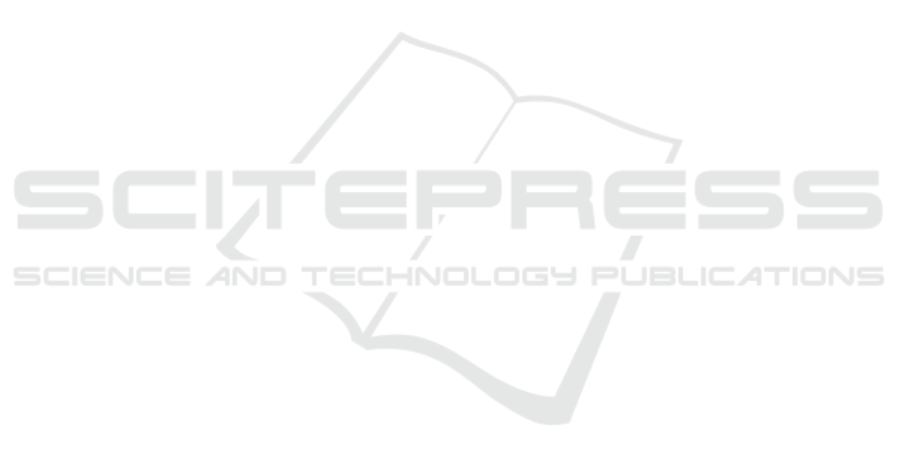
Kim, S. N. and Lee, H. (2022). Capturing reality: Valida-
tion of omnidirectional video-based immersive virtual
reality as a streetscape quality auditing method. Land-
scape and Urban Planning, 218. Publisher: Elsevier
B.V.
Li, Y., Yabuki, N., and Fukuda, T. (2022). Measuring
visual walkability perception using panoramic street
view images, virtual reality, and deep learning. Sus-
tainable Cities and Society, 86.
Li, Y., Yabuki, N., Fukuda, T., and Zhang, J. (2020). A
big data evaluation of urban street walkability using
deep learning and environmental sensors a case study
around Osaka University Suita campus.
Liao, B., van den Berg, P. E., van Wesemael, P., and Ar-
entze, T. A. (2022). Individuals’ perception of walk-
ability: Results of a conjoint experiment using videos
of virtual environments. Cities, 125:103650. Pub-
lisher: Elsevier Ltd.
Ma, X., Ma, C., Wu, C., Xi, Y., Yang, R., Peng, N., Zhang,
C., and Ren, F. (2021). Measuring human perceptions
of streetscapes to better inform urban renewal: A per-
spective of scene semantic parsing. Cities, 110. Pub-
lisher: Elsevier Ltd.
Makransky, G., Lilleholt, L., and Aaby, A. (2017). Develop-
ment and validation of the Multimodal Presence Scale
for virtual reality environments: A confirmatory factor
analysis and item response theory approach. Comput-
ers in Human Behavior, 72:276–285.
Martinez, E. S., Wu, A. S., and McMahan, R. P. (2022).
Research Trends in Virtual Reality Locomotion Tech-
niques. In 2022 IEEE Conference on Virtual Reality
and 3D User Interfaces (VR), pages 270–280. ISSN:
2642-5254.
Morris, J. N. and Hardman, A. E. (1997). Walking to
Health. Sports Medicine, 23(5):306–332.
Nagata, S., Nakaya, T., Hanibuchi, T., Amagasa, S.,
Kikuchi, H., and Inoue, S. (2020). Objective scor-
ing of streetscape walkability related to leisure walk-
ing: Statistical modeling approach with semantic seg-
mentation of Google Street View images. Health and
Place, 66.
Nakamura, K. (2021). Experimental analysis of walkabil-
ity evaluation using virtual reality application. Envi-
ronment and Planning B: Urban Analytics and City
Science, 48(8):2481–2496. Publisher: SAGE Publi-
cations Ltd.
Pak, B. and Ag-ukrikul, C. (2017). Participatory Evaluation
of the Walkability of two Neighborhoods in Brussels
- Human Sensors versus Space Syntax. pages 553–
560. Conference Name: eCAADe 2017 : ShoCK!
– Sharing of Computable Knowledge! Place: Rome,
Italy.
