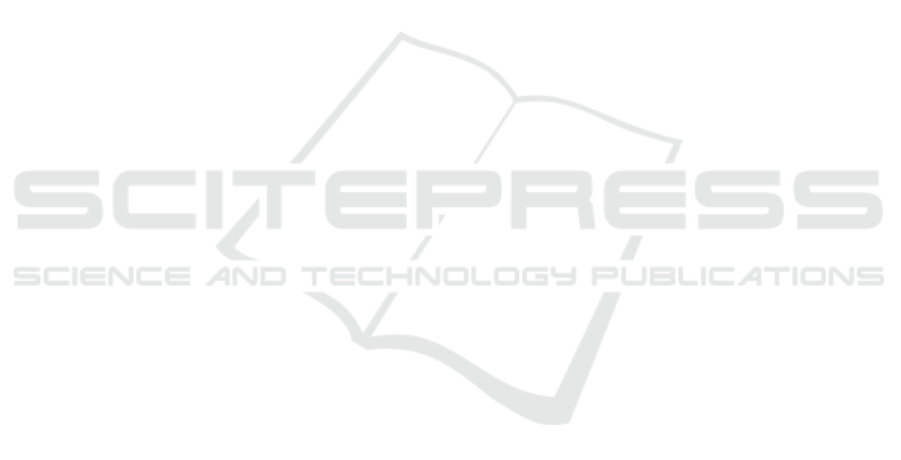
2021 ACM conference on fairness, accountability, and
transparency, pages 805–815.
Jyothsna, V., Prasad, R., and Prasad, K. M. (2011). A review
of anomaly based intrusion detection systems. Inter-
national Journal of Computer Applications, 28(7):26–
35.
Kalakoti, R., Bahsi, H., and N
˜
omm, S. (2024a). Improving
iot security with explainable ai: Quantitative evalua-
tion of explainability for iot botnet detection. IEEE
Internet of Things Journal.
Kalakoti, R., Bahsi, H., and N
˜
omm, S. (2024b). Explainable
federated learning for botnet detection in iot networks.
In 2024 IEEE International Conference on Cyber Se-
curity and Resilience (CSR), pages 01–08.
Kalakoti, R., N
˜
omm, S., and Bahsi, H. (2022). In-depth
feature selection for the statistical machine learning-
based botnet detection in iot networks. IEEE Access,
10:94518–94535.
Kalakoti, R., N
˜
omm, S., and Bahsi, H. (2023). Improving
transparency and explainability of deep learning based
iot botnet detection using explainable artificial intel-
ligence (xai). In 2023 International Conference on
Machine Learning and Applications (ICMLA), pages
595–601. IEEE.
Kalakoti, R., N
˜
omm, S., and Bahsi, H. (2024c). Enhancing
iot botnet attack detection in socs with an explainable
active learning framework. In 2024 IEEE World AI
IoT Congress (AIIoT), pages 265–272. IEEE.
Kidmose, E., Stevanovic, M., Brandbyge, S., and Peder-
sen, J. M. (2020). Featureless discovery of correlated
and false intrusion alerts. IEEE Access, 8:108748–
108765.
Kumar, A. and Thing, V. L. (2024). Evaluating the explain-
ability of state-of-the-art machine learning-based iot
network intrusion detection systems. arXiv preprint
arXiv:2408.14040.
Lundberg, S. M. and Lee, S.-I. (2017). A unified approach
to interpreting model predictions. Advances in neural
information processing systems, 30.
Luss, R., Chen, P.-Y., Dhurandhar, A., Sattigeri, P., Shan-
mugam, K., and Tu, C.-C. (2019). Generating con-
trastive explanations with monotonic attribute func-
tions. arXiv preprint arXiv:1905.12698, 3.
Mane, S. and Rao, D. (2021). Explaining network intru-
sion detection system using explainable ai framework.
arXiv preprint arXiv:2103.07110.
Moustafa, N., Koroniotis, N., Keshk, M., Zomaya, A. Y.,
and Tari, Z. (2023). Explainable intrusion detection
for cyber defences in the internet of things: Opportu-
nities and solutions. IEEE Communications Surveys
& Tutorials, 25(3):1775–1807.
