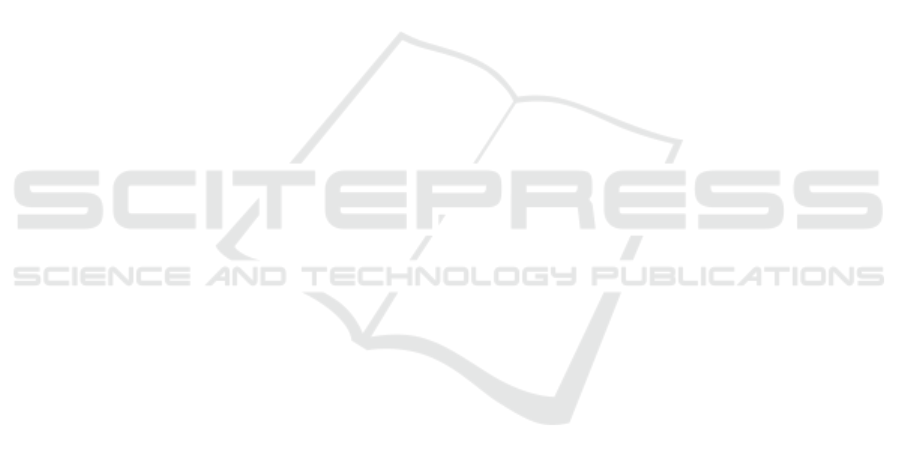
significance of correcting the dataset’s class imbal-
ance. However, the feature selection model using
MLP, SGD, and SMOTE performed relatively poorly,
indicating that this combination might not be as use-
ful in the network anomaly specific context. Over-
all, the results indicate that feature selection with a
CNN equipped with SGD, SMOTE, and XGBoost is
very successful when applied to network anomaly de-
tection tasks within a Federated Anomaly environ-
ment. To improve the results’ generalizability, fu-
ture research ought to investigate into other optimiza-
tion techniques for these models and their applica-
tions across various domains. Furthermore, testing
our experiments on different datasets would provide
a broader overview of the model’s performance.
ACKNOWLEDGEMENTS
The research leading to these results was supported
by the Ministry of Higher Education and Scientific
Research of Tunisia.
REFERENCES
Aburomman, A. A. and Reaz, M. B. I. (2016). A novel
svm-knn-pso ensemble method for intrusion detection
system. Applied Soft Computing, 38:360–372.
Ali, A. H., Charfeddine, M., Ammar, B., and Hamed,
B. B. (2024a). Intrusion detection schemes based on
synthetic minority oversampling technique and ma-
chine learning models. In 2024 IEEE 27th Interna-
tional Symposium on Real-Time Distributed Comput-
ing (ISORC), pages 1–8. IEEE.
Ali, A. H., Charfeddine, M., Ammar, B., Hamed, B. B.,
Albalwy, F., Alqarafi, A., and Hussain, A. (2024b).
Unveiling machine learning strategies and considera-
tions in intrusion detection systems: a comprehensive
survey. Frontiers in Computer Science, 6:1387354.
Bharati, S., Mondal, M., Podder, P., and Prasath, V. (2022).
Federated learning: Applications, challenges and fu-
ture directions. International Journal of Hybrid Intel-
ligent Systems, 18(1-2):19–35.
Chen, T. and Guestrin, C. (2016). Xgboost: A scalable
tree boosting system. In Proceedings of the 22nd acm
sigkdd international conference on knowledge discov-
ery and data mining, pages 785–794.
Doriguzzi-Corin, R. and Siracusa, D. (2024). Flad: adaptive
federated learning for ddos attack detection. Comput-
ers & Security, 137:103597.
Fki, Z., Ammar, B., Fourati, R., Fendri, H., Hussain, A.,
and Ben Ayed, M. (2024). A novel iot-based deep
neural network for covid-19 detection using a soft-
attention mechanism. Multimedia Tools and Applica-
tions, 83(18):54989–55009.
Garg, S., Kaur, K., Batra, S., Kaddoum, G., Kumar, N., and
Boukerche, A. (2020). A multi-stage anomaly detec-
tion scheme for augmenting the security in iot-enabled
applications. Future Generation Computer Systems,
104:105–118.
Hdaib, M., Rajasegarar, S., and Pan, L. (2024). Quan-
tum deep learning-based anomaly detection for en-
hanced network security. Quantum Machine Intelli-
gence, 6(1):26.
Lakey, D. and Schlippe, T. (2024). A comparison of deep
learning architectures for spacecraft anomaly detec-
tion. In 2024 IEEE Aerospace Conference, pages 1–
11. IEEE.
Lyu, L., Yu, H., Ma, X., Chen, C., Sun, L., Zhao, J., Yang,
Q., and Philip, S. Y. (2022). Privacy and robustness in
federated learning: Attacks and defenses. IEEE trans-
actions on neural networks and learning systems.
Marfo, W., Tosh, D. K., and Moore, S. V. (2022). Net-
work anomaly detection using federated learning. In
MILCOM 2022-2022 IEEE Military Communications
Conference (MILCOM), pages 484–489. IEEE.
Moustafa, N. and Slay, J. (2015). Unsw-nb15: a compre-
hensive data set for network intrusion detection sys-
tems (unsw-nb15 network data set). In 2015 military
communications and information systems conference
(MilCIS), pages 1–6. IEEE.
Priyadarshini, I. (2024). Anomaly detection of iot cyberat-
tacks in smart cities using federated learning and split
learning. Big Data and Cognitive Computing, 8(3):21.
Rafique, S. H., Abdallah, A., Musa, N. S., and Murugan,
T. (2024). Machine learning and deep learning tech-
niques for internet of things network anomaly detec-
tion—current research trends. Sensors, 24(6):1968.
Saheb, M. C. P., Yadav, M. S., Babu, S., Pujari, J. J., and
Maddala, J. B. (2021). A review of ddos evaluation
dataset: Cicddos2019 dataset. In International Con-
ference on Energy Systems, Drives and Automations,
pages 389–397. Springer.
Sun, Y., Que, H., Cai, Q., Zhao, J., Li, J., Kong, Z., and
Wang, S. (2022). Borderline smote algorithm and
feature selection-based network anomalies detection
strategy. Energies, 15(13):4751.
Torabi, H., Mirtaheri, S. L., and Greco, S. (2023). Practical
autoencoder based anomaly detection by using vector
reconstruction error. Cybersecurity, 6(1):1.
Zhao, Y., Chen, J., Wu, D., Teng, J., and Yu, S. (2019).
Multi-task network anomaly detection using federated
learning. In Proceedings of the 10th international
symposium on information and communication tech-
nology, pages 273–279.
ENASE 2025 - 20th International Conference on Evaluation of Novel Approaches to Software Engineering
350