Papoutsaki, A., Laskey, J., and Huang, J. (2017).
SearchGazer: Webcam Eye Tracking for Remote
Studies of Web Search. In Proceedings of the ACM
SIGIR Conference on Human Information Interaction
& Retrieval (CHIIR). ACM.
Raswol, L. M. (2020). Qualitative Assessment for Walk-
ability: Duhok University Campus as a Case Study.
IOP Conference Series: Materials Science and Engi-
neering, 978(1):012001. Publisher: IOP Publishing.
Razzaque, S., Kohn, Z., and Whitton, M. C. (2001). Redi-
rected Walking. Accepted: 2015-11-11T18:52:51Z
Publisher: Eurographics Association.
Salazar Miranda, A., Fan, Z., Duarte, F., and Ratti, C.
(2021). Desirable streets: Using deviations in pedes-
trian trajectories to measure the value of the built en-
vironment. Computers, Environment and Urban Sys-
tems, 86:101563.
Silvennoinen, H., Kuliga, S., Herthogs, P., Recchia, D. R.,
and Tunc¸er, B. (2022). Effects of Gehl’s urban design
guidelines on walkability: A virtual reality experiment
in Singaporean public housing estates. Environment
and Planning B: Urban Analytics and City Science,
49(9):2409–2428. Publisher: SAGE Publications Ltd
STM.
Stokes, D. and Biggs, S. (2014). The Dominance of the
Visual. In Stokes, D., Matthen, M., and Biggs, S.,
editors, Perception and Its Modalities, page 0. Oxford
University Press.
Talen, E. and Koschinsky, J. (2013). The Walkable Neigh-
borhood: A Literature Review. International Journal
of Sustainable Land Use and Urban Planning, 1.
Thibaud, J.-P. (2013). Commented City Walks. Wi: Journal
of Mobile Culture, vol.7(n°1):pp.1–32.
Tudor-Locke, C., Craig, C. L., Brown, W. J., Clemes, S. A.,
De Cocker, K., Giles-Corti, B., Hatano, Y., Inoue,
S., Matsudo, S. M., Mutrie, N., Oppert, J.-M., Rowe,
D. A., Schmidt, M. D., Schofield, G. M., Spence, J. C.,
Teixeira, P. J., Tully, M. A., and Blair, S. N. (2011).
How many steps/day are enough? for adults. Interna-
tional Journal of Behavioral Nutrition and Physical
Activity, 8(1):79.
White, M. P., Alcock, I., Grellier, J., Wheeler, B. W., Har-
tig, T., Warber, S. L., Bone, A., Depledge, M. H., and
Fleming, L. E. (2019). Spending at least 120 minutes
a week in nature is associated with good health and
wellbeing. Scientific Reports, 9(1):7730. Number: 1
Publisher: Nature Publishing Group.
Xu, X., Qiu, W., Li, W., Liu, X., Zhang, Z., Li, X., and Luo,
D. (2022). Associations Between Street-View Per-
ceptions and Housing Prices: Subjective vs. Objec-
tive Measures Using Computer Vision and Machine
Learning Techniques. Remote Sensing, 14(4). Pub-
lisher: MDPI.
Zhang, R. X. and Zhang, L. M. (2021). Panoramic visual
perception and identification of architectural cityscape
elements in a virtual-reality environment. Future Gen-
eration Computer Systems, 118:107–117. Publisher:
Elsevier B.V.
Zhang, Y., Zou, Y., Zhu, Z., Guo, X., and Feng, X.
(2022). Evaluating Pedestrian Environment Using
DeepLab Models Based on Street Walkability in
Small and Medium-Sized Cities: Case Study in Gaop-
ing, China. Sustainability (Switzerland), 14(22). Pub-
lisher: MDPI.
Zhou, H., He, S., Cai, Y., Wang, M., and Su, S. (2019). So-
cial inequalities in neighborhood visual walkability:
Using street view imagery and deep learning technolo-
gies to facilitate healthy city planning. Sustainable
Cities and Society, 50. Publisher: Elsevier Ltd.
GISTAM 2024 - 10th International Conference on Geographical Information Systems Theory, Applications and Management
210