Rawal, A., McCoy, J., Rawat, D. B., Sadler, B. M., and
Amant, R. S. (2021). Recent advances in trustworthy
explainable artificial intelligence: Status, challenges,
and perspectives. IEEE Transactions on Artificial In-
telligence, 3(6):852–866.
Ribeiro, M. T., Singh, S., and Guestrin, C. (2016). ” why
should i trust you?” explaining the predictions of any
classifier. In Proceedings of the 22nd ACM SIGKDD
international conference on knowledge discovery and
data mining, pages 1135–1144.
Senevirathna, T., Siniarski, B., Liyanage, M., and Wang,
S. (2024). Deceiving post-hoc explainable ai (xai)
methods in network intrusion detection. In 2024 IEEE
21st Consumer Communications & Networking Con-
ference (CCNC), pages 107–112. IEEE.
Shin, I., Choi, Y., Kwon, T., Lee, H., and Song, J. (2019).
Platform design and implementation for flexible data
processing and building ml models of ids alerts. In
2019 14th Asia Joint Conference on Information Se-
curity (AsiaJCIS), pages 64–71. IEEE.
Shrikumar, A., Greenside, P., and Kundaje, A. (2017).
Learning important features through propagating ac-
tivation differences. In International conference on
machine learning, pages 3145–3153. PMLR.
Sundararajan, M., Taly, A., and Yan, Q. (2017). Axiomatic
attribution for deep networks. In International confer-
ence on machine learning, pages 3319–3328. PMLR.
Szczepa
´
nski, M., Chora
´
s, M., Pawlicki, M., and Kozik, R.
(2020). Achieving explainability of intrusion detec-
tion system by hybrid oracle-explainer approach. In
2020 International Joint Conference on neural net-
works (IJCNN), pages 1–8. IEEE.
Tavallaee, M., Bagheri, E., Lu, W., and Ghorbani, A. A.
(2009). A detailed analysis of the kdd cup 99 data
set. In 2009 IEEE symposium on computational intel-
ligence for security and defense applications, pages
1–6. Ieee.
Tsai, C.-F., Hsu, Y.-F., Lin, C.-Y., and Lin, W.-Y. (2009).
Intrusion detection by machine learning: A review. ex-
pert systems with applications, 36(10):11994–12000.
Vaarandi, R. (2021). A stream clustering algorithm for clas-
sifying network ids alerts. In 2021 IEEE International
Conference on Cyber Security and Resilience (CSR),
pages 14–19. IEEE.
Vaarandi, R. and Guerra-Manzanares, A. (2024). Stream
clustering guided supervised learning for classifying
nids alerts. Future Generation Computer Systems,
155:231–244.
Vaarandi, R. and M
¨
ases, S. (2022). How to build a soc on
a budget. In 2022 IEEE International Conference on
Cyber Security and Resilience (CSR), pages 171–177.
IEEE.
Van Ede, T., Aghakhani, H., Spahn, N., Bortolameotti, R.,
Cova, M., Continella, A., van Steen, M., Peter, A.,
Kruegel, C., and Vigna, G. (2022). Deepcase: Semi-
supervised contextual analysis of security events. In
2022 IEEE Symposium on Security and Privacy (SP),
pages 522–539. IEEE.
Wang, T., Zhang, C., Lu, Z., Du, D., and Han, Y. (2019).
Identifying truly suspicious events and false alarms
based on alert graph. In 2019 IEEE International Con-
ference on Big Data (Big Data), pages 5929–5936.
IEEE.
Woolson, R. F. (2005). Wilcoxon signed-rank test. Ency-
clopedia of Biostatistics, 8.
Zolanvari, M., Yang, Z., Khan, K., Jain, R., and Meskin,
N. (2021). Trust xai: Model-agnostic explanations for
ai with a case study on iiot security. IEEE internet of
things journal, 10(4):2967–2978.
ICISSP 2025 - 11th International Conference on Information Systems Security and Privacy
58