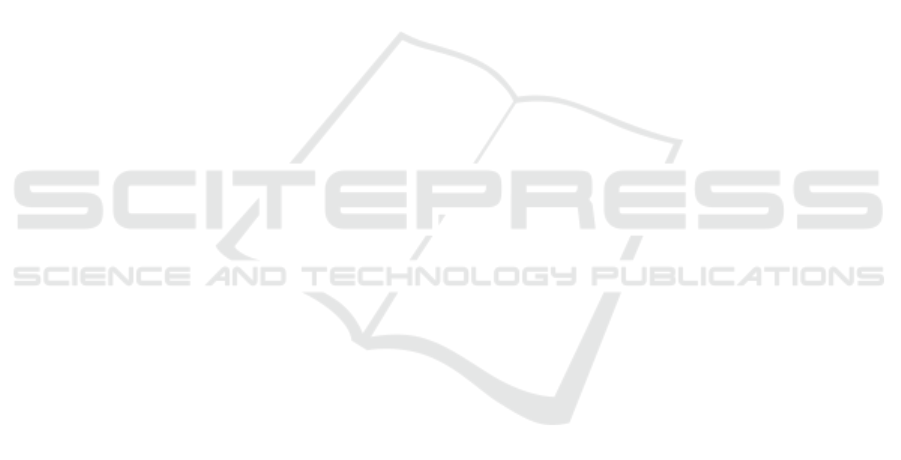
line which represents a reduction of more than 150
tons per line and per year. Compare to references in
the literature (Stewart and El-Geneidy, 2016) explain
that bus stop consolidation is effective and simple to
the quality of service, and conclude that remove aver-
age 23% bus stops and reducing the system coverage
area by 1%. In addition (Kehoe, 2004) show that bus
stop consolidation improve timetable reliability and
has no negative impact on ridership, and can even im-
prove it.
This study is an initial effort to examine the feasi-
bility of solutions to reduce the carbon impact on the
environment, while also assessing the impact on qual-
ity of service. Our model is not perfect and needs to
be modified to include more factors in the quantifica-
tion of greenhouse gas emissions. However, we also
need to assess the influences and trends on the choice
of mode of transport when there is a modal shift.
ACKNOWLEDGEMENTS
All the mentioned authors performed the contribu-
tions to the present paper. This work was carried
out within the framework of a scientific collabora-
tion agreement with Tiss
´
eo Collectivit
´
es. The authors
thank Tiss
´
eo Collectivit
´
es for having sharing the used
data and their support.
REFERENCES
ADEME (2023). Share of public transport in total passenger
traffic. https://www.odyssee-mure.eu/publications/
efficiency-by-sector/transport/public-transport.html.
Consulted on 2024-01-08.
Alsger, A., Assemi, B., Mesbah, M., and Ferreira, L.
(2016). Validating and improving public transport ori-
gin–destination estimation algorithm using smart card
fare data. Transportation Research Part C: Emerging
Technologies, 68:490–506.
Boulange, C., Gunn, L., Giles-Corti, B., Mavoa, S., Pet-
tit, C., and Badland, H. (2017). Examining associ-
ations between urban design attributes and transport
mode choice for walking, cycling, public transport
and private motor vehicle trips. Journal of transport
& health, 6:155–166.
Cui, A. (2006). Bus passenger origin-destination matrix
estimation using automated data collection systems.
PhD Thesis, Massachusetts Institute of Technology.
Daraio, C., Diana, M., Di Costa, F., Leporelli, C., Mat-
teucci, G., and Nastasi, A. (2016). Efficiency and ef-
fectiveness in the urban public transport sector: A crit-
ical review with directions for future research. Euro-
pean Journal of Operational Research, 248(1):1–20.
Dickens, M. (2023). Public transportation fact book.
https://www.apta.com/wp-content/uploads/APTA-
2022-Public-Transportation-Fact-Book.pdf. Con-
sulted on 2024-01-08.
El Mahrsi, M., C
ˆ
ome, E., Baro, J., and Oukhellou, L.
(2014). Understanding Passenger Patterns in Pub-
lic Transit Through Smart Card and Socioeconomic
Data: A case study in Rennes, France.
Feng, J., Li, Y., Zhang, C., Sun, F., Meng, F., Guo, A., and
Jin, D. (2018). DeepMove: Predicting Human Mo-
bility with Attentional Recurrent Networks. In Pro-
ceedings of the 2018 World Wide Web Conference,
WWW ’18, pages 1459–1468, Republic and Can-
ton of Geneva, CHE. International World Wide Web
Conferences Steering Committee. event-place: Lyon,
France.
Ge, Q. and Fukuda, D. (2016). Updating origin–destination
matrices with aggregated data of GPS traces. Trans-
portation Research Part C: Emerging Technologies,
69:291–312.
Hora, J., Dias, T. G., Camanho, A., and Sobral, T. (2017).
Estimation of Origin-Destination matrices under Au-
tomatic Fare Collection: the case study of Porto trans-
portation system. Transportation Research Procedia,
27:664–671.
Hrelja, R., Khan, J., and Pettersson, F. (2020). How to cre-
ate efficient public transport systems? a systematic re-
view of critical problems and approaches for address-
ing the problems. Transport Policy, 98:186–196.
Huang, D., Yu, J., Shen, S., Li, Z., Zhao, L., and Gong,
C. (2020). A Method for Bus OD Matrix Estimation
Using Multisource Data. Journal of Advanced Trans-
portation, 2020:5740521.
Hussain, E., Bhaskar, A., and Chung, E. (2021). Transit od
matrix estimation using smartcard data: Recent de-
velopments and future research challenges. Trans-
portation Research Part C: Emerging Technologies,
125:103044.
INFRAS (2023). Hbefa. https://www.hbefa.net/en/
software#online-version. Consulted on 2024-01-08.
Ji, Y., Cao, Y., Liu, Y., Guo, W., and Gao, L. (2019).
Research on classification and influencing factors of
metro commuting patterns by combining smart card
data and household travel survey data. IET Intelligent
Transport Systems, 13(10):1525–1532.
Kehoe, O. d. (2004). Effects of bus stop consolidation on
transit speed and reliability: A test case. Master of
Science), University of Washington.
Li, W. (2016). Modelling bike-sharing choice in a develop-
ing country with a focus on the impacts of air pollu-
tion and weather conditions. In General Proceedings
of the 14th World Conference on Transport Research,
volume 14. World Conference on Transport Research
Society (WCTRS).
MinisterForEcology (2019). Se d
´
eplacer en
voiture. https://www.statistiques.developpement-
durable.gouv.fr/se-deplacer-en-voiture-seul-
plusieurs-ou-en-covoiturage-0. Consulted on
2024-01-08.
VEHITS 2024 - 10th International Conference on Vehicle Technology and Intelligent Transport Systems
376