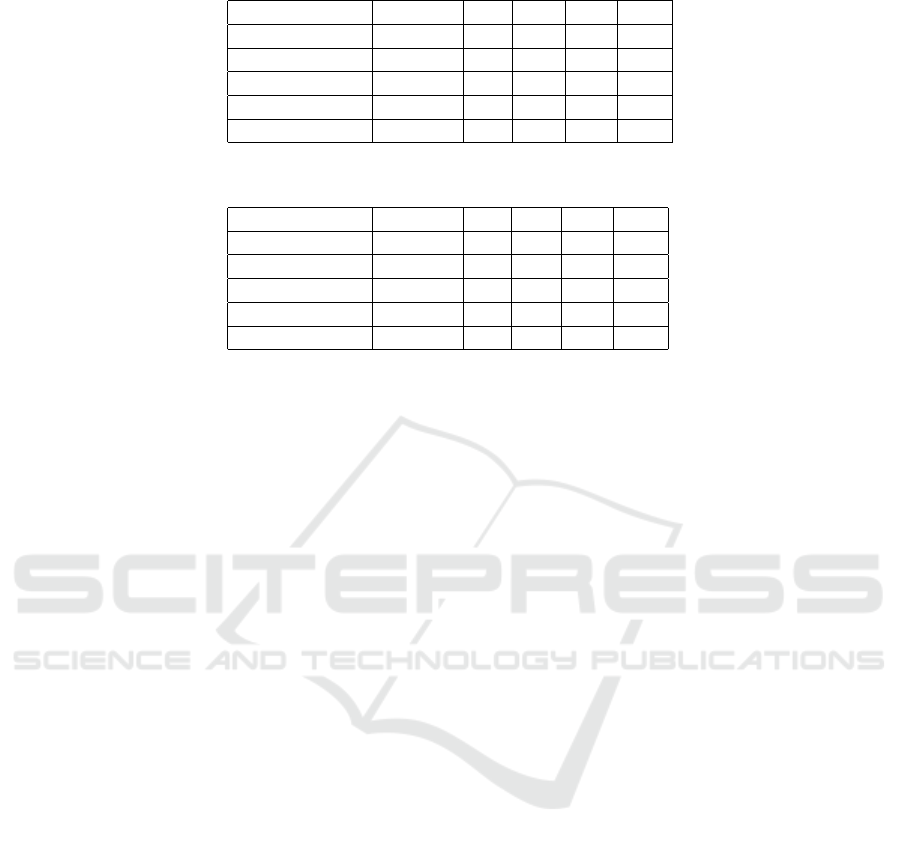
Table 1: Dynamic Workload management via the proposed methodology for allotting edge devices to mobile users. ES:Edge
Server,LT:Latency (ms),TP:Throughput (Mbps), RU:Resource Utilization (%),SW:Social Welfare
Mobile Device ES LT TP RU SW
Device 1 Server 1 50 100 70 850
Device 2 Server 2 60 95 65 820
Device 3 Server 3 45 105 75 870
Device 4 Server 1 55 98 80 880
Device 5 Server 2 52 102 72 840
Table 2: Bursty traffic management via the proposed methodology for allotting edge devices to mobile users. ES:Edge
Server,LT:Latency (ms),TP:Throughput (Mbps), RU:Resource Utilization (%),SW:Social Welfare
Mobile Device ES LT TP RU SW
Device 1 Server 1 60 80 60 700
Device 2 Server 2 70 75 55 680
Device 3 Server 3 55 85 65 720
Device 4 Server 1 65 78 70 730
Device 5 Server 2 62 82 62 710
ing dynamic workloads utilizing both the proposed
methodology and the round-robin procedure. Sim-
ilarly, in Tables 6 and 7, we supply these metrics
for managing bursty traffic utilizing the suggested
methodology and round-robin procedure. Eventu-
ally, in Table 8 and 9, the same investigation is
performed for handling network congestion utilizing
both methodologies.
Visualizing the tabular data, fig. 2 demonstrates
the effectiveness of the suggested methodology in
comparison to the round-robin technique in lower-
ing latency across three domains: handling dynamic
workloads, handling bursty traffic, and network con-
gestion. Fig. 3 indicates the usefulness of the
suggested procedure in improving throughput cor-
responding to the round-robin technique across the
same domains. Similarly, Fig. 4 showcases the
progress in resource utilization accomplished by the
suggested methodology over round-robin across these
domains. Lastly, Fig. 5 portrays the enhancement in
social welfare, directing to better customer satisfac-
tion, attained by the presented methodology over the
round-robin procedure across the same domains.
7 CONCLUSION AND FUTURE
WORKS
This research paper presents a newly integrated re-
source allotment approach for Mobile Edge Com-
puting (MEC) utilizing Multi-Armed Bandit (MAB)
and Reinforcement Learning (RL). By presenting re-
source allotment as an MAB problem and employing
the Upper Confidence Bound (UCB) algorithm, it al-
lows adaptive allotment of computational resources
in real-time, improving performance and minimiz-
ing latency. The investigation exhibits the signifi-
cance of the procedure in dynamically determining
edge servers founded on real-time feedback, exceed-
ing static allotment strategies. Future research av-
enues comprise upgrading dynamic MEC models, in-
vestigating multi-objective improvements, and han-
dling security issues. Real-world integration and part-
nership with industry stakeholders are crucial for val-
idation and implementation. Overall, the research do-
nates to growing MEC systems for latency-prone util-
ities in the 5G era and beyond.
ACKNOWLEDGMENT
We wholeheartedly thank the Department of Com-
puter Science Engineering of Kalinga Institute of In-
dustrial Technology for giving us this opportunity
to work in this cutting-edge field. Furthermore, we
thank our esteemed professors who constantly sup-
ported us throughout this research study by reviewing
our work with productive feedback and suggestions to
improve further.
REFERENCES
Ateya, A. A., Muthanna, A., Koucheryavy, A., Maleh, Y.,
and El-Latif, A. A. A. (2023). Energy efficient of-
floading scheme for mec-based augmented reality sys-
tem. Cluster Computing, 26(1):789–806.
Chi, H. R., Silva, R., Santos, D., Quevedo, J., Corujo, D.,
Abboud, O., Radwan, A., Hecker, A., and Aguiar,
R. L. (2023). Multi-criteria dynamic service migration
IC3Com 2024 - International Conference on Cognitive & Cloud Computing
112