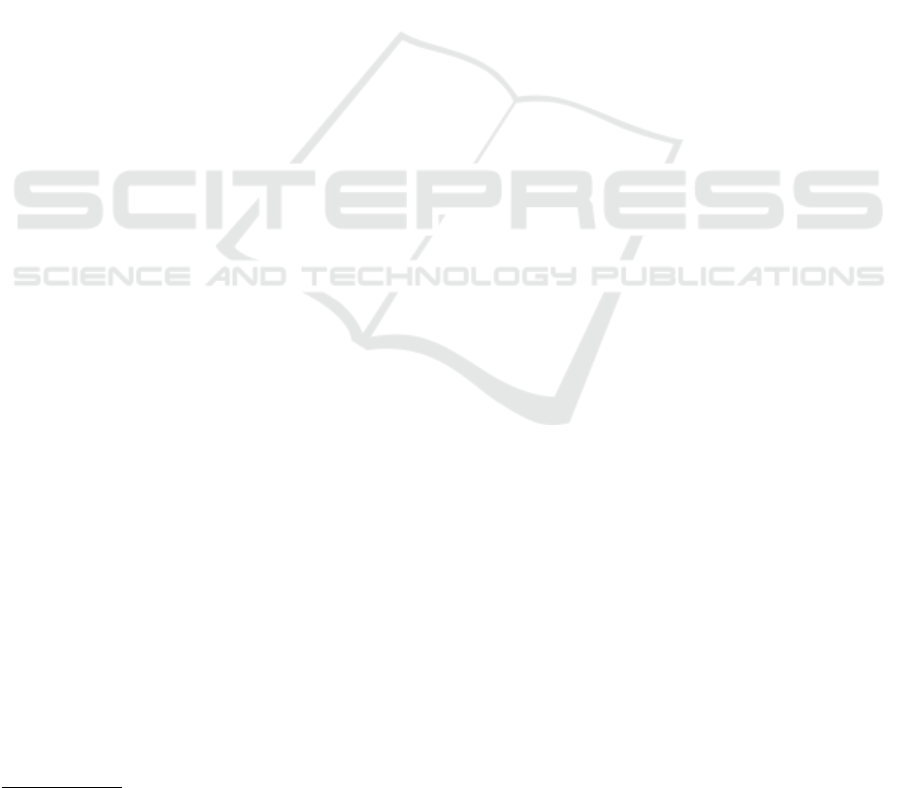
cations to advance XAI in industrial settings. It aims
to support practitioners and designers in developing
human-centered XAI for industrial applications.
ACKNOWLEDGMENTS
The present study is funded by VINNOVA Swe-
den (2021-04336), Bundesministerium f
¨
ur Bildung
und Forschung (BMBF; 01IS22030), and Rijksdienst
voor Ondernemend Nederland (AI2212001) under the
project Explanatory Artificial Interactive Intelligence
for Industry (EXPLAIN)
2
. We would like to thank
S
¨
odra Skogs
¨
agarna Ekonomisk F
¨
orening to support
the prototype development and user evaluation. We
also want to thank all user study participants.
REFERENCES
Ates, E., Aksar, B., Leung, V. J., and Coskun, A. K. (2021).
Counterfactual explanations for multivariate time se-
ries. In Proc. of ICAPAI, pages 1–8. IEEE.
Bertrand, A., Viard, T., Belloum, R., Eagan, J. R., and
Maxwell, W. (2023). On selective, mutable and dia-
logic XAI: A review of what users say about different
types of interactive explanations. In Proc. of CHI ’23.
ACM.
Brandt, E., Binder, T., and Sanders, E. B.-N. (2012). Tools
and techniques: Ways to engage telling, making and
enacting. In Routledge International Handbook of
Participatory Design, pages 145–181. Routledge.
Braun, V. and Clarke, V. (2012). Thematic analysis. In
APA Handbook of Research Methods in Psychology,
volume 2, pages 57–71. APA.
Chatzimparmpas, A., Kucher, K., and Kerren, A. (2024).
Visualization for trust in machine learning revisited:
The state of the field in 2023. IEEE CG&A, 44(3):99–
113.
Chatzimparmpas, A., Martins, R. M., Jusufi, I., Kucher,
K., Rossi, F., and Kerren, A. (2020). The state of
the art in enhancing trust in machine learning models
with the use of visualizations. Comp. Graph. Forum,
39(3):713–756.
