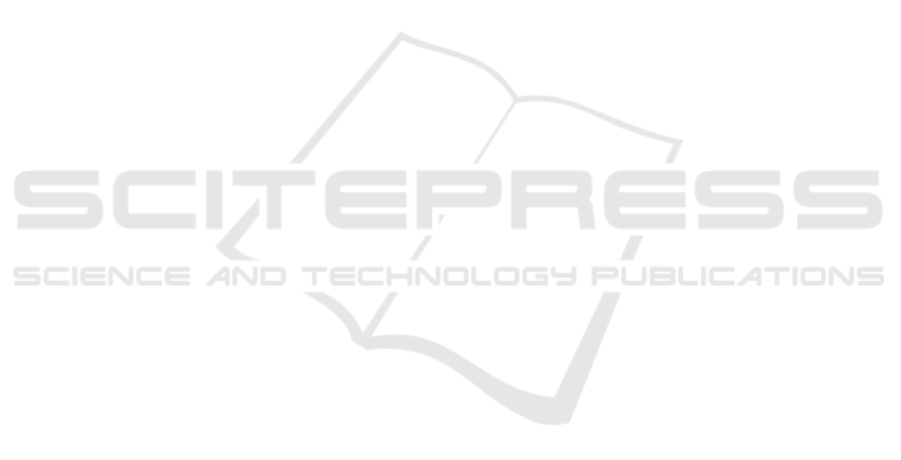
the CIFAR-10 and STL-10 datasets, with the largest
margin of improvement being obtained on the STL-
10 dataset. On CIFAR-100 our method achieves
error rates that are comparable to the results re-
ported in the literature in the 10000-label regime. On
the CIFAR-100 dataset with 2500 labeled samples,
Diff-SySC has a higher error rate than the best litera-
ture approach, CRMatch, but it still is able to outper-
form other methods, such as the Π-Model, Pseudo-
labeling, Mean Teacher and MixMatch. The results
obtained on CIFAR-100 could be due to the larger
number of categories in this dataset and the shared
similarities between classes that belong to the same
super-class. This leads to a more complex label dis-
tribution that the model needs to learn.
To summarize, on CIFAR-100, considering
both datasets (with 2500 and 10000 labels), our
Diff-SySC approach outperforms the related work
depicted in Table 3 in 72.2% of the cases (13 out of
18 comparisons). Overall, considering all datasets
and experiments, a better performance is observed
for Diff-SySC in 90.74% of the cases (49 out of 54
comparisons). We also note small standard devia-
tions of the error rates achieved by our proposed semi-
supervised diffusion-based architecture, thus empha-
sizing the stability and robustness of Diff-SySC.
Figure 3 gives insights into the training dynamics
by showing the accuracy obtained during the train-
ing iterations and the proportions of labeled and un-
labeled data, as progressively more annotations (real
and generated labels) are used for training the model.
The top figure shows the train and test set accuracy
of the model after each of the training iterations.
Additionally, the proportion of correctly generated
pseudo-labels is depicted in the case of CIFAR-10
and CIFAR-100. This metric is omitted in the case
of STL-10 due to the fact the ground truth labels are
not available for the unlabeled data. Figure 3 high-
lights that the largest number of annotations is gen-
erated at the end of the first iterations, with a good
accuracy (over 90% of the pseudo-labels generated
after the first iteration are correct), while fewer sam-
ples are annotated during subsequent iterations. Even
though the accuracy of the pseudo-labeling procedure
decreases over the iterations, as it becomes more dif-
ficult to annotate new samples, the test set accuracy
is not affected. This highlights the robustness of our
approach to the presence of noisy pseudo-labels.
Additionally, we analyze how the training con-
vergence is reflected within the pseudo-annotation of
the unlabeled dataset. For the CIFAR-10 with 250
labels and STL-10 with 250 labels, only a few un-
labeled samples have not been confidently pseudo-
labeled throughout the training process. Meanwhile,
on CIFAR-100 with 10000 labels, the training does
not conclude with a complete coverage of the unla-
beled dataset. This phenomenon can be attributed to
the higher complexity of the data involved and the
observed overfitting accumulated throughout the it-
erations, as shown on the top row. Nonetheless, the
confident pseudo-labels are predominantly accurate,
with 97.96% aggregated pseudo-labels accuracy on
CIFAR-10 and 87.05% on CIFAR-100.
A potential limitation of our method is the depen-
dence on a pre-trained feature encoder for training the
diffusion model. While general-purpose models like
CLIP can be effective in most cases, other tasks that
involve images sampled from a very different distri-
bution (e.g., medical images, radar or satellite data),
may require more specialized encoders. Nevertheless,
our framework is flexible enough to allow the inte-
gration of any type of feature extractor trained in an
unsupervised manner on the unlabeled data. A sec-
ond limitation is represented by the fact that the unla-
beled data is not used directly during training until it
is pseudo-annotated with confident predictions. This
could constitute a drawback in scenarios with very
few labels per class, as the initial model, D
0
, may not
have enough information to be effectively trained. A
possible strategy to alleviate this issue is to integrate
unsupervised objective functions in the training of the
LRA-Diffusion model.
6 CONCLUSIONS
In this work, we introduced a diffusion-based ap-
proach for semi-supervised learning, Diff-SySC. The
method was evaluated on three image benchmarks:
CIFAR-10, CIFAR-100 and STL-10, with varying
ratios of labeled data. The research questions for-
mulated in Section 1 have been answered. RQ1
was answered by introducing the multi-stage semi-
supervised learning approach Diff-SySC which uses
a diffusion model for label generation, unlike the ex-
isting literature approaches that use diffusion mod-
els for enhancing the training dataset. For answer-
ing RQ2, Diff-SySC was compared with multiple
related work methods covering diverse methodolo-
gies and strategies for semi-supervised learning. The
conducted comparison highlighted a performance im-
provement achieved by Diff-SySC over the related
work in 90.74% of the cases. In addition, the robust-
ness and stability of Diff-SySC has been emphasized
through small standard deviations of the error rates
achieved by our model over multiple runs.
Future work will investigate extensions of our
method that integrate unsupervised loss functions,
ICAART 2025 - 17th International Conference on Agents and Artificial Intelligence
138