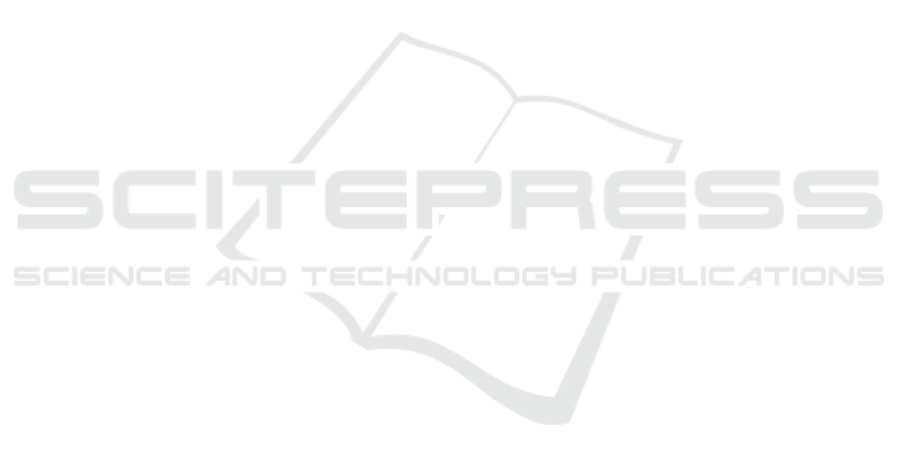
Choi, Y., Kim, Y., Kim, M., and Yoon, B. (2019). Muscle
synergies for turning during human walking. Journal
of Motor Behavior, Vol.51(No.1):1–9.
Coscia, M., Cheung, V. C., Tropea, P., Koenig, A., Monaco,
V., Bennis, C., Micera, S., Bonato, P., and Cheung,
V. C. K. (2014). The effect of arm weight support on
upper limb muscle synergies during reaching move-
ments. Journal of neuroengineering and rehabilita-
tion, Vol.11(No.1):22.
Curado, M. R., Cossio, E. G., Broetz, D., Agostini, M., Cho,
W., Brasil, F. L., Yilmaz, O., Liberati, G., Lepski, G.,
Birbaumer, N., and Ramos-Murguialday, A. (2015).
Residual upper arm motor function primes innerva-
tion of paretic forearm muscles in chronic stroke af-
ter brain-machine interface (bmi) training. PloS one,
Vol.10(No.10):e0140161.
d’Avella, Andrea.and Saltiel, P. and Bizzi, E. (2003). Com-
binations of muscle synergies in the construction
of a natural motor behavior. Nature neuroscience,
Vol.6(No.3):300–308.
Esmaeili, J. and Maleki, A. (2020). Muscle coordina-
tion analysis by time-varying muscle synergy extrac-
tion during cycling across various mechanical condi-
tions. Biocybernetics and Biomedical Engineering,
Vol.40(No.1):90–99.
Ettema, G. J. C., Taylor, Emma.and North, J. D., and Kip-
pers, V. (2005). Muscle synergies at the elbow in static
and oscillating isometric torque tasks with dual de-
grees of freedom. Motor Control, Vol.9(No.1):59–74.
Feldman, A. G. and Levin, M. F. (1995). The origin and use
of positional frames of reference in motor control. Be-
havioral and Brain Sciences, Vol.18(No.4):723–806.
Franklin, D. W., Osu, R., Burdet, E., and Kawato, Mit-
suo.and Milner, T. E. (2003). Adaptation to stable and
unstable dynamics achieved by combined impedance
control and inverse dynamics model. Journal of Neu-
rophysiology, Vol.90(No.5):3270–3282.
Franklin, D. W. and Theodore E., e. a. (2007). Endpoint
stiffness of the arm is directionally tuned to instabil-
ity in the environment. The Journal of Neuroscience,
Vol.27(No.29):7705–7716.
Hermens, H. J., Freriks, B., Dißelhorst-Klug, C., and
Rau, G. (2000). Development of recommenda-
tions for semg sensors and sensor placement proce-
dures. Journal of Electromyography and Kinesiology,
Vol.10(No.5):361–374.
