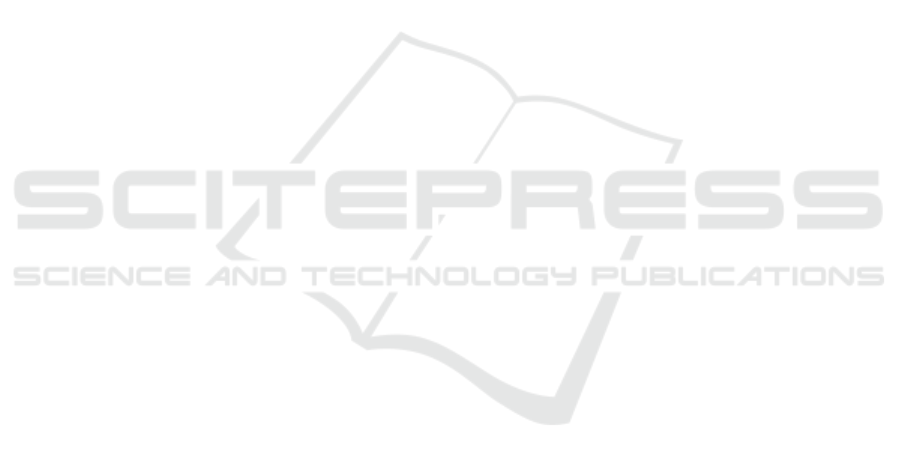
ture. JPEN. Journal of parenteral and enteral nutri-
tion, 38.
Geurts, S. A. and Sonnentag, S. (2006). Recovery as
an explanatory mechanism in the relation between
acute stress reactions and chronic health impairment.
Scandinavian journal of work, environment & health,
pages 482–492.
Goggins, R. W., Spielholz, P., and Nothstein, G. L. (2008).
Estimating the effectiveness of ergonomics interven-
tions through case studies: Implications for predic-
tive cost-benefit analysis. Journal of Safety Research,
39(3):339–344.
Hammer, J. and Newth, C. (2009). Assessment of thoraco-
abdominal asynchrony. Paediatric Respiratory Re-
views, 10(2):75 –80.
He, H., Tan, Y., and Wang, Y. (2015). Optimal base wavelet
selection for ECG noise reduction using a comprehen-
sive entropy criterion. Entropy, 17(9):6093–6109.
Hess-nielsen, N. and Wickerhauser, M. (1996). Wavelets
and time-frequency analysis. Proceedings of the
IEEE, 84:523–540.
Holtermann, A., Hansen, J. V., Burr, H., Søgaard, K., and
Sjøgaard, G. (2012). The health paradox of occupa-
tional and leisure-time physical activity. British Jour-
nal of Sports Medicine, 46(4):291–295.
Holtermann, A., Krause, N., van der Beek, A. J., and
Straker, L. (2018). The physical activity paradox:
six reasons why occupational physical activity (OPA)
does not confer the cardiovascular health benefits that
leisure time physical activity does. British Journal of
Sports Medicine, 52(3):149–150.
Kazmierczak, K., Mathiassen, S. E., Forsman, M.,
and Winkel, J. (2005). An integrated analysis
of ergonomics and time consumption in Swedish
‘craft-type’ car disassembly. Applied Ergonomics,
36(3):263–273.
Krause, N., Arah, O. A., and Kauhanen, J. (2017). Physi-
cal activity and 22-year all-cause and coronary heart
disease mortality. American Journal of Industrial
Medicine, 60(11):976–990.
Krause, N., Brand, R. J., Kaplan, G. A., Kauhanen,
J., Malla, S., Tuomainen, T.-P., and Salonen, J. T.
(2007). Occupational physical activity, energy expen-
diture and 11-year progression of carotid atheroscle-
rosis. Scandinavian Journal of Work, Environment &
Health, (6):405–424.
Lester, J., Hannaford, B., and Borriello, G. (2004). “Are
You with Me?” – Using Accelerometers to Determine
If Two Devices Are Carried by the Same Person. vol-
ume 3001, pages 33–50.
