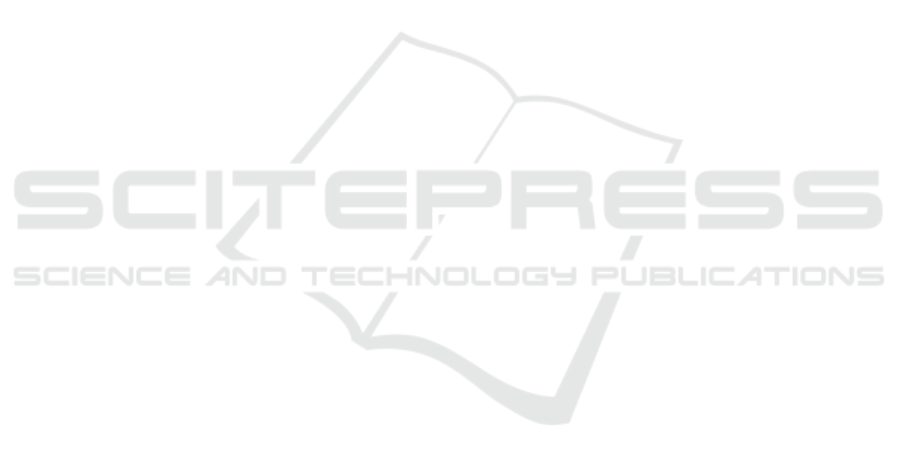
methodology used was thematic analysis, with key
items divided into classes based on frequency.
The responses to the survey revealed that AI will
significantly impact daily life, particularly in areas
such as medicine, education, and photo/video pro-
cessing. While there are no significant differences
between men and women in their perceptions of the
most affected domains, men are more aware of poten-
tial changes in Computer Science, autonomous driv-
ing, image and video processing, and chatbot usage.
Women, however, mention more the impact of losing
human abilities.
Both men and women perceive potential threats in
the same manner, with men more aware of war, AI
controlled drones, terrain recognition, and informa-
tional war. They also seem to be more aware of fake
news and disinformation.
Ethical reasons were more prominent among
women, with women expressing a desire to help peo-
ple in various aspects of life, such as saving people
from rare diseases and making society more comfort-
able. Men were more preoccupied with financial as-
pects, while women were more concerned with help-
ing others.
The study aimed to minimize potential risks and
validate the findings through construct validity, inter-
nal validity, and external validity.
The study revealed that both men and women had
distinct motivations and priorities when it came to
emerging technologies. While men focused more
on financial gains and advancements, women had
a stronger inclination towards ethical considerations
and helping others. Overall, the research shed light on
the gender differences in motivations and highlighted
the need for a balanced approach in the development
and implementation of emerging technologies.
In the future, we hope to expand the study to in-
clude a larger and more diverse cohort of students
from other universities, resulting in a widely compa-
rable data set across European or international institu-
tions. Analyzing the data not only in terms of gender
but also comparing differences among different na-
tionalities as well as the development over time for
several generations of students would be great next
steps, and the paper at hand is the first step in that
direction.
REFERENCES
Abdullah, S. M. (2019). Artificial intelligence (ai) and its
associated ethical issues. ICR Journal, 10:124–126.
AI bots have achieved the capability to interact with
humans and build relationships through conversations.
Baeza-Yates, R. (2022). Ethical challenges in ai. Proceed-
ings of the Fifteenth ACM International Conference
on Web Search and Data Mining, pages 1–2.
Banciu, D. and C
ˆ
ırnu, C. E. (2022). Ai ethics and data pri-
vacy compliance. In 2022 14th International Confer-
ence on Electronics, Computers and Artificial Intelli-
gence (ECAI), pages 1–5. IEEE.
Borenstein, J. and Howard, A. (2021). Emerging challenges
in ai and the need for ai ethics education. AI and
Ethics, 1:61–65.
Braun, V., Clarke, V., Hayfield, N., and Terry, G. (2019).
Handbook of Research Methods in Health Social Sci-
ences, chapter Thematic Analysis, pages 843–860.
Brown, J., Park, T., Chang, J., Andrus, M., Xiang, A., and
Custis, C. (2022). Attrition of workers with minori-
tized identities on ai teams. In Equity and Access in
Algorithms, Mechanisms, and Optimization, pages 1–
9.
Callas, V. . (1992). Predicting ethical values and training
needs in ethics. Journal of Business ethics, 11:761–
769.
Cave, S., Dihal, K., Drage, E., and McInerney, K. (2023).
Who makes ai? gender and portrayals of ai scientists
in popular film, 1920–2020. Public Understanding of
Science, 32:745–760.
Cernadas, E. and Calvo-Iglesias, E. (2020). Gender per-
spective in artificial intelligence (ai). ACM Interna-
tional Conference Proceeding Series, pages 173–176.
Fisher, E. J. P. and Fisher, E. (2023). A fresh look at ethical
perspectives on artificial intelligence applications and
their potential impacts at work and on people. Busi-
ness and Economic Research, 13(3):1–22.
Gallivan, M., Adya, M., Ahuja, M., Hoonakker, P., and
Woszczynski, A. (2006). Workforce diversity in the
it profession: Recognizing and resolving the shortage
of women and minority employees panel: Workforce
diversity in the it profession: Recognizing and resolv-
ing the shortage of women and minority employees.
pages 44–45.
Green, B. P. (2018). Ethical reflections on artificial intelli-
gence.
Jakesch, M., Buc¸inca, Z., Amershi, S., and Olteanu, A.
(2022). How different groups prioritize ethical val-
ues for responsible ai. pages 310–323. Association
for Computing Machinery.
Kennedy, J. A., Kray, L. J., and Kray, J. (2013). Who is
willing to sacrifice ethical values for money and so-
cial status?: Gender differences in reactions to ethical
compromises. Social Psychological and Personality
Science, 5.
Kennedy, J. A., Kray, L. J., and Kray, J. (2014). Gender
and reactions to ethical compromises 2 who is willing
to sacrifice ethical values for money and social sta-
tus? gender differences in reactions to ethical compro-
mises. Social Psychological and Personality Science,
5:52–59.
Kiger, M. E. and Varpio, L. (2020). Thematic analysis
of qualitative data: Amee guide no. 131. Medical
Teacher, 42(8):846–854.
CSEDU 2025 - 17th International Conference on Computer Supported Education
34