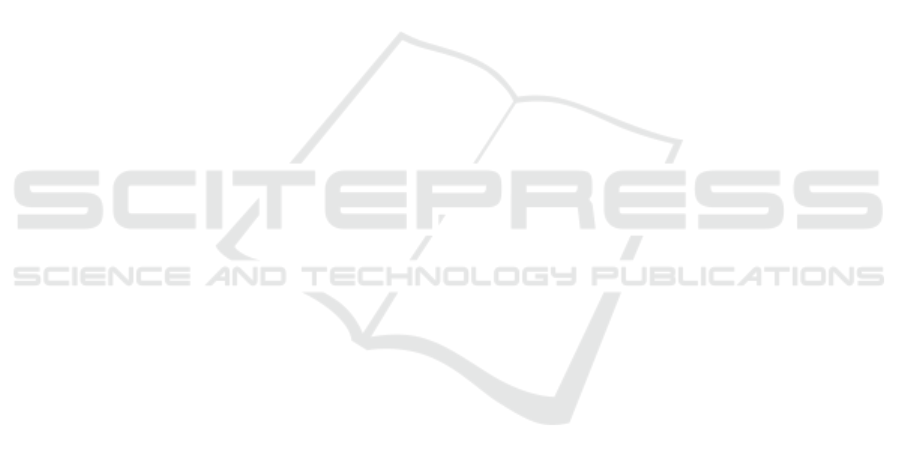
reward related to the relationships between facilities is
only partially calculated during facility placement ac-
tions. This suggests that the relationships between fa-
cilities do not significantly contribute to the reinforce-
ment learning objective of maximizing profit. There-
fore, since the reward in equation (5) improved the
success rate of layout generation, we plan to examine
whether applying a similar reward to the relationships
between facilities can further reduce the DI analysis
evaluation values. For example, a method that im-
poses negative rewards on the number of relationships
between facilities with distances exceeding a certain
value at the end of an episode, thereby encouraging
the placement of facilities considering their relation-
ships to ensure that the distances between facilities
fall within a certain range, can be mentioned.
6.2.2 Improvement of MLSH
Not limited to MLSH, hierarchical reinforcement
learning, which divides the target problem into multi-
ple sub-tasks for learning, is said to contribute to re-
ducing the exploration space during learning due to its
structure of having multiple sub-policies. However,
it has been pointed out that methods that automati-
cally acquire such sub-policies may result in all sub-
policies converging to the same policy, thereby losing
diversity among them. In response to this, Huo et al.
proposed a method that updates MLSH sub-policies
to differentiate them from each other using similar-
ity measures of probability distributions, such as KL
divergence, thereby effectively utilizing the multi-
ple sub-policies (Huo et al., 2023). Experiments on
various tasks have shown that this method increases
the rewards compared to conventional MLSH. In this
study, we aim to introduce such methods that lever-
age the structural advantages of MLSH to optimize
the learning of facility relationships.
REFERENCES
Arulkumaran, K., Deisenroth, M. P., Brundage, M., and
Bharath, A. A. (2017). Deep Reinforcement Learn-
ing: A Brief Survey. IEEE Signal Processing Maga-
zine, 34(6):26–38.
Di, X. and Yu, P. (2021a). Deep Reinforcement Learning
for Producing Furniture Layout in Indoor Scenes.
Di, X. and Yu, P. (2021b). Multi-Agent Reinforcement
Learning of 3D Furniture Layout Simulation in Indoor
Graphics Scenes. CoRR, abs/2102.09137.
Dietterich, T. G. (2000). Hierarchical Reinforcement Learn-
ing with the MAXQ Value Function Decomposition.
Journal of Artificial Intelligence Research, 13:227–
303.
Drira, A., Pierreval, H., and Hajri-Gabouj, S. (2007). Fa-
cility layout problems: A survey. Annual Reviews in
Control, 31(2):255–267.
Frans, K., Ho, J., Chen, X., Abbeel, P., and Schulman, J.
(2017). Meta Learning Shared Hierarchies.
Huo, L., Wang, Z., Xu, M., and Song, Y. (2023). A Task-
Agnostic Regularizer for Diverse Subpolicy Discov-
ery in Hierarchical Reinforcement Learning. IEEE
Transactions on Systems, Man, and Cybernetics: Sys-
tems, 53(3):1932–1944.
Husoon, O. O., Kadhim, D. A., and Raheem, K. M. H.
(2022). Reconfigration of manufacturing facility lay-
out using meta heuristic particle swarm optimization.
AIP Conference Proceedings, 2386(1):050013.
Ikeda., H., Nakagawa., H., and Tsuchiya., T. (2023). Au-
tomatic Facility Layout Design System Using Deep
Reinforcement Learning. In Proceedings of the 15th
International Conference on Agents and Artificial In-
telligence - Volume 2: ICAART, pages 221–230. IN-
STICC, SciTePress.
Kaelbling, L. P., Littman, M. L., and Moore, A. W. (1996).
Reinforcement learning: a survey. J. Artif. Int. Res.,
4(1):237–285.
Kar Yan Tam (1992). Genetic algorithms, function opti-
mization, and facility layout design. European Jour-
nal of Operational Research, 63(2):322–346. Strate-
gic Planning of Facilities.
Meller, R. D. and Bozer, Y. A. (1997). Alternative
Approaches to Solve the Multi-Floor Facility Lay-
out Problem. Journal of Manufacturing Systems,
16(6):457–458.
Paes, F. G., Pessoa, A. A., and Vidal, T. (2017). A hy-
brid genetic algorithm with decomposition phases for
the Unequal Area Facility Layout Problem. European
Journal of Operational Research, 256(3):742–756.
Ripon, K. S. N., Glette, K., Høvin, M., and Torresen, J.
(2010). A Genetic Algorithm to Find Pareto-optimal
Solutions for the Dynamic Facility Layout Problem
with Multiple Objectives. In Wong, K. W., Mendis, B.
S. U., and Bouzerdoum, A., editors, Neural Informa-
tion Processing. Theory and Algorithms, pages 642–
651, Berlin, Heidelberg. Springer Berlin Heidelberg.
Saaty, T. L. (1980). The analytic hierarchy process (AHP).
The Journal of the Operational Research Society,
41(11):1073–1076.
Sutton, R. S., Precup, D., and Singh, S. (1999). Between
MDPs and semi-MDPs: A framework for temporal
abstraction in reinforcement learning. Artificial Intel-
ligence, 112(1):181–211.
Facility Layout Generation Using Hierarchical Reinforcement Learning
157