Liu, S., Gao, R. X., John, D., Staudenmayer, J., and Freed-
son, P. (2013). Tissue Artifact Removal from Respi-
ratory Signals Based on Empirical Mode Decompo-
sition. Annals of biomedical engineering, 41:1003–
1015.
Lundberg, U., Granqvist, M., Hanssonand, T., Magnusson,
M., and Wallin, L. (1989). Psychological and physi-
ological stress responses during repetitive work at an
assembly line. Work & Stress, 3(2):143–153.
Makowski, D., Pham, T., Lau, Z. J., Brammer, J. C.,
Lespinasse, F., Pham, H., Sch
¨
olzel, C., and Chen, S.
H. A. (2021). NeuroKit2: A python toolbox for neu-
rophysiological signal processing. Behavior Research
Methods, 53(4):1689–1696.
Mang, Z. A., Realzola, R. A., Ducharme, J., Bellissimo,
G. F., Beam, J. R., Mermier, C., de Castro Magalhaes,
F., Kravitz, L., and Amorim, F. T. (2022). The Effect
of Repetition Tempo on Cardiovascular and Metabolic
Stress When Time Under Tension is Matched During
Lower Body Exercise. European Journal of Applied
Physiology, 122(6):1485–1495.
Massaroni, C., Nicol
`
o, A., Lo Presti, D., Sacchetti, M.,
Silvestri, S., and Schena, E. (2019). Contact-Based
Methods for Measuring Respiratory Rate. Sensors,
19(4):908.
Mccraty, R. and Shaffer, F. (2015). Heart Rate Variabil-
ity: New Perspectives on Physiological Mechanisms,
Assessment of Self-regulatory Capacity, and Health
Risk. Global Advances in Health and Medicine,
4(1):46–61. PMID: 25694852.
Nardolillo, A. M., Baghdadi, A., and Cavuoto, L. A. (2017).
Heart Rate Variability During a Simulated Assembly
Task; Influence of Age and Gender. volume 61, pages
1853–1857.
Nicol
`
o, A., Marcora, S. M., Bazzucchi, I., and Sacchetti, M.
(2017a). Differential control of respiratory frequency
and tidal volume during high-intensity interval train-
ing. Experimental physiology, 102(8):934–949.
Nicol
`
o, A., Massaroni, C., and Passfield, L. (2017b). Res-
piratory Frequency During Exercise: The Neglected
Physiological Measure. Frontiers in Physiology,
8:922.
NIOSH (1981). Work Practices Guide for Manual Lifting.
Number 81-122 in DHHS (NIOSH) publication. U.S.
Department of Health and Human Services, Public
Health Service, Centers for Disease Control, National
Institute for Occupational Safety and Health, Division
of Biomedical and Behavioral Science.
Niu, S. (2010). Ergonomics and occupational safety and
health: An ILO perspective. Applied Ergonomics,
41(6):744–753.
Palmerud, G., Forsman, M., Neumann, W. P., and Winkel,
J. (2012). Mechanical exposure implications of ratio-
nalization: A comparison of two flow strategies in a
Swedish manufacturing plant. Applied Ergonomics,
43(6):1110–1121.
Pickering, T. G., Devereux, R. B., James, G. D., Gerin, W.,
Landsbergis, P., Schnall, P. L., and Schwartz, J. E.
(1996). Environmental influences on blood pressure
and the role of job strain. Journal of hypertension.
Supplement : official journal of the International So-
ciety of Hypertension, 14(5):S179–85.
R
´
etory, Y., Niedzialkowski, P., de Picciotto, C., Bonay, M.,
and Petitjean, M. (2016). New Respiratory Inductive
Plethysmography (RIP) Method for Evaluating Ven-
tilatory Adaptation during Mild Physical Activities.
PLoS ONE, 11.
Rodrigues, J., Liu, H., Folgado, D., Belo, D., Schultz,
T., and Gamboa, H. (2022). Feature-Based Informa-
BIODEVICES 2024 - 17th International Conference on Biomedical Electronics and Devices
80