K, Z., Z, Z., H, W., and A, S. (2021). Intra-subject
and inter-subject movement variability quantified with
muscle synergies in upper-limb reaching movements.
Biomimetics, Vol.6(No.4):63.
Kieliba, P., Tropea, P., Pirondini, E., Coscia, M., Micera, S.,
and Artoni, F. (2018). How are muscle synergies af-
fected by electromyography pre-processing? IEEE
Transactions on Neural Systems and Rehabilitation
Engineering, Vol.26(No.4):882–893.
Kojima, S., Takeda, M., Nambu, I., and Wada, Y. (2017).
Relations between required accuracy and muscle syn-
ergy in isometric contraction tasks. 2017 IEEE
INTERNATIONAL CONFERENCE ON SYSTEMS,
MAN, AND CYBERNETICS (SMC), pages 1191–
1195.
L. H. Ting, J. M. M. (2004). A limited set of muscle syner-
gies for force control during a postural task. Journal
of Neurophysiology, Vol.93(No.1):609–613.
Lee, D. D. and Seung, H. S. (1999). Learning the parts of
objects by non-negative matrix factorization. Nature,
Vol.401(No.6755):788.
McGraw, K. O. and Wong, S. P. (1996). Forming inferences
about some intraclass correlation coefficients. Psycho-
logical Methods, Vol.1(No.1):30–46.
McKay, J. L. and Ting, L. H. (2008). Functional muscle
synergies constrain force production during postural
tasks. Journal of biomechanics, Vol.41(No.2):299–
306.
Mira, R. M., Tosatti, L. M., Sacco, M., and Scano, A.
(2021). Detailed characterization of physiological
emg activations and directional tuning of upper-limb
and trunk muscles in point-to-point reaching move-
ments. Current Research in Physiology, Vol.4:60–72.
Miyazaki, H. T. K. H. (2011). Extraction and Implemen-
tation of Muscle Synergies in Hand-Force Control.
Department of Mechanical Science and Bioengineer-
ing,Graduate School of Engineering Science,Osaka
University,Japan.
Nicolas A, T., Romain, M., and Mickael, B. (2020). Shoul-
der muscle activation strategies differ when lifting or
lowering a load. European journal of applied physiol-
ogy, Vol.120(No.11):2417–2429.
Osu, Rieko.and Kamimura, N., , Nakano, E., and Harris,
Chris M.and Wada, Y. (2004). Optimal impedance
control for task achievement in the presence of
signal-dependent noise. Journal of Neurophysiology,
Vol.92(No.2):1199–1215.
Ouyang, C. Y., Cai, L. M., Liu, B., and Zhang, T. X. (2023).
An improved wavelet threshold denoising approach
for surface electromyography signal. Eurasip Journal
on Advances in Signal Processing, 2023(1).
Robert, T. and Latash, M. (2008). Time evolution of
the organization of multi-muscle postural responses
to sudden changes in the external force applied
at the trunk level(article). Neuroscience Letters,
Vol.438(No.2):238–241.
Roh, J., Rymer, W., and Beer, R. (2012). Robustness of
muscle synergies underlying three-dimensional force
generation at the hand in healthy humans(article).
Journal of Neurophysiology, Vol.107(No.8):2123–
2142.
Roh, J., Rymer, W., Perreault, E., Yoo, S., and Beer, R.
(2013). Alterations in upper limb muscle synergy
structure in chronic stroke survivors(article). Journal
of Neurophysiology, Vol.109(No.3):768–781.
Salman, B., Vahdat, S., Lambercy, O., Dovat, L., Burdet, E.,
and Milner, T. (2010). Changes in Muscle Activation
Patterns Following Robot-assisted Training of Hand
Function after Stroke. Sion Frser Universiy, Burnby,
BC CndMcGi Universiy, Monre, QC CndETH Zurich,
Zurich, SwizerndGoech, SwizerndIeri Coege of Sci-
ence, Technoogy nd Medicine, London UKMcGi Uni-
versiy.
Sangwan, S., Green, R. A., and Taylor, N. F. (2015).
Stabilizing characteristics of rotator cuff muscles: a
Associating Endpoint Accuracy and Similarity of Muscle Synergies
693