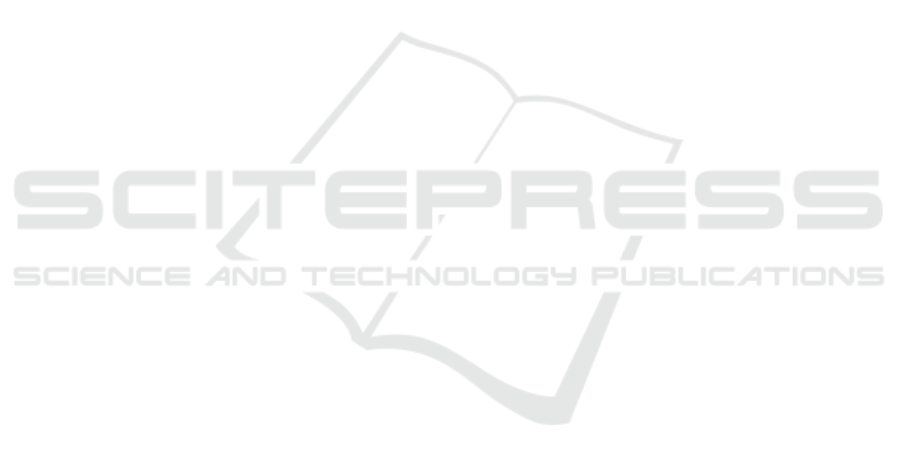
medicine. Evidence-Based Complementary and Al-
ternative Medicine, 2014:1–2.
Liu, H. and Schultz, T. (2022). How long are various types
of daily activities? statistical analysis of a multimodal
wearable sensor-based human activity dataset. In Pro-
ceedings of the 15th International Joint Conference
on Biomedical Engineering Systems and Technologies
(BIOSTEC 2022) - Volume 5: HEALTHINF, pages
680–688.
Liu, H., Xue, T., and Schultz, T. (2023). On a real real-time
wearable human activity recognition system. In Pro-
ceedings of the 16th International Joint Conference
on Biomedical Engineering Systems and Technologies
(BIOSTEC 2023) - WHC, pages 711–720.
Malik, M., Bigger, J. T., Camm, A. J., Kleiger, R. E.,
Malliani, A., Moss, A. J., and Schwartz, P. J. (1996).
Heart rate variability: Standards of measurement,
physiological interpretation, and clinical use. Euro-
pean Heart Journal, 17(3):354–381.
Martin, K., Meeusen, R., Thompson, K. G., Keegan, R.,
and Rattray, B. (2018). Mental fatigue impairs en-
durance performance: A physiological explanation.
Sports Medicine, 48(9):2041–2051.
Melliti, N., Zarrouk, F., and Souissi, N. (2016). Motiva-
tion expectations and motivational styles adopted by
the physical education teacher towards his students:
A study in a natural context of teaching and learning.
Creative Education, 07(15):2226–2250.
Moradi, J., Bahrami, A., and Dana, A. (2020). Motivation
for participation in sports based on athletes in team
and individual sports. Physical Culture and Sport.
Studies and Research, 85(1):14–21.
Morici, G., Gruttad’Auria, C. I., Baiamonte, P., Mazzuca,
E., Castrogiovanni, A., and Bonsignore, M. R. (2016).
Endurance training: Is it bad for&you?; Breathe,
12(2):140–147.
Pagani, M., Lombardi, F., Guzzetti, S., Rimoldi, O.,
Furlan, R., Pizzinelli, P., Sandrone, G., Malfatto, G.,
Dell’Orto, S., and Piccaluga, E. (1986). Power spec-
tral analysis of heart rate and arterial pressure vari-
abilities as a marker of sympatho-vagal interaction
in man and conscious dog. Circulation Research,
59(2):178–193.
Paulus, J., M
¨
uller, M., and Klapuri, A. (2010). State of
the art report: Audio-based music structure analysis.
pages 625–636.
Reaz, M. B., Hussain, M. S., and Mohd-Yasin, F. (2006).
Techniques of emg signal analysis: Detection, pro-
cessing, classification and applications. Biological
Procedures Online, 8(1):11–35.
Rodrigues, J., Liu, H., Folgado, D., Belo, D., Schultz,
T., and Gamboa, H. (2022). Feature-based informa-
tion retrieval of multimodal biosignals with a self-
similarity matrix: Focus on automatic segmentation.
Biosensors, 12(12):1182.
Ryan, R. M., Lynch, M. F., Vansteenkiste, M., and Deci,
E. L. (2010). Motivation and autonomy in counseling,
psychotherapy, and behavior change: A look at the-
ory and practice 1ψ7. The Counseling Psychologist,
39(2):193–260.
Santos, A., Rodrigues, J., Folgado, D., Santos, S., Fuj
˜
ao,
C., and Gamboa, H. (2021). Self-similarity matrix
of morphological features for motion data analysis in
manufacturing scenarios. Proceedings of the 14th In-
ternational Joint Conference on Biomedical Engineer-
ing Systems and Technologies.
Shaffer, F. and Ginsberg, J. P. (2017). An overview of heart
rate variability metrics and norms. Frontiers in Public
Health, 5.
Shi, W., Zhou, C., Zhang, Y., Li, K., Ren, X., Liu, H., and
Ye, X. (2023). Hybrid modeling on reconstitution of
continuous arterial blood pressure using finger photo-
plethysmography. Biomedical Signal Processing and
Control, 85:104972.
Taylor, I. M., Boat, R., and Murphy, S. L. (2018). Integrat-
ing theories of self-control and motivation to advance
endurance performance. International Review of Sport
and Exercise Psychology, 13(1):1–20.
Tiwari, R., Kumar, R., Malik, S., Raj, T., and Kumar, P.
(2021). Analysis of heart rate variability and implica-
tion of different factors on heart rate variability. Cur-
rent Cardiology Reviews, 17(5).
Veldanda, A., Liu, H., Koschke, R., Schultz, T., and K
¨
uster,
D. (2024). Can electromyography alone reveal facial
action units? a pilot emg-based action unit recogni-
tion study with real-time validation. In BIODEVICES
2024 — 17th International Conference on Biomedical
Electronics and Devices. INSTICC, SciTePress.
Wang, H., Li, K., Liu, H., Ye, X., and Zhou, C. (2024).
Comfort assessment method of eeg-based exoskele-
ton walkingassistive device. In BIOSIGNALS 2024
— 17th International Conference on Bio-Inspired Sys-
tems and Signal Processing. INSTICC, SciTePress.
Weiss, M. R. and Williams, L. (2004). The Why of Youth
Sport Involvement: A Developmental Perspective on
Motivational Processes., page 223–268. Fitness In-
formation Technology.
Wu, C., Huang, Y., and Hwang, J. (2015). Review of af-
fective computing in education/learning: Trends and
challenges. British Journal of Educational Technol-
ogy, 47(6):1304–1323.
Really Can’t Hold On Anymore? Physiological Indicators Versus Self-Reported Motivation Drop During Jogging
831