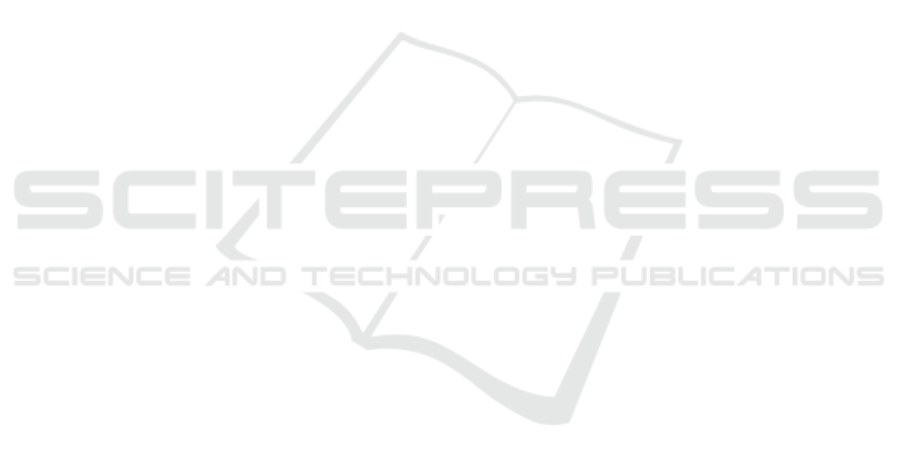
Breiman, L. (2001). Random forests. Machine learning,
45(1):5–32.
Bruen, D., Delaney, C., Florea, L., and Diamond, D. (2017).
Glucose sensing for diabetes monitoring: recent de-
velopments. Sensors, 17(8):1866.
Budiastutik, I., Kartasurya, M. I., Subagio, H. W., and Wid-
janarko, B. (2022). High prevalence of prediabetes
and associated risk factors in urban areas of pontianak,
indonesia: A cross-sectional study. Journal of Obe-
sity, 2022(1):4851044.
Cahn, A., Shoshan, A., Sagiv, T., Yesharim, R., Goshen,
R., Shalev, V., and Raz, I. (2020). Prediction
of progression from pre-diabetes to diabetes: de-
velopment and validation of a machine learning
model. Diabetes/Metabolism Research and Reviews,
36(2):e3252.
Chauhan, A. S., Varre, M. S., Izuora, K., Trabia, M. B., and
Dufek, J. S. (2023). Prediction of diabetes mellitus
progression using supervised machine learning. Sen-
sors, 23(10):4658.
Drucker, H., Burges, C. J., Kaufman, L., Smola, A., and
Vapnik, V. (1996). Support vector regression ma-
chines. Advances in neural information processing
systems, 9.
Echouffo-Tcheugui, J. B. and Selvin, E. (2021). Prediabetes
and what it means: the epidemiological evidence. An-
nual review of public health, 42(1):59–77.
Friedman, J. H. (2001). Greedy function approximation: A
gradient boosting machine. Annals of statistics, pages
1189–1232.
Haffner, S. M. (2003). Insulin resistance, inflammation, and
the prediabetic state. The American journal of cardi-
ology, 92(4):18–26.
JhaJay, C., ChowBryna, S., CooperMark, E., et al. (2016).
Diabetes and kidney disease: role of oxidative stress.
Antioxidants & redox signaling.
Katsarou, A., Gudbj
¨
ornsdottir, S., Rawshani, A., Dabelea,
D., Bonifacio, E., Anderson, B. J., Jacobsen, L. M.,
Schatz, D. A., and Lernmark,
˚
A. (2017). Type 1
diabetes mellitus. Nature reviews Disease primers,
3(1):1–17.
Klein, R. and Klein, B. E. (1995). Vision disorders in dia-
betes. Diabetes in America, 1:293.
Lee, J., Xue, X., Au, E., McIntyre, W. B., Asgariroozbe-
hani, R., Panganiban, K., Tseng, G. C., Papoulias,
M., Smith, E., Monteiro, J., et al. (2024). Glucose
dysregulation in antipsychotic-naive first-episode psy-
chosis: in silico exploration of gene expression signa-
tures. Translational Psychiatry, 14(1):19.
Liu, H., Li, Z., Che, S., Feng, Y., Guan, L., Yang, X., Zhao,
Y., Wang, J., Zvyagin, A. V., Yang, B., et al. (2022).
A smart hydrogel patch with high transparency, ad-
hesiveness and hemostasis for all-round treatment and
glucose monitoring of diabetic foot ulcers. Journal of
Materials Chemistry B, 10(30):5804–5817.
Liu, Y., Yang, L., and Cui, Y. (2024). A wear-
able, rapidly manufacturable, stability-enhancing mi-
croneedle patch for closed-loop diabetes management.
Microsystems & nanoengineering, 10(1):112.
Long, J., Yang, Z., Wang, L., Han, Y., Peng, C., Yan, C.,
and Yan, D. (2020). Metabolite biomarkers of type 2
diabetes mellitus and pre-diabetes: A systematic re-
view and meta-analysis. BMC endocrine disorders,
20:1–17.
Mansourian, M., Yazdani, A., Faghihimani, E., Aminor-
raya, A., Amini, M., and Jafari-Koshki, T. (2020).
Factors associated with progression to pre-diabetes:
a recurrent events analysis. Eating and Weight
Disorders-Studies on Anorexia, Bulimia and Obesity,
25:135–141.
Menke, A., Knowler, W. C., and Cowie, C. C. (2021). Phys-
ical and metabolic characteristics of persons with di-
abetes and prediabetes. Europepmc.
Mohamed, J., Nafizah, A. N., Zariyantey, A., and Budin, S.
(2016). Mechanisms of diabetes-induced liver dam-
age: the role of oxidative stress and inflammation. Sul-
tan qaboos university medical journal, 16(2):e132.
Mustafa, I. (2021). Diabetes prediction dataset.
https://www.kaggle.com/datasets/iammustafatz/
diabetes-prediction-dataset. Accessed: 2024-09-26.
Organization, W. H. (2023). Diabetes. Accessed: 28-Sep-
2024.
Perveen, S., Shahbaz, M., Keshavjee, K., and Guergachi,
A. (2019). Prognostic modeling and prevention of di-
abetes using machine learning technique. Scientific
reports, 9(1):13805.
Quinlan, J. R. (1986). Induction of decision trees. Machine
learning, 1(1):81–106.
Rumelhart, D. E., Hinton, G. E., and Williams, R. J. (1986).
Learning representations by back-propagating errors.
Nature, 323(6088):533–536.
Seber, G. A. F. and Lee, A. J. (2003). Linear Regression
Analysis. John Wiley & Sons.
Sokolova, M. and Lapalme, G. (2009). A systematic analy-
sis of performance measures for classification tasks.
Information Processing & Management, 45(4):427–
437.
Willmott, C. J. and Matsuura, K. (2005). Advantages of the
mean absolute error (mae) over the root mean square
error (rmse) in assessing average model performance.
Climate research, 30(1):79–82.
Zhang, J., Xu, J., Lim, J., Nolan, J. K., Lee, H., and
Lee, C. H. (2021). Wearable glucose monitor-
ing and implantable drug delivery systems for dia-
betes management. Advanced Healthcare Materials,
10(17):2100194.
Machine Learning-Driven Monitoring for Early Detection and Management of Prediabetes
263