Cheng, F., Ming, Y., and Qu, H. (2021). DECE: Decision
explorer with counterfactual explanations for machine
learning models. IEEE TVCG, 27(2):1438–1447.
Ciorna, V., Melanc¸on, G., Petry, F., and Ghoniem, M.
(2024). Interact: A visual what-if analysis tool for
virtual product design. Inf. Vis., 23(2):123–141.
Collaris, D., Weerts, H. J., Miedema, D., van Wijk, J. J.,
and Pechenizkiy, M. (2022). Characterizing data sci-
entists’ mental models of local feature importance. In
Proc. of NordiCHI. ACM.
2
https://explain-project.eu/ (last accessed: December 6,
2024)
Crispen, P. and Hoffman, R. R. (2016). How many experts?
IEEE Intell. Syst., 31(6):56–62.
De Rademaeker, E., Suter, G., Pasman, H. J., and Fabiano,
B. (2014). A review of the past, present and future
of the European loss prevention and safety promotion
in the process industries. Process Saf. Environ. Prot.,
92(4):280–291.
Duda, S., Warburton, C., and Black, N. (2020). Contextual
research: Why we need to research in context to de-
liver great products. In Human-Computer Interaction.
Design and User Experience, pages 33–49. Springer.
El-Assady, M. and Moruzzi, C. (2022). Which biases and
reasoning pitfalls do explanations trigger? Decom-
posing communication processes in human–AI inter-
action. IEEE CG&A, 42(6):11–23.
Fl
¨
uck, D. (2024). Coblis — Color blindness sim-
ulator. https://www.color-blindness.com/
coblis-color-blindness-simulator/. Last accessed:
September 5, 2024.
Forbes, M. G., Patwardhan, R. S., Hamadah, H., and
Gopaluni, R. B. (2015). Model predictive control
in industry: Challenges and opportunities. IFAC-
PapersOnLine, 48(8):531–538.
Friedman, J. H., Bentley, J. L., and Finkel, R. A. (1977).
An algorithm for finding best matches in logarithmic
expected time. ACM TOMS, 3(3):209–226.
Gomez, O., Holter, S., Yuan, J., and Bertini, E. (2020).
ViCE: Visual counterfactual explanations for machine
learning models. In Proc. of IUI, pages 531–535.
ACM.
Gomez, O., Holter, S., Yuan, J., and Bertini, E. (2021). Ad-
ViCE: Aggregated visual counterfactual explanations
for machine learning model validation. In Proc. of
VIS, pages 31–35. IEEE.
Grandi, F., Zanatto, D., Capaccioli, A., Napoletano, L.,
Cavallaro, S., and Peruzzini, M. (2024). A method-
ology to guide companies in using Explainable AI-
driven interfaces in manufacturing contexts. Procedia
Computer Science, 232:3112–3120.
Harrower, M. and Brewer, C. A. (2003). ColorBrewer.org:
An online tool for selecting colour schemes for maps.
Cartogr. J., 40(1):27–37.
Hartikainen, M., V
¨
a
¨
an
¨
anen, K., Lehti
¨
o, A., Ala-Luopa, S.,
and Olsson, T. (2022). Human-centered AI design in
reality: A study of developer companies’ practices. In
Proc. of NordiCHI. ACM.
Keane, M. T., Kenny, E. M., Delaney, E., and Smyth, B.
(2021). If only we had better counterfactual expla-
nations: Five key deficits to rectify in the evaluation
of counterfactual XAI techniques. In Proc. of IJCAI,
pages 4466–4474. IJCAI Organization.
Kotriwala, A., Kl
¨
opper, B., Dix, M., Gopalakrishnan, G.,
Ziobro, D., and Potschka, A. (2021). XAI for opera-
tions in the process industry — Applications, theses,
and research directions. In Proc. of AAAI-MAKE ’21.
CEUR Workshop Proceedings.
La Rosa, B., Blasilli, G., Bourqui, R., Auber, D., Santucci,
G., Capobianco, R., Bertini, E., Giot, R., and An-
gelini, M. (2023). State of the art of visual analytics
Designing Explainable and Counterfactual-Based AI Interfaces for Operators in Process Industries
841