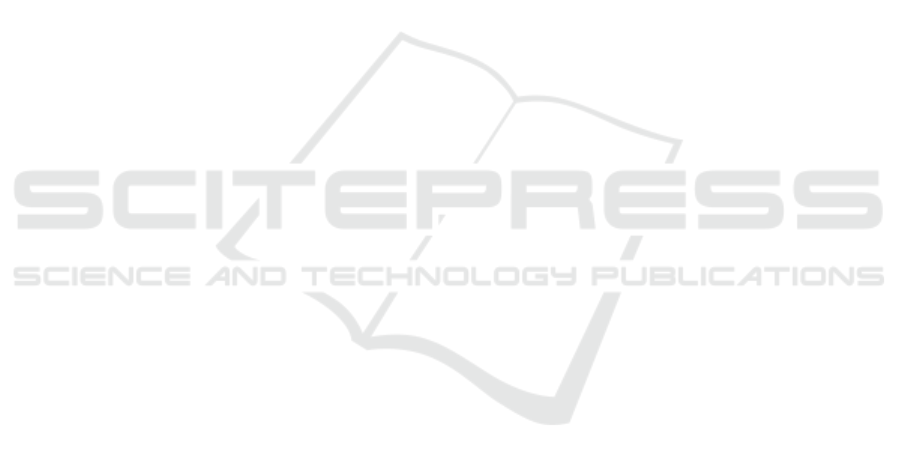
explored in this paper. The current automatic ap-
proaches, which are intended to find floret images
stemming from the same millefiori cane, allow for
a distinction between the different floret types. The
precision of the ordering in the computed image se-
quence needs further improvement. The visual ana-
lytics part of MillefioriAnalyzer allows for interactive
layout of the florets for visual analysis and retaining
the connection to the meta data at the same time. The
connection to the meta data has been lost in the ar-
chaeologists previous layout workflow.
As described throughout this paper, the Millefio-
riAnalyzer software and the archaeological analysis
are not yet complete. As usual in new digital human-
ities projects (J
¨
anicke, 2016), more iterations of de-
velopment and evaluation are needed. We will incor-
porate manually segmented images into our machine
learning part. These segmentations, provided by ar-
chaeological experts, contain the most significant pat-
terns of the florets an thus will positively influence the
minimization of the Siamese cost function. As a re-
sult, the calculated image similarities will be less con-
founded by damage, reflections and other noisy pat-
terns. In the future we will also acquire photographs
of higher quality and of more millefiori artefacts.
ACKNOWLEDGMENTS
We would like to thank T. Wendler, P. Kretler, M.
Kretler and S. Brender for their implementation sup-
port, and S. Lambert-Gates and S. Sarkar for support-
ing us in preparing the photographs and tracings.
REFERENCES
Aslan, S., Vascon, S., and Pelillo, M. (2020). Two sides
of the same coin: Improved ancient coin classification
using graph transduction games. Pattern Recognition
Letters, 131:158–165.
Ballard, D. H. (1987). Modular learning in neural networks.
In Proceedings of the sixth National conference on Ar-
tificial intelligence-Volume 1, pages 279–284.
Bickler, S. H. (2021). Machine learning arrives in archaeol-
ogy. Advances in Archaeolog. Practice, 9(2):186–191.
Brailsford, J. W. (1954). Guide to the antiquities of Roman
Britain. The Trustees of the British Museum.
Deligio, C., Tolle, K., and Wigg-Wolf, D. (2023). Support-
ing the analysis of a large coin hoard with AI-based
methods. In CAA2023 Conf. Proc. CAA, Zenodo.
Deufemia, V., Indelli Pisano, V., Paolino, L., and
de Roberto, P. (2014). A visual analytics system for
supporting rock art knowledge discovery. In Com-
putational Science and Its Applications–ICCSA 2014:
14th Internat. Conf., pages 466–480. Springer.
Exner, K. (1939). Die provinzialr
¨
omischen Emailfibeln der
Rheinlande. Bericht der r
¨
omisch-germanischen Kom-
mission, pages 31–121.
He, K., Zhang, X., Ren, S., and Sun, J. (2016). Deep resid-
ual learning for image recognition. In Proceedings of
the IEEE CVPR, pages 770–778.
Healey, C. and Enns, J. (2012). Attention and visual mem-
ory in visualization and computer graphics. IEEE
TVCG, 18(7):1170–1188.
Henry, P. (1933).
´
Emailleurs d’occident. Prehistoire II,
pages 66–146.
Inselberg, A. and Dimsdale, B. (1990). Parallel coordinates:
a tool for visualizing multi-dimensional geometry. In
Proceedings of the first IEEE conference on visualiza-
tion: visualization90, pages 361–378. IEEE.
J
¨
anicke, S. (2016). Valuable research for visualization and
digital humanities: A balancing act. In Worksh. on Vis.
for the Digital Humanities, IEEE VIS, volume 7.
Kingma, D. P. (2013). Auto-encoding variational bayes.
arXiv preprint arXiv:1312.6114.
Kiourt, C. and Evangelidis, V. (2021). Ancoins:
Image-based automated identification of ancient coins
through transfer learning approaches. In International
Conf. on Pattern Recognition, pages 54–67. Springer.
Koch, G., Zemel, R., Salakhutdinov, R., et al. (2015).
Siamese neural networks for one-shot image recog-
nition. In ICML deep learning workshop, volume 2,
pages 1–30. Lille.
Lee, J. E. (1862). Isca Silurum. Or an illustrated catalogue
of the Museum of Antiquities at Caerleon. Longman,
Green, Longmans & Roberts.
Li, J., Lai, C., Zhang, H., and Yuan, X. (2024). PM-Vis: A
visual analytics system for tracing and analyzing the
evolution of pottery motifs. IEEE TVCG, 30(6):3022–
3034.
Lidwell, W., Holden, K., and Butler, J. (2010). Universal
principles of design, revised and updated: 125 ways to
enhance usability, influence perception, increase ap-
peal, make better design decisions, and teach through
design. Rockport Pub.
Llobera, M. (2011). Archaeological visualization: towards
an archaeological information science (aisc). Journal
of Archaeological Method and Theory, 18:193–223.
Schlag, I. and Arandjelovic, O. (2017). Ancient roman
coin recognition in the wild using deep learning based
recognition of artistically depicted face profiles. In
Proceedings of the IEEE international conference on
computer vision workshops, pages 2898–2906.
Shneiderman, B. (2003). The eyes have it: A task by data
type taxonomy for information visualizations. In The
craft of inform. vis., pages 364–371. Elsevier.
Van der Maaten, L. and Hinton, G. (2008). Visualizing data
using t-sne. J. of machine learning research, 9(11).
van Helden, D., Mirkes, E., Tyukin, I., and Allison, P.
(2022). The arch-i-scan project: Artificial intelligence
and 3d simulation for developing new approaches to
roman foodways. J. of Comp. Applic. in Archaeology.
Wong, P. C. and Thomas, J. (2004). Visual analytics. IEEE
Computer Graphics and Applications, 24(5):20–21.
IVAPP 2025 - 16th International Conference on Information Visualization Theory and